Attention! Your ePaper is waiting for publication!
By publishing your document, the content will be optimally indexed by Google via AI and sorted into the right category for over 500 million ePaper readers on YUMPU.
This will ensure high visibility and many readers!
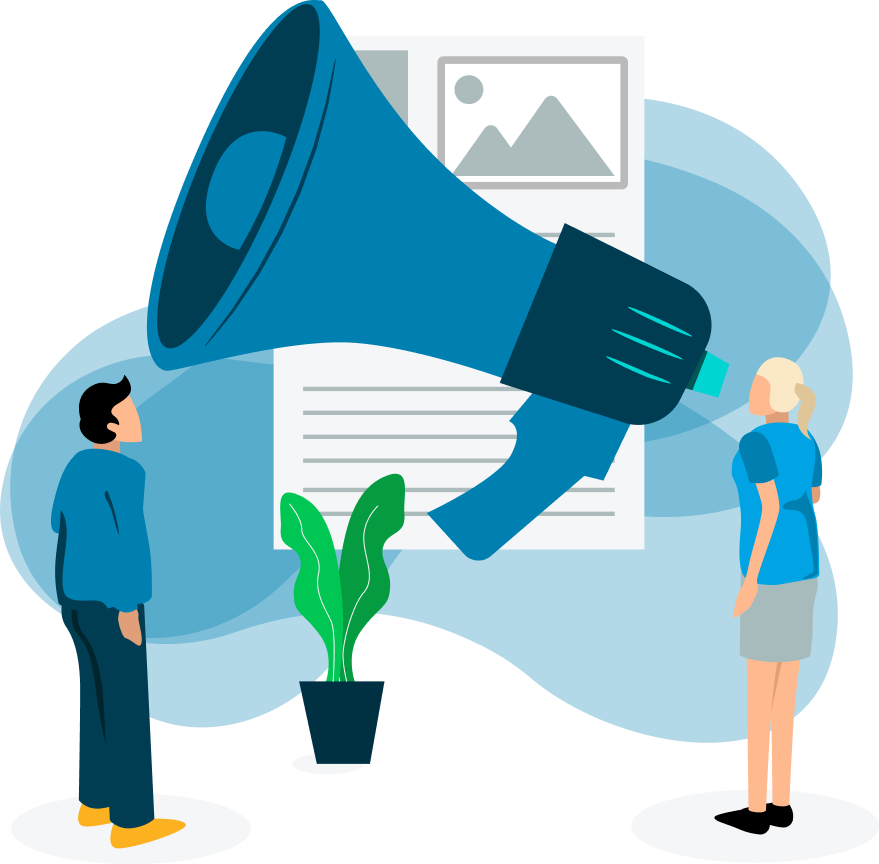
Your ePaper is now published and live on YUMPU!
You can find your publication here:
Share your interactive ePaper on all platforms and on your website with our embed function
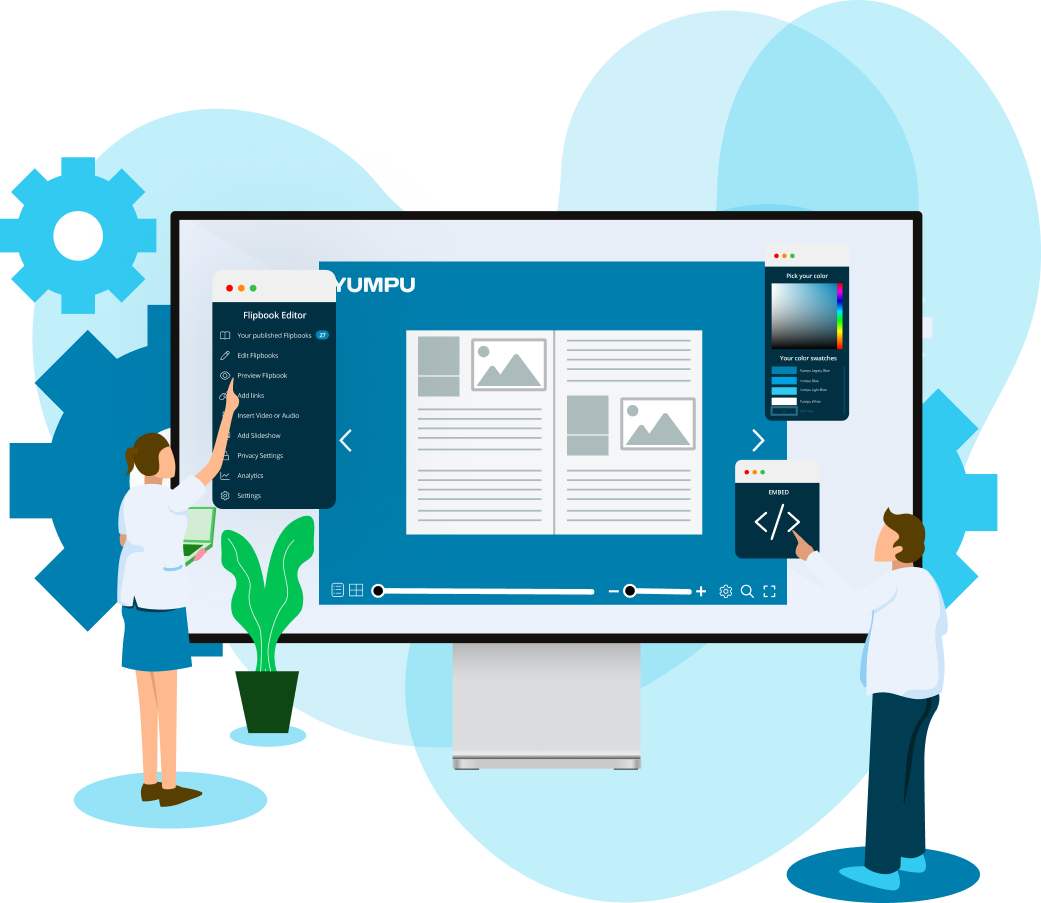
Ivancevic_Applied-Diff-Geom
Ivancevic_Applied-Diff-Geom
Ivancevic_Applied-Diff-Geom
Create successful ePaper yourself
Turn your PDF publications into a flip-book with our unique Google optimized e-Paper software.
