Attention! Your ePaper is waiting for publication!
By publishing your document, the content will be optimally indexed by Google via AI and sorted into the right category for over 500 million ePaper readers on YUMPU.
This will ensure high visibility and many readers!
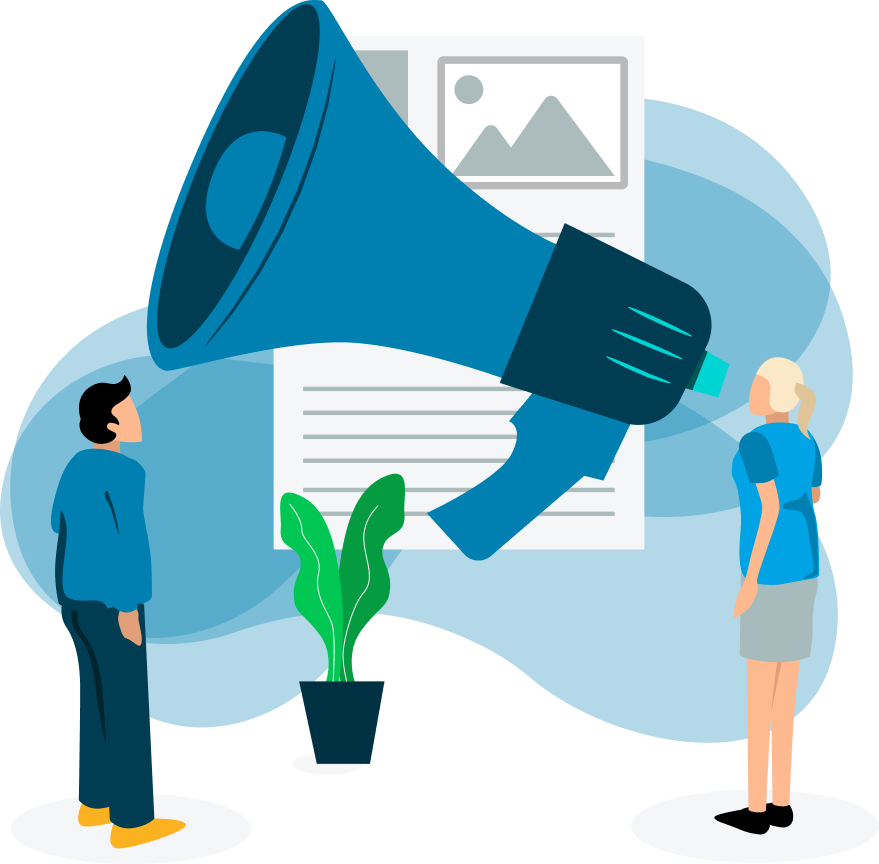
Your ePaper is now published and live on YUMPU!
You can find your publication here:
Share your interactive ePaper on all platforms and on your website with our embed function
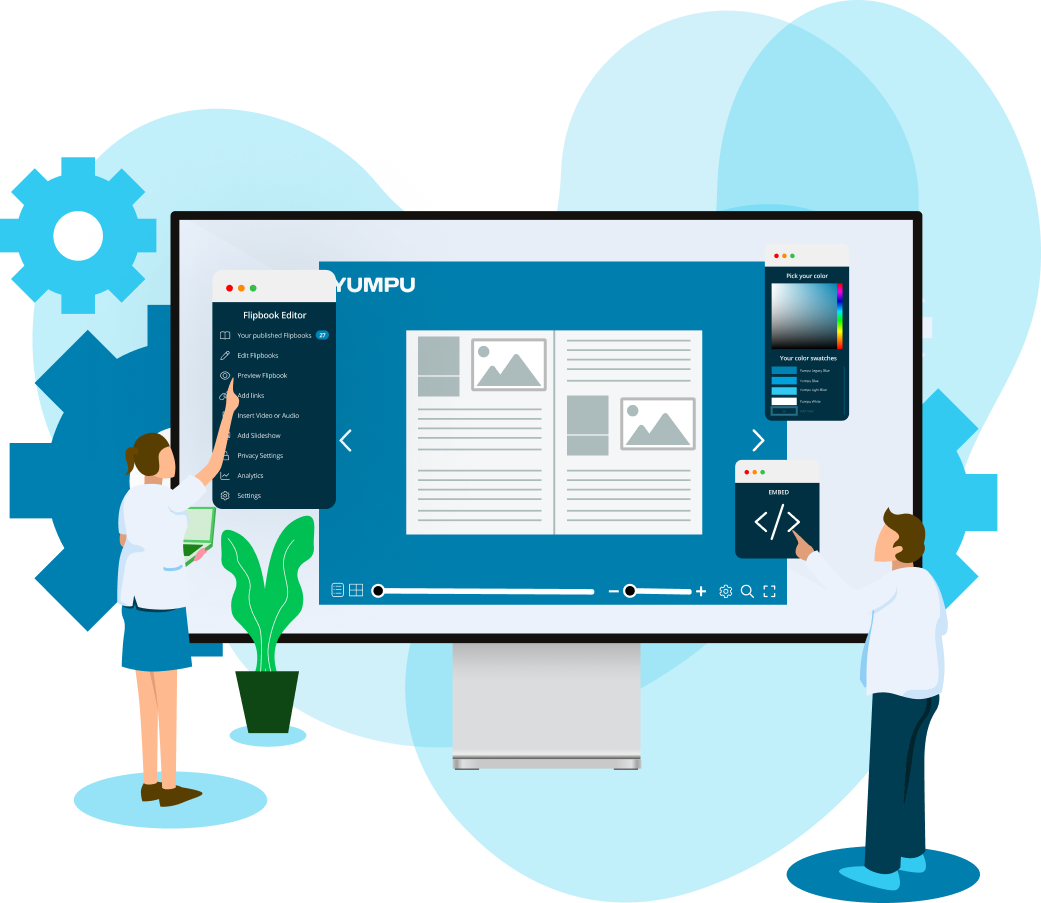
Abstracts Book - IMRC 2018
You also want an ePaper? Increase the reach of your titles
YUMPU automatically turns print PDFs into web optimized ePapers that Google loves.