<strong>Geom</strong>etrical Path Integrals and Their Applications 1089transition process, according to one of the two standard neural learningschemes, in which the micro–time level is traversed in discrete steps, i.e., ift = t 0 , t 1 , ..., t s then t + 1 = t 1 , t 2 , ..., t s+1 :(1) A self–organized, unsupervised (e.g., Hebbian–like [Hebb (1949)]) learningrule:w s (t + 1) = w s (t) + σ η (wd s(t) − w a s (t)), (6.135)where σ = σ(t), η = η(t) denote signal and noise, respectively, whilesuperscripts d and a denote desired and achieved micro–states, respectively;or(2) A certain form of a supervised gradient descent learning:w s (t + 1) = w s (t) − η∇J(t), (6.136)where η is a small constant, called the step size, or the learning rateand ∇J(n) denotes the gradient of the ‘performance hyper–surface’ atthe t−th iteration.Both Hebbian and supervised learning are used for the local decision makingprocess (see below) occurring at the intention formation faze F.In this way, local micro–level of LSF total represents an infinite–dimensional neural network. In the cognitive psychology framework, ouradaptive path integral (6.133) can be interpreted as semantic integration(see [Bransford and Franks (1971); Ashcraft (1994)]).Motion and Decision Making in LSF pathsOn the macro–level in the subspace LSF paths we have the (loco)motionaction principleδS[x] = 0,with the Newtonian–like action S[x] given byS[x] =∫ tfint inidt [ 1 2 g ij ẋ i ẋ j + ϕ i (x i )], (6.137)where overdot denotes time derivative, so that ẋ i represents processingspeed, or (loco)motion velocity vector. The first bracket term in (6.137)represents the kinetic energy T ,T = 1 2 g ij ẋ i ẋ j ,
<strong>Geom</strong>etrical Path Integrals and Their Applications 1089transition process, according to one of the two standard neural learningschemes, in which the micro–time level is traversed in discrete steps, i.e., ift = t 0 , t 1 , ..., t s then t + 1 = t 1 , t 2 , ..., t s+1 :(1) A self–organized, unsupervised (e.g., Hebbian–like [Hebb (1949)]) learningrule:w s (t + 1) = w s (t) + σ η (wd s(t) − w a s (t)), (6.135)where σ = σ(t), η = η(t) denote signal and noise, respectively, whilesuperscripts d and a denote desired and achieved micro–states, respectively;or(2) A certain form of a supervised gradient descent learning:w s (t + 1) = w s (t) − η∇J(t), (6.136)where η is a small constant, called the step size, or the learning rateand ∇J(n) denotes the gradient of the ‘performance hyper–surface’ atthe t−th iteration.Both Hebbian and supervised learning are used for the local decision makingprocess (see below) occurring at the intention formation faze F.In this way, local micro–level of LSF total represents an infinite–dimensional neural network. In the cognitive psychology framework, ouradaptive path integral (6.133) can be interpreted as semantic integration(see [Bransford and Franks (1971); Ashcraft (1994)]).Motion and Decision Making in LSF pathsOn the macro–level in the subspace LSF paths we have the (loco)motionaction principleδS[x] = 0,with the Newtonian–like action S[x] given byS[x] =∫ tfint inidt [ 1 2 g ij ẋ i ẋ j + ϕ i (x i )], (6.137)where overdot denotes time derivative, so that ẋ i represents processingspeed, or (loco)motion velocity vector. The first bracket term in (6.137)represents the kinetic energy T ,T = 1 2 g ij ẋ i ẋ j ,
1090 <strong>Applied</strong> <strong>Diff</strong>erential <strong>Geom</strong>etry: A Modern Introductiongenerated by the Riemannian metric tensor g ij , while the second bracketterm, ϕ i (x i ), denotes the family of potential force–fields, driving the(loco)mo-tions x i = x i (t) (the strengths of the fields ϕ i (x i ) depend ontheir positions x i in LSF, see LSF fields below). The corresponding Euler–Lagrangian equation gives the Newtonian–like equation of motionddt T ẋ i − T x i = −ϕi xi, (6.138)(subscripts denote the partial derivatives), which can be put into the standardLagrangian formddt L ẋ i = L x i, with L = T − ϕi (x i ).In the next subsection we use the micro–level implications of the actionS[x] as given by (6.137), for dynamical descriptions of the local decision–making process.On the micro–level in the subspace LSF paths , instead of a single pathdefined by the Newtonian–like equation of motion (6.138), we have an ensembleof fluctuating and crossing paths with weighted probabilities (of theunit total sum). This ensemble of micro–paths is defined by the simplestinstance of our adaptive path integral (6.133), similar to the Feynman’soriginal sum over histories,∫〈Action|Intention〉 paths = Σ D[wx] e iS[x] , (6.139)where D[wx] is a functional measure on the space of all weighted paths,and the exponential depends on the action S[x] given by (6.137). Thisprocedure can be redefined in a mathematically cleaner way if we Wick–rotate the time variable t to imaginary values t ↦→ τ = it, thereby makingall integrals real:∫ ∫Σ D[wx] e iS[x] W ick✲ Σ D[wx] e −S[x] . (6.140)Discretization of (6.140) gives the thermodynamic–like partition functionZ = ∑ je −wjEj /T , (6.141)where E j is the motion energy eigenvalue (reflecting each possible motivationalenergetic state), T is the temperature–like environmental controlparameter, and the sum runs over all motion energy eigenstates (labelled
- Page 2 and 3:
APPLIEDDIFFERENTIALGEOMETRYA Modern
- Page 4 and 5:
APPLIEDDIFFERENTIALGEOMETRYA Modern
- Page 6 and 7:
Dedicated to:Nitya, Atma and Kali
- Page 8 and 9:
PrefaceApplied Differential Geometr
- Page 10 and 11:
ixAcknowledgmentsThe authors wish t
- Page 12 and 13:
Glossary of Frequently Used Symbols
- Page 14 and 15:
Glossary of Frequently Used Symbols
- Page 16 and 17:
Glossary of Frequently Used Symbols
- Page 18 and 19:
ContentsPrefaceGlossary of Frequent
- Page 20 and 21:
Contentsxix2.1.5.2 Forces Acting on
- Page 22 and 23:
Contentsxxi3.6.3.4 Stokes Theorem a
- Page 24 and 25:
Contentsxxiii3.10.3.1 Basis of Lagr
- Page 26 and 27:
Contentsxxv3.17 Applied Unorthodox
- Page 28 and 29:
Contentsxxvii4.9.8.2 Geometrodynami
- Page 30 and 31:
Contentsxxix4.14.7.5 Monopole Conde
- Page 32 and 33:
Contentsxxxi5.12.3 Hawking-Penrose
- Page 34 and 35:
Contentsxxxiii6.5.2.4 Dimensional R
- Page 36 and 37:
Chapter 1IntroductionIn this introd
- Page 38 and 39:
Introduction 3Fig. 1.1 The four cha
- Page 40 and 41:
Introduction 5• Riemannian manifo
- Page 42 and 43:
Introduction 7charts.The atlas cont
- Page 44 and 45:
Introduction 9every point has a nei
- Page 46 and 47:
Introduction 11of curves include ci
- Page 48 and 49:
Introduction 13the variables define
- Page 50 and 51:
Introduction 15U i denotes one of t
- Page 52 and 53:
Introduction 17which varies smoothl
- Page 54 and 55:
Introduction 19interactions.1.1.5.2
- Page 56 and 57:
Introduction 21real number. This co
- Page 58 and 59:
Introduction 23Such a force is inde
- Page 60 and 61:
Introduction 25tons. In this formul
- Page 62 and 63:
Introduction 27vector-field are sol
- Page 64 and 65:
Introduction 29or simply connected)
- Page 66 and 67:
Introduction 311.1.8 Application: A
- Page 68 and 69:
Introduction 33theory itself existe
- Page 70 and 71:
Introduction 35mediate the forces.
- Page 72 and 73:
Introduction 37theory. 50 Fig. 1.3
- Page 74 and 75:
Introduction 39to describe a univer
- Page 76 and 77:
Introduction 41which have two disti
- Page 78 and 79:
Introduction 43first theory can be
- Page 80 and 81:
Introduction 45the Casimir effect,
- Page 82 and 83:
Introduction 47• External coordin
- Page 84 and 85:
Introduction 49a connection-base de
- Page 86 and 87:
Chapter 2Technical Preliminaries: T
- Page 88 and 89:
Technical Preliminaries: Tensors, A
- Page 90 and 91:
Technical Preliminaries: Tensors, A
- Page 92 and 93:
Technical Preliminaries: Tensors, A
- Page 94 and 95:
Technical Preliminaries: Tensors, A
- Page 96 and 97:
Technical Preliminaries: Tensors, A
- Page 98 and 99:
Technical Preliminaries: Tensors, A
- Page 100 and 101:
Technical Preliminaries: Tensors, A
- Page 102 and 103:
Technical Preliminaries: Tensors, A
- Page 104 and 105:
Technical Preliminaries: Tensors, A
- Page 106 and 107:
Technical Preliminaries: Tensors, A
- Page 108 and 109:
Technical Preliminaries: Tensors, A
- Page 110 and 111:
Technical Preliminaries: Tensors, A
- Page 112 and 113:
Technical Preliminaries: Tensors, A
- Page 114 and 115:
Technical Preliminaries: Tensors, A
- Page 116 and 117:
Technical Preliminaries: Tensors, A
- Page 118 and 119:
Technical Preliminaries: Tensors, A
- Page 120 and 121:
Technical Preliminaries: Tensors, A
- Page 122 and 123:
Technical Preliminaries: Tensors, A
- Page 124 and 125:
Technical Preliminaries: Tensors, A
- Page 126 and 127:
Technical Preliminaries: Tensors, A
- Page 128 and 129:
Technical Preliminaries: Tensors, A
- Page 130 and 131:
Technical Preliminaries: Tensors, A
- Page 132 and 133:
Technical Preliminaries: Tensors, A
- Page 134 and 135:
Technical Preliminaries: Tensors, A
- Page 136 and 137:
Technical Preliminaries: Tensors, A
- Page 138 and 139:
Technical Preliminaries: Tensors, A
- Page 140 and 141:
Technical Preliminaries: Tensors, A
- Page 142 and 143:
Technical Preliminaries: Tensors, A
- Page 144 and 145:
Technical Preliminaries: Tensors, A
- Page 146 and 147:
Technical Preliminaries: Tensors, A
- Page 148 and 149:
Technical Preliminaries: Tensors, A
- Page 150 and 151:
Technical Preliminaries: Tensors, A
- Page 152 and 153:
Technical Preliminaries: Tensors, A
- Page 154 and 155:
Technical Preliminaries: Tensors, A
- Page 156 and 157:
Technical Preliminaries: Tensors, A
- Page 158 and 159:
Technical Preliminaries: Tensors, A
- Page 160 and 161:
Technical Preliminaries: Tensors, A
- Page 162 and 163:
Technical Preliminaries: Tensors, A
- Page 164 and 165:
Technical Preliminaries: Tensors, A
- Page 166 and 167:
Technical Preliminaries: Tensors, A
- Page 168 and 169:
Technical Preliminaries: Tensors, A
- Page 170 and 171:
Technical Preliminaries: Tensors, A
- Page 172 and 173:
Chapter 3Applied Manifold Geometry3
- Page 174 and 175:
Applied Manifold Geometry 139where
- Page 176 and 177:
Applied Manifold Geometry 141In loc
- Page 178 and 179:
Applied Manifold Geometry 143on the
- Page 180 and 181:
Applied Manifold Geometry 145exist
- Page 182 and 183:
Applied Manifold Geometry 147then E
- Page 184 and 185:
Applied Manifold Geometry 1490D man
- Page 186 and 187:
Applied Manifold Geometry 151Fig. 3
- Page 188 and 189:
Applied Manifold Geometry 153the fo
- Page 190 and 191:
Applied Manifold Geometry 155contin
- Page 192 and 193:
Applied Manifold Geometry 157In oth
- Page 194 and 195:
Applied Manifold Geometry 159scalar
- Page 196 and 197:
Applied Manifold Geometry 161where
- Page 198 and 199:
Applied Manifold Geometry 1633.6 Te
- Page 200 and 201:
Applied Manifold Geometry 165Let ϕ
- Page 202 and 203:
Applied Manifold Geometry 1673.6.1.
- Page 204 and 205:
Applied Manifold Geometry 169or, if
- Page 206 and 207:
Applied Manifold Geometry 171If X i
- Page 208 and 209:
Applied Manifold Geometry 173along
- Page 210 and 211:
Applied Manifold Geometry 175is dua
- Page 212 and 213:
Applied Manifold Geometry 177where
- Page 214 and 215:
Applied Manifold Geometry 179(1) α
- Page 216 and 217:
Applied Manifold Geometry 181diagra
- Page 218 and 219:
Applied Manifold Geometry 183Clearl
- Page 220 and 221:
Applied Manifold Geometry 1853.6.3.
- Page 222 and 223:
Applied Manifold Geometry 187duces,
- Page 224 and 225:
Applied Manifold Geometry 189This i
- Page 226 and 227:
Applied Manifold Geometry 191Hodge
- Page 228 and 229:
Applied Manifold Geometry 193The in
- Page 230 and 231:
Applied Manifold Geometry 195since
- Page 232 and 233:
Applied Manifold Geometry 197If a l
- Page 234 and 235:
Applied Manifold Geometry 199asGive
- Page 236 and 237:
Applied Manifold Geometry 201The co
- Page 238 and 239:
Applied Manifold Geometry 203at the
- Page 240 and 241:
Applied Manifold Geometry 205For ex
- Page 242 and 243:
Applied Manifold Geometry 207axis,
- Page 244 and 245:
Applied Manifold Geometry 209For ex
- Page 246 and 247:
Applied Manifold Geometry 211The sp
- Page 248 and 249:
Applied Manifold Geometry 213corres
- Page 250 and 251:
Applied Manifold Geometry 215corres
- Page 252 and 253:
Applied Manifold Geometry 217Specia
- Page 254 and 255:
Applied Manifold Geometry 219the co
- Page 256 and 257:
Applied Manifold Geometry 221moment
- Page 258 and 259:
Applied Manifold Geometry 223produc
- Page 260 and 261:
Applied Manifold Geometry 225has a
- Page 262 and 263:
Applied Manifold Geometry 2273.8.5
- Page 264 and 265:
Applied Manifold Geometry 229in whi
- Page 266 and 267:
Applied Manifold Geometry 231with
- Page 268 and 269:
Applied Manifold Geometry 233itly:(
- Page 270 and 271:
Applied Manifold Geometry 235play t
- Page 272 and 273:
Applied Manifold Geometry 237Some r
- Page 274 and 275:
Applied Manifold Geometry 239Basic
- Page 276 and 277:
Applied Manifold Geometry 241orthog
- Page 278 and 279:
Applied Manifold Geometry 243is adm
- Page 280 and 281:
Applied Manifold Geometry 245Root S
- Page 282 and 283:
Applied Manifold Geometry 247groups
- Page 284 and 285:
Applied Manifold Geometry 2493.9.1.
- Page 286 and 287:
Applied Manifold Geometry 251second
- Page 288 and 289:
Applied Manifold Geometry 253where
- Page 290 and 291:
Applied Manifold Geometry 255be a v
- Page 292 and 293:
Applied Manifold Geometry 257Now, a
- Page 294 and 295:
Applied Manifold Geometry 259This i
- Page 296 and 297:
Applied Manifold Geometry 2613.9.4
- Page 298 and 299:
Applied Manifold Geometry 263other
- Page 300 and 301:
Applied Manifold Geometry 265where
- Page 302 and 303:
Applied Manifold Geometry 267result
- Page 304 and 305:
Applied Manifold Geometry 269where
- Page 306 and 307:
Applied Manifold Geometry 2713.10 R
- Page 308 and 309:
Applied Manifold Geometry 273More p
- Page 310 and 311:
Applied Manifold Geometry 275Y (t 0
- Page 312 and 313:
Applied Manifold Geometry 277which
- Page 314 and 315:
Applied Manifold Geometry 279for an
- Page 316 and 317:
Applied Manifold Geometry 281where
- Page 318 and 319:
Applied Manifold Geometry 283Thus w
- Page 320 and 321:
Applied Manifold Geometry 2853.10.2
- Page 322 and 323:
Applied Manifold Geometry 287evolut
- Page 324 and 325:
Applied Manifold Geometry 2893.10.3
- Page 326 and 327:
Applied Manifold Geometry 2913.10.3
- Page 328 and 329:
Applied Manifold Geometry 293‘ren
- Page 330 and 331:
Applied Manifold Geometry 295mute;
- Page 332 and 333:
Applied Manifold Geometry 297Rather
- Page 334 and 335:
Applied Manifold Geometry 299of the
- Page 336 and 337:
Applied Manifold Geometry 301clidea
- Page 338 and 339:
Applied Manifold Geometry 303Second
- Page 340 and 341:
Applied Manifold Geometry 305i.e.,
- Page 342 and 343:
Applied Manifold Geometry 307invari
- Page 344 and 345:
Applied Manifold Geometry 309The Ba
- Page 346 and 347:
Applied Manifold Geometry 311Let us
- Page 348 and 349:
Applied Manifold Geometry 313all cu
- Page 350 and 351:
Applied Manifold Geometry 315ders.
- Page 352 and 353:
Applied Manifold Geometry 317this c
- Page 354 and 355:
Applied Manifold Geometry 319In par
- Page 356 and 357:
Applied Manifold Geometry 321As I p
- Page 358 and 359:
Applied Manifold Geometry 323the fo
- Page 360 and 361:
Applied Manifold Geometry 325per (1
- Page 362 and 363:
Applied Manifold Geometry 327Genera
- Page 364 and 365:
Applied Manifold Geometry 329The ma
- Page 366 and 367:
Applied Manifold Geometry 331pretat
- Page 368 and 369:
Applied Manifold Geometry 333byMDL
- Page 370 and 371:
Applied Manifold Geometry 3353.12 S
- Page 372 and 373:
Applied Manifold Geometry 337H 2 (M
- Page 374 and 375:
Applied Manifold Geometry 339bracke
- Page 376 and 377:
Applied Manifold Geometry 341Any so
- Page 378 and 379:
Applied Manifold Geometry 343All on
- Page 380 and 381:
Applied Manifold Geometry 345The ac
- Page 382 and 383:
Applied Manifold Geometry 347linear
- Page 384 and 385:
Applied Manifold Geometry 349such t
- Page 386 and 387:
Applied Manifold Geometry 351Let γ
- Page 388 and 389:
Applied Manifold Geometry 353This i
- Page 390 and 391:
Applied Manifold Geometry 355chart
- Page 392 and 393:
Applied Manifold Geometry 357phase-
- Page 394 and 395:
Applied Manifold Geometry 359( ) 0
- Page 396 and 397:
Applied Manifold Geometry 361hetero
- Page 398 and 399:
Applied Manifold Geometry 363thus c
- Page 400 and 401:
Applied Manifold Geometry 365Action
- Page 402 and 403:
Applied Manifold Geometry 367and we
- Page 404 and 405:
Applied Manifold Geometry 369action
- Page 406 and 407:
Applied Manifold Geometry 371and we
- Page 408 and 409:
Applied Manifold Geometry 373by (J(
- Page 410 and 411:
Applied Manifold Geometry 375fields
- Page 412 and 413:
Applied Manifold Geometry 377Let Tx
- Page 414 and 415:
Applied Manifold Geometry 379where
- Page 416 and 417:
Applied Manifold Geometry 381The ve
- Page 418 and 419:
Applied Manifold Geometry 383and gi
- Page 420 and 421:
Applied Manifold Geometry 385The co
- Page 422 and 423:
Applied Manifold Geometry 387tor Ca
- Page 424 and 425:
Applied Manifold Geometry 389where
- Page 426 and 427:
Applied Manifold Geometry 3912 −
- Page 428 and 429:
Applied Manifold Geometry 393comple
- Page 430 and 431:
Applied Manifold Geometry 395F[C]
- Page 432 and 433:
Applied Manifold Geometry 397become
- Page 434 and 435:
Applied Manifold Geometry 399of fuz
- Page 436 and 437:
Applied Manifold Geometry 401In our
- Page 438 and 439:
Applied Manifold Geometry 403Biodyn
- Page 440 and 441:
Applied Manifold Geometry 405for T
- Page 442 and 443:
Applied Manifold Geometry 407A vari
- Page 444 and 445:
Applied Manifold Geometry 409such t
- Page 446 and 447:
Applied Manifold Geometry 411of neg
- Page 448 and 449:
Applied Manifold Geometry 413where
- Page 450 and 451:
Applied Manifold Geometry 415Follow
- Page 452 and 453:
Applied Manifold Geometry 417and J
- Page 454 and 455:
Applied Manifold Geometry 419This t
- Page 456 and 457:
Applied Manifold Geometry 421above,
- Page 458 and 459:
Applied Manifold Geometry 423As a L
- Page 460 and 461:
Applied Manifold Geometry 425Theore
- Page 462 and 463:
Applied Manifold Geometry 427real v
- Page 464 and 465:
Applied Manifold Geometry 429indepe
- Page 466 and 467:
Applied Manifold Geometry 431In ter
- Page 468 and 469:
Applied Manifold Geometry 433M, we
- Page 470 and 471:
Applied Manifold Geometry 435The cu
- Page 472 and 473:
Applied Manifold Geometry 437(iv) (
- Page 474 and 475:
Applied Manifold Geometry 439where
- Page 476 and 477:
. ..Applied Manifold Geometry 441in
- Page 478 and 479:
Applied Manifold Geometry 443⌋ is
- Page 480 and 481:
Applied Manifold Geometry 445Now, a
- Page 482 and 483:
Applied Manifold Geometry 447symmet
- Page 484 and 485:
Applied Manifold Geometry 449study
- Page 486 and 487:
Applied Manifold Geometry 451togeth
- Page 488 and 489:
Applied Manifold Geometry 453result
- Page 490 and 491:
Applied Manifold Geometry 455will b
- Page 492 and 493:
Applied Manifold Geometry 457g 11 =
- Page 494 and 495:
Applied Manifold Geometry 459is def
- Page 496 and 497:
Applied Manifold Geometry 461ordere
- Page 498 and 499:
Applied Manifold Geometry 463Now, r
- Page 500 and 501:
Applied Manifold Geometry 465Simila
- Page 502 and 503:
Applied Manifold Geometry 467which
- Page 504 and 505:
Applied Manifold Geometry 469From t
- Page 506 and 507:
Applied Manifold Geometry 471where
- Page 508 and 509:
Applied Manifold Geometry 4733.17.2
- Page 510 and 511:
Applied Manifold Geometry 475On the
- Page 512 and 513:
Applied Manifold Geometry 477ing di
- Page 514 and 515:
Applied Manifold Geometry 479Note t
- Page 516 and 517:
Applied Manifold Geometry 481Then w
- Page 518 and 519:
Applied Manifold Geometry 483consis
- Page 520 and 521:
Chapter 4Applied Bundle Geometry4.1
- Page 522 and 523:
Applied Bundle Geometry 487is calle
- Page 524 and 525:
Applied Bundle Geometry 489V and an
- Page 526 and 527:
Applied Bundle Geometry 4914.3 Vect
- Page 528 and 529:
Applied Bundle Geometry 493dimensio
- Page 530 and 531:
Applied Bundle Geometry 495orthogon
- Page 532 and 533:
Applied Bundle Geometry 497t, is a
- Page 534 and 535:
Applied Bundle Geometry 499Let T Y
- Page 536 and 537:
Applied Bundle Geometry 501In other
- Page 538 and 539:
Applied Bundle Geometry 503we shall
- Page 540 and 541:
Applied Bundle Geometry 505Pendulum
- Page 542 and 543:
Applied Bundle Geometry 507Using L
- Page 544 and 545:
Applied Bundle Geometry 509the isom
- Page 546 and 547:
Applied Bundle Geometry 511theory f
- Page 548 and 549:
Applied Bundle Geometry 513of the c
- Page 550 and 551:
Applied Bundle Geometry 515where Ω
- Page 552 and 553:
Applied Bundle Geometry 517nals, th
- Page 554 and 555:
Applied Bundle Geometry 519(1985)])
- Page 556 and 557:
Applied Bundle Geometry 521finite-d
- Page 558 and 559:
Applied Bundle Geometry 523and thes
- Page 560 and 561:
Applied Bundle Geometry 525Now, let
- Page 562 and 563:
Applied Bundle Geometry 527gravity
- Page 564 and 565:
Applied Bundle Geometry 529a Dp−b
- Page 566 and 567:
Applied Bundle Geometry 531A princi
- Page 568 and 569:
Applied Bundle Geometry 533is the r
- Page 570 and 571:
Applied Bundle Geometry 535Now, let
- Page 572 and 573:
Applied Bundle Geometry 537By cycli
- Page 574 and 575:
Applied Bundle Geometry 5394.9.2 Fe
- Page 576 and 577:
Applied Bundle Geometry 541This lin
- Page 578 and 579:
Applied Bundle Geometry 543(1) L g
- Page 580 and 581:
Applied Bundle Geometry 545and ω 0
- Page 582 and 583:
Applied Bundle Geometry 547isfiesω
- Page 584 and 585:
Applied Bundle Geometry 549of the c
- Page 586 and 587:
Applied Bundle Geometry 551Controll
- Page 588 and 589:
Applied Bundle Geometry 553Foliatio
- Page 590 and 591:
Applied Bundle Geometry 555ψ : (x,
- Page 592 and 593:
Applied Bundle Geometry 557from res
- Page 594 and 595:
Applied Bundle Geometry 559Motion a
- Page 596 and 597:
Applied Bundle Geometry 561where Z
- Page 598 and 599:
Applied Bundle Geometry 563Systems
- Page 600 and 601:
Applied Bundle Geometry 5653. Recal
- Page 602 and 603:
Applied Bundle Geometry 567Therefor
- Page 604 and 605:
Applied Bundle Geometry 569smooth m
- Page 606 and 607:
Applied Bundle Geometry 571state va
- Page 608 and 609:
Applied Bundle Geometry 573The geom
- Page 610 and 611:
Applied Bundle Geometry 575Y ∋ y
- Page 612 and 613:
Applied Bundle Geometry 577autonomo
- Page 614 and 615:
Applied Bundle Geometry 579(see 3.1
- Page 616 and 617:
Applied Bundle Geometry 581and init
- Page 618 and 619:
Applied Bundle Geometry 583potentia
- Page 620 and 621:
Applied Bundle Geometry 585effector
- Page 622 and 623:
Applied Bundle Geometry 587Fig. 4.9
- Page 624 and 625:
Applied Bundle Geometry 589physical
- Page 626 and 627:
Applied Bundle Geometry 591open Lio
- Page 628 and 629:
Applied Bundle Geometry 593et al. (
- Page 630 and 631:
Applied Bundle Geometry 595(2) Acti
- Page 632 and 633:
Applied Bundle Geometry 597˙Φ ≤
- Page 634 and 635:
Applied Bundle Geometry 599number o
- Page 636 and 637:
Applied Bundle Geometry 601where Ψ
- Page 638 and 639:
Applied Bundle Geometry 603ing to c
- Page 640 and 641:
Applied Bundle Geometry 605where [
- Page 642 and 643:
Applied Bundle Geometry 607interval
- Page 644 and 645:
Applied Bundle Geometry 609The foll
- Page 646 and 647:
Applied Bundle Geometry 611In parti
- Page 648 and 649:
Applied Bundle Geometry 613The pull
- Page 650 and 651:
Applied Bundle Geometry 615(2) δ
- Page 652 and 653:
Applied Bundle Geometry 617of unita
- Page 654 and 655:
Applied Bundle Geometry 619system o
- Page 656 and 657:
Applied Bundle Geometry 621of H pre
- Page 658 and 659:
Applied Bundle Geometry 623It follo
- Page 660 and 661:
Applied Bundle Geometry 625a produc
- Page 662 and 663:
Applied Bundle Geometry 627B Ham(M)
- Page 664 and 665:
Applied Bundle Geometry 629b.Let us
- Page 666 and 667:
Applied Bundle Geometry 631If P is
- Page 668 and 669:
Applied Bundle Geometry 633question
- Page 670 and 671:
Applied Bundle Geometry 635spheres
- Page 672 and 673:
Applied Bundle Geometry 637be thoug
- Page 674 and 675:
Applied Bundle Geometry 639of the b
- Page 676 and 677:
Applied Bundle Geometry 641in C 1 (
- Page 678 and 679:
Applied Bundle Geometry 643from a t
- Page 680 and 681:
Applied Bundle Geometry 645Now let
- Page 682 and 683:
Applied Bundle Geometry 647for t <
- Page 684 and 685:
Applied Bundle Geometry 649is a Ham
- Page 686 and 687:
Applied Bundle Geometry 651The foll
- Page 688 and 689:
Applied Bundle Geometry 653is the u
- Page 690 and 691:
Applied Bundle Geometry 655every no
- Page 692 and 693:
Applied Bundle Geometry 657P × P w
- Page 694 and 695:
Applied Bundle Geometry 659that the
- Page 696 and 697:
Applied Bundle Geometry 661extend t
- Page 698 and 699:
Applied Bundle Geometry 663identity
- Page 700 and 701:
Applied Bundle Geometry 665The mapw
- Page 702 and 703:
Applied Bundle Geometry 667It is a
- Page 704 and 705:
Applied Bundle Geometry 669I Q is i
- Page 706 and 707:
Applied Bundle Geometry 671whereC i
- Page 708 and 709:
Applied Bundle Geometry 673form Q.
- Page 710 and 711:
Applied Bundle Geometry 675τ( exp(
- Page 712 and 713:
Applied Bundle Geometry 6774.13.2.2
- Page 714 and 715:
Applied Bundle Geometry 679Therefor
- Page 716 and 717:
Applied Bundle Geometry 6814.13.2.3
- Page 718 and 719:
Applied Bundle Geometry 683⎛⎞co
- Page 720 and 721:
Applied Bundle Geometry 685Usually,
- Page 722 and 723:
Applied Bundle Geometry 687on the s
- Page 724 and 725:
Applied Bundle Geometry 6894.13.2.5
- Page 726 and 727:
Applied Bundle Geometry 6914.13.3 P
- Page 728 and 729:
Applied Bundle Geometry 693Since T
- Page 730 and 731:
Applied Bundle Geometry 695On the o
- Page 732 and 733:
Applied Bundle Geometry 697may writ
- Page 734 and 735:
Applied Bundle Geometry 699determin
- Page 736 and 737:
Applied Bundle Geometry 701section,
- Page 738 and 739:
Applied Bundle Geometry 703for deri
- Page 740 and 741:
Applied Bundle Geometry 705dictions
- Page 742 and 743:
Applied Bundle Geometry 707too sing
- Page 744 and 745:
Applied Bundle Geometry 709In (4.18
- Page 746 and 747:
Applied Bundle Geometry 7114.13.4.4
- Page 748 and 749:
Applied Bundle Geometry 713sis repr
- Page 750 and 751:
Applied Bundle Geometry 7154.13.4.6
- Page 752 and 753:
Applied Bundle Geometry 717a (linea
- Page 754 and 755:
Applied Bundle Geometry 719past few
- Page 756 and 757:
Applied Bundle Geometry 721global q
- Page 758 and 759:
Applied Bundle Geometry 7239. It ha
- Page 760 and 761:
Applied Bundle Geometry 725needs to
- Page 762 and 763:
Applied Bundle Geometry 727pear at
- Page 764 and 765:
Applied Bundle Geometry 729X, F A i
- Page 766 and 767:
Applied Bundle Geometry 731ifolds,
- Page 768 and 769:
Applied Bundle Geometry 733and not
- Page 770 and 771:
Applied Bundle Geometry 735under U(
- Page 772 and 773:
Applied Bundle Geometry 737N = 2 br
- Page 774 and 775:
Applied Bundle Geometry 739thusa
- Page 776 and 777:
Applied Bundle Geometry 741antisymm
- Page 778 and 779:
Applied Bundle Geometry 743see this
- Page 780 and 781:
Applied Bundle Geometry 745Coupling
- Page 782 and 783:
Applied Bundle Geometry 747acts as
- Page 784 and 785:
Applied Bundle Geometry 749good coo
- Page 786 and 787:
Applied Bundle Geometry 751Φ. Brea
- Page 788 and 789:
Applied Bundle Geometry 753equating
- Page 790 and 791:
Applied Bundle Geometry 755differen
- Page 792 and 793:
Applied Bundle Geometry 7574.14.10
- Page 794 and 795:
Applied Bundle Geometry 759deformed
- Page 796 and 797:
Applied Bundle Geometry 761phenomen
- Page 798 and 799:
Applied Bundle Geometry 763appears
- Page 800 and 801:
Applied Bundle Geometry 7654.14.10.
- Page 802 and 803:
Applied Bundle Geometry 767For J >
- Page 804 and 805:
Applied Bundle Geometry 769K 1/2
- Page 806 and 807:
Applied Bundle Geometry 771(i) The
- Page 808 and 809:
Applied Bundle Geometry 773by α =
- Page 810 and 811:
Applied Bundle Geometry 775(4.279)
- Page 812 and 813:
Applied Bundle Geometry 777Λ ⊗
- Page 814 and 815:
Applied Bundle Geometry 779coupling
- Page 816 and 817:
Applied Bundle Geometry 781where we
- Page 818 and 819:
Applied Bundle Geometry 783The posi
- Page 820 and 821:
Applied Bundle Geometry 785Although
- Page 822 and 823:
Applied Bundle Geometry 787Let us a
- Page 824 and 825:
Applied Bundle Geometry 789of the t
- Page 826 and 827:
Applied Bundle Geometry 791The Riem
- Page 828 and 829:
Applied Bundle Geometry 793To compl
- Page 830 and 831:
Applied Bundle Geometry 795Ω 1 :Ω
- Page 832 and 833:
Chapter 5Applied Jet GeometryModern
- Page 834 and 835:
Applied Jet Geometry 799x, with coe
- Page 836 and 837:
Applied Jet Geometry 801s i (x), as
- Page 838 and 839:
Applied Jet Geometry 803It is conve
- Page 840 and 841:
Applied Jet Geometry 805has the 1
- Page 842 and 843:
Applied Jet Geometry 807which split
- Page 844 and 845:
Applied Jet Geometry 809gives the h
- Page 846 and 847:
Applied Jet Geometry 811Furthermore
- Page 848 and 849:
Applied Jet Geometry 813This is a c
- Page 850 and 851:
Applied Jet Geometry 815Due to this
- Page 852 and 853:
Applied Jet Geometry 817The affine
- Page 854 and 855:
Applied Jet Geometry 819In particul
- Page 856 and 857:
Applied Jet Geometry 821Building on
- Page 858 and 859:
Applied Jet Geometry 823on J ∞ (X
- Page 860 and 861:
Applied Jet Geometry 825its tangent
- Page 862 and 863:
Applied Jet Geometry 827Fig. 5.3 Hi
- Page 864 and 865:
Applied Jet Geometry 829It follows
- Page 866 and 867:
Applied Jet Geometry 831affine, whi
- Page 868 and 869:
Applied Jet Geometry 833where Γ i
- Page 870 and 871:
Applied Jet Geometry 8355.6.5 Jacob
- Page 872 and 873:
Applied Jet Geometry 837is also the
- Page 874 and 875:
Applied Jet Geometry 839A generic m
- Page 876 and 877:
Applied Jet Geometry 841where ϑ eH
- Page 878 and 879:
Applied Jet Geometry 843Since E L|
- Page 880 and 881:
Applied Jet Geometry 845Thus, every
- Page 882 and 883:
Applied Jet Geometry 847along u. Ev
- Page 884 and 885:
Applied Jet Geometry 849equations c
- Page 886 and 887:
Applied Jet Geometry 851A Hamiltoni
- Page 888 and 889:
Applied Jet Geometry 853Connections
- Page 890 and 891:
Applied Jet Geometry 855Lagrangian
- Page 892 and 893:
Applied Jet Geometry 857on V ∗ M.
- Page 894 and 895:
Applied Jet Geometry 859contain no
- Page 896 and 897:
Applied Jet Geometry 861(ii) For an
- Page 898 and 899:
Applied Jet Geometry 8635.6.16 Lyap
- Page 900 and 901:
Applied Jet Geometry 865for any t >
- Page 902 and 903:
Applied Jet Geometry 867vertical co
- Page 904 and 905:
Applied Jet Geometry 869difficulty,
- Page 906 and 907:
Applied Jet Geometry 871the energy
- Page 908 and 909:
Applied Jet Geometry 873by the tran
- Page 910 and 911:
Applied Jet Geometry 875The conditi
- Page 912 and 913:
Applied Jet Geometry 877Let Γ = (M
- Page 914 and 915:
Applied Jet Geometry 879geometrical
- Page 916 and 917:
Applied Jet Geometry 881Let (M, I)
- Page 918 and 919:
Applied Jet Geometry 883so referrin
- Page 920 and 921:
Applied Jet Geometry 885The Poincar
- Page 922 and 923:
Applied Jet Geometry 887where N n
- Page 924 and 925:
Applied Jet Geometry 889be a projec
- Page 926 and 927:
Applied Jet Geometry 891to differen
- Page 928 and 929:
Applied Jet Geometry 893for every c
- Page 930 and 931:
Applied Jet Geometry 895vector-fiel
- Page 932 and 933:
Applied Jet Geometry 897This is phr
- Page 934 and 935:
Applied Jet Geometry 899where a, b
- Page 936 and 937:
Applied Jet Geometry 901represent t
- Page 938 and 939:
Applied Jet Geometry 903̂L : J 1 (
- Page 940 and 941:
Applied Jet Geometry 905space J 1 (
- Page 942 and 943:
Applied Jet Geometry 907First of al
- Page 944 and 945:
Applied Jet Geometry 909Therefore,
- Page 946 and 947:
Applied Jet Geometry 911Recall that
- Page 948 and 949:
Applied Jet Geometry 9135.11 Applic
- Page 950 and 951:
Applied Jet Geometry 915the vector
- Page 952 and 953:
Applied Jet Geometry 9175.11.4 Gaug
- Page 954 and 955:
Applied Jet Geometry 9195.11.5 Lagr
- Page 956 and 957:
Applied Jet Geometry 921are represe
- Page 958 and 959:
Applied Jet Geometry 923which are t
- Page 960 and 961:
Applied Jet Geometry 925of this fun
- Page 962 and 963:
Applied Jet Geometry 927a principal
- Page 964 and 965:
Applied Jet Geometry 929Recall that
- Page 966 and 967:
Applied Jet Geometry 931Moreover, t
- Page 968 and 969:
Applied Jet Geometry 933other only
- Page 970 and 971:
Applied Jet Geometry 935In particul
- Page 972 and 973:
Applied Jet Geometry 937Note that,
- Page 974 and 975:
Applied Jet Geometry 939This equali
- Page 976 and 977:
Applied Jet Geometry 941Then, the s
- Page 978 and 979:
Applied Jet Geometry 943In the Lagr
- Page 980 and 981:
Applied Jet Geometry 945Let τ be a
- Page 982 and 983:
Applied Jet Geometry 947Using (5.42
- Page 984 and 985:
Applied Jet Geometry 949associated
- Page 986 and 987:
Applied Jet Geometry 951parts,F α
- Page 988 and 989:
Applied Jet Geometry 953of the Hilb
- Page 990 and 991:
Applied Jet Geometry 955For example
- Page 992 and 993:
Applied Jet Geometry 957the convent
- Page 994 and 995:
Applied Jet Geometry 959left ideal
- Page 996 and 997:
Applied Jet Geometry 961LX → Σ i
- Page 998 and 999:
Applied Jet Geometry 963Levi-Civita
- Page 1000 and 1001:
Applied Jet Geometry 965see this fr
- Page 1002 and 1003:
Applied Jet Geometry 967curves. And
- Page 1004 and 1005:
Applied Jet Geometry 969One sees th
- Page 1006 and 1007:
Applied Jet Geometry 971sums over a
- Page 1008 and 1009:
Applied Jet Geometry 973all this to
- Page 1010 and 1011:
Applied Jet Geometry 975universe ev
- Page 1012 and 1013:
Applied Jet Geometry 977on Σ. If M
- Page 1014 and 1015:
Applied Jet Geometry 979(1) Lorentz
- Page 1016 and 1017:
Applied Jet Geometry 981terpret thi
- Page 1018 and 1019:
Chapter 6Geometrical Path Integrals
- Page 1020 and 1021:
Geometrical Path Integrals and Thei
- Page 1022 and 1023:
Geometrical Path Integrals and Thei
- Page 1024 and 1025:
Geometrical Path Integrals and Thei
- Page 1026 and 1027:
Geometrical Path Integrals and Thei
- Page 1028 and 1029:
Geometrical Path Integrals and Thei
- Page 1030 and 1031:
Geometrical Path Integrals and Thei
- Page 1032 and 1033:
Geometrical Path Integrals and Thei
- Page 1034 and 1035:
Geometrical Path Integrals and Thei
- Page 1036 and 1037:
Geometrical Path Integrals and Thei
- Page 1038 and 1039:
Geometrical Path Integrals and Thei
- Page 1040 and 1041:
Geometrical Path Integrals and Thei
- Page 1042 and 1043:
Geometrical Path Integrals and Thei
- Page 1044 and 1045:
Geometrical Path Integrals and Thei
- Page 1046 and 1047:
Geometrical Path Integrals and Thei
- Page 1048 and 1049:
Geometrical Path Integrals and Thei
- Page 1050 and 1051:
Geometrical Path Integrals and Thei
- Page 1052 and 1053:
Geometrical Path Integrals and Thei
- Page 1054 and 1055:
Geometrical Path Integrals and Thei
- Page 1056 and 1057:
Geometrical Path Integrals and Thei
- Page 1058 and 1059:
Geometrical Path Integrals and Thei
- Page 1060 and 1061:
Geometrical Path Integrals and Thei
- Page 1062 and 1063:
Geometrical Path Integrals and Thei
- Page 1064 and 1065:
Geometrical Path Integrals and Thei
- Page 1066 and 1067:
Geometrical Path Integrals and Thei
- Page 1068 and 1069:
Geometrical Path Integrals and Thei
- Page 1070 and 1071:
Geometrical Path Integrals and Thei
- Page 1072 and 1073:
Geometrical Path Integrals and Thei
- Page 1074 and 1075: Geometrical Path Integrals and Thei
- Page 1076 and 1077: Geometrical Path Integrals and Thei
- Page 1078 and 1079: Geometrical Path Integrals and Thei
- Page 1080 and 1081: Geometrical Path Integrals and Thei
- Page 1082 and 1083: Geometrical Path Integrals and Thei
- Page 1084 and 1085: Geometrical Path Integrals and Thei
- Page 1086 and 1087: Geometrical Path Integrals and Thei
- Page 1088 and 1089: Geometrical Path Integrals and Thei
- Page 1090 and 1091: Geometrical Path Integrals and Thei
- Page 1092 and 1093: Geometrical Path Integrals and Thei
- Page 1094 and 1095: Geometrical Path Integrals and Thei
- Page 1096 and 1097: Geometrical Path Integrals and Thei
- Page 1098 and 1099: Geometrical Path Integrals and Thei
- Page 1100 and 1101: Geometrical Path Integrals and Thei
- Page 1102 and 1103: Geometrical Path Integrals and Thei
- Page 1104 and 1105: Geometrical Path Integrals and Thei
- Page 1106 and 1107: Geometrical Path Integrals and Thei
- Page 1108 and 1109: Geometrical Path Integrals and Thei
- Page 1110 and 1111: Geometrical Path Integrals and Thei
- Page 1112 and 1113: Geometrical Path Integrals and Thei
- Page 1114 and 1115: Geometrical Path Integrals and Thei
- Page 1116 and 1117: Geometrical Path Integrals and Thei
- Page 1118 and 1119: Geometrical Path Integrals and Thei
- Page 1120 and 1121: Geometrical Path Integrals and Thei
- Page 1122 and 1123: Geometrical Path Integrals and Thei
- Page 1126 and 1127: Geometrical Path Integrals and Thei
- Page 1128 and 1129: Geometrical Path Integrals and Thei
- Page 1130 and 1131: Geometrical Path Integrals and Thei
- Page 1132 and 1133: Geometrical Path Integrals and Thei
- Page 1134 and 1135: Geometrical Path Integrals and Thei
- Page 1136 and 1137: Geometrical Path Integrals and Thei
- Page 1138 and 1139: Geometrical Path Integrals and Thei
- Page 1140 and 1141: Geometrical Path Integrals and Thei
- Page 1142 and 1143: Geometrical Path Integrals and Thei
- Page 1144 and 1145: Geometrical Path Integrals and Thei
- Page 1146 and 1147: Geometrical Path Integrals and Thei
- Page 1148 and 1149: Geometrical Path Integrals and Thei
- Page 1150 and 1151: Geometrical Path Integrals and Thei
- Page 1152 and 1153: Geometrical Path Integrals and Thei
- Page 1154 and 1155: Geometrical Path Integrals and Thei
- Page 1156 and 1157: Geometrical Path Integrals and Thei
- Page 1158 and 1159: Geometrical Path Integrals and Thei
- Page 1160 and 1161: Geometrical Path Integrals and Thei
- Page 1162 and 1163: Geometrical Path Integrals and Thei
- Page 1164 and 1165: Geometrical Path Integrals and Thei
- Page 1166 and 1167: Geometrical Path Integrals and Thei
- Page 1168 and 1169: Geometrical Path Integrals and Thei
- Page 1170 and 1171: Geometrical Path Integrals and Thei
- Page 1172 and 1173: Geometrical Path Integrals and Thei
- Page 1174 and 1175:
Geometrical Path Integrals and Thei
- Page 1176 and 1177:
Geometrical Path Integrals and Thei
- Page 1178 and 1179:
Geometrical Path Integrals and Thei
- Page 1180 and 1181:
Geometrical Path Integrals and Thei
- Page 1182 and 1183:
Geometrical Path Integrals and Thei
- Page 1184 and 1185:
Geometrical Path Integrals and Thei
- Page 1186 and 1187:
Geometrical Path Integrals and Thei
- Page 1188 and 1189:
Geometrical Path Integrals and Thei
- Page 1190 and 1191:
Geometrical Path Integrals and Thei
- Page 1192 and 1193:
Geometrical Path Integrals and Thei
- Page 1194 and 1195:
Geometrical Path Integrals and Thei
- Page 1196 and 1197:
Geometrical Path Integrals and Thei
- Page 1198 and 1199:
Geometrical Path Integrals and Thei
- Page 1200 and 1201:
Geometrical Path Integrals and Thei
- Page 1202 and 1203:
Geometrical Path Integrals and Thei
- Page 1204 and 1205:
Geometrical Path Integrals and Thei
- Page 1206 and 1207:
Geometrical Path Integrals and Thei
- Page 1208 and 1209:
Geometrical Path Integrals and Thei
- Page 1210 and 1211:
Geometrical Path Integrals and Thei
- Page 1212 and 1213:
Geometrical Path Integrals and Thei
- Page 1214 and 1215:
Geometrical Path Integrals and Thei
- Page 1216 and 1217:
Geometrical Path Integrals and Thei
- Page 1218 and 1219:
Geometrical Path Integrals and Thei
- Page 1220 and 1221:
Geometrical Path Integrals and Thei
- Page 1222 and 1223:
Geometrical Path Integrals and Thei
- Page 1224 and 1225:
Geometrical Path Integrals and Thei
- Page 1226 and 1227:
Geometrical Path Integrals and Thei
- Page 1228 and 1229:
Geometrical Path Integrals and Thei
- Page 1230 and 1231:
Geometrical Path Integrals and Thei
- Page 1232 and 1233:
Geometrical Path Integrals and Thei
- Page 1234 and 1235:
Geometrical Path Integrals and Thei
- Page 1236 and 1237:
Geometrical Path Integrals and Thei
- Page 1238 and 1239:
Geometrical Path Integrals and Thei
- Page 1240 and 1241:
Geometrical Path Integrals and Thei
- Page 1242 and 1243:
Geometrical Path Integrals and Thei
- Page 1244 and 1245:
Geometrical Path Integrals and Thei
- Page 1246 and 1247:
Geometrical Path Integrals and Thei
- Page 1248 and 1249:
Geometrical Path Integrals and Thei
- Page 1250 and 1251:
Geometrical Path Integrals and Thei
- Page 1252 and 1253:
Geometrical Path Integrals and Thei
- Page 1254 and 1255:
Geometrical Path Integrals and Thei
- Page 1256 and 1257:
Geometrical Path Integrals and Thei
- Page 1258 and 1259:
Geometrical Path Integrals and Thei
- Page 1260 and 1261:
Geometrical Path Integrals and Thei
- Page 1262 and 1263:
Geometrical Path Integrals and Thei
- Page 1264 and 1265:
Geometrical Path Integrals and Thei
- Page 1266 and 1267:
Geometrical Path Integrals and Thei
- Page 1268 and 1269:
Geometrical Path Integrals and Thei
- Page 1270 and 1271:
Geometrical Path Integrals and Thei
- Page 1272 and 1273:
Geometrical Path Integrals and Thei
- Page 1274 and 1275:
Geometrical Path Integrals and Thei
- Page 1276 and 1277:
Geometrical Path Integrals and Thei
- Page 1278 and 1279:
Geometrical Path Integrals and Thei
- Page 1280 and 1281:
Geometrical Path Integrals and Thei
- Page 1282 and 1283:
Geometrical Path Integrals and Thei
- Page 1284 and 1285:
Geometrical Path Integrals and Thei
- Page 1286 and 1287:
Geometrical Path Integrals and Thei
- Page 1288 and 1289:
BibliographyAblowitz, M.J., Clarkso
- Page 1290 and 1291:
Bibliography 1255equations. Springe
- Page 1292 and 1293:
Bibliography 1257connections, Phys.
- Page 1294 and 1295:
Bibliography 1259JHEP 0003:007.Bran
- Page 1296 and 1297:
Bibliography 1261Clementi, C. Petti
- Page 1298 and 1299:
Bibliography 1263Domany, E., Van He
- Page 1300 and 1301:
Bibliography 1265geometrical signat
- Page 1302 and 1303:
Bibliography 1267Bergmann theory of
- Page 1304 and 1305:
Bibliography 1269to Complex Systems
- Page 1306 and 1307:
Bibliography 1271Action for String
- Page 1308 and 1309:
Bibliography 1273Ivancevic, V., Bea
- Page 1310 and 1311:
Bibliography 1275Krener, A. (1984).
- Page 1312 and 1313:
Bibliography 1277Li, M., Vitanyi, P
- Page 1314 and 1315:
Bibliography 1279Nature, 261(5560),
- Page 1316 and 1317:
Bibliography 1281Lambert and R. Gol
- Page 1318 and 1319:
Bibliography 1283dynamics of human
- Page 1320 and 1321:
Bibliography 1285Reshetikhin, N.Yu,
- Page 1322 and 1323:
Bibliography 1287Rev. E, 58, 6333-6
- Page 1324 and 1325:
Bibliography 1289Stasheff, J.D. (19
- Page 1326 and 1327:
Bibliography 1291matical Physics. S
- Page 1328 and 1329:
Bibliography 1293Witten, E. (1991).
- Page 1330 and 1331:
Index1−jet bundle, 8271−jet lif
- Page 1332 and 1333:
Index 1297Chan-Paton factor, 1238Ch
- Page 1334 and 1335:
Index 1299Dirac-Born-Infeld theory,
- Page 1336 and 1337:
Index 1301Gauss, 5Gauss map, 284Gau
- Page 1338 and 1339:
Index 1303isometric, 17isomorphic,
- Page 1340 and 1341:
Index 1305Maxwell Lagrangian, 34Max
- Page 1342 and 1343:
Index 1307probability manifold, 333
- Page 1344 and 1345:
Index 1309super-space, 1215supercov
- Page 1346:
Index 1311Yang-Mills theory, 39, 29
Inappropriate
Loading...
Inappropriate
You have already flagged this document.
Thank you, for helping us keep this platform clean.
The editors will have a look at it as soon as possible.
Mail this publication
Loading...
Embed
Loading...
Delete template?
Are you sure you want to delete your template?
DOWNLOAD ePAPER
This ePaper is currently not available for download.
You can find similar magazines on this topic below under ‘Recommendations’.