• SD2-O031 Invited Talk<br />
MACHINE-LEARNING OF CRYSTAL STRUCTURE ENERGY<br />
LANDSCAPES<br />
Richard Hennig 1<br />
1 University of Florida, Materials Science and Engineering, United States.<br />
Evolutionary algorithms provide an efficient method to explore the global<br />
energy landscape of materials and identify low-energy minima but usually rely<br />
on density-functional theory codes to perform such calculations at high<br />
computational cost. To accelerate the structure search, it is desirable to utilize<br />
the data obtained during structural relaxations through the learning of a<br />
surrogate model. We present a machine learning approach for the formation<br />
energies of crystal structures. We explore two types of machine-learning<br />
techniques: kernel-based learning algorithms and artificial neural networks. The<br />
efficiency of machine learning approaches relies on suitable data<br />
representations that encode the relevant physical information about the crystal<br />
structures. We test several physically motivated structure representations. We<br />
show that machine learning of the energy landscape using representations that<br />
encode the radial and angular distribution functions predict the formation<br />
energies of Li-Ge crystals with chemical accuracy and a small prediction error of<br />
20 meV/atom across the composition and structure space. The accuracy<br />
demonstrates the potential of machine-learning models to reduce the<br />
computational cost needed to identify low-energy candidate structures and<br />
improve the performance of the genetic algorithm for structure predictions.<br />
Acknowledgment:<br />
Supported by NSF awards 1440547, 1549132, and 1748464.<br />
Keywords: machine learning, density-functional theory, genetic algorithms<br />
Presenting authors email: rhennig@ufl.edu
• SD2-O031 Invited Talk MACHINE-LEARNING OF CRYSTAL STRUCTURE ENERGY LANDSCAPES Richard Hennig 1 1 University of Florida, Materials Science and Engineering, United States. Evolutionary algorithms provide an efficient method to explore the global energy landscape of materials and identify low-energy minima but usually rely on density-functional theory codes to perform such calculations at high computational cost. To accelerate the structure search, it is desirable to utilize the data obtained during structural relaxations through the learning of a surrogate model. We present a machine learning approach for the formation energies of crystal structures. We explore two types of machine-learning techniques: kernel-based learning algorithms and artificial neural networks. The efficiency of machine learning approaches relies on suitable data representations that encode the relevant physical information about the crystal structures. We test several physically motivated structure representations. We show that machine learning of the energy landscape using representations that encode the radial and angular distribution functions predict the formation energies of Li-Ge crystals with chemical accuracy and a small prediction error of 20 meV/atom across the composition and structure space. The accuracy demonstrates the potential of machine-learning models to reduce the computational cost needed to identify low-energy candidate structures and improve the performance of the genetic algorithm for structure predictions. Acknowledgment: Supported by NSF awards 1440547, 1549132, and 1748464. Keywords: machine learning, density-functional theory, genetic algorithms Presenting authors email: rhennig@ufl.edu
• SD2-O032 Invited Talk LEVERAGING MACHINE LEARNING AND FIRST PRINCIPLES MODELING TO ACCELERATE MATERIALS CHARACTERIZATION AND DESIGN Maria Chan 1 , Arun Mannodi Kanakkithodi 1 , Spencer Hills 1 , Eric Schwenker 1,2 , Fatih Sen 1 , Liang Li 1 , Kendra Letchworth Weaver 1 1 Argonne National Laboratory, Center for Nanoscale Materials, United States. 2 Northwestern University, , United States. Improvements in renewable energy technologies, such as in photovoltaic or catalytic energy conversion, or energy storage, require significant efforts in characterization of existing materials and design of new ones. Advances in firstprinciples theories, algorithms and computational capabilities have enabled efficient and accurate calculations of materials properties first principles. However, first principles modeling requires as inputs atomistic configurations, and the determination of atomistic structures in systems with reduced symmetry, such as nanostructures and defects, is particularly challenging due to the large number of possible configurations. In this talk, we will discuss how physics-informed machine learning is used to combine first principles modeling and materials characterization data, such as x-ray and electron microscopies, to allow for a high-confidence solution of atomistic structure. In addition, we will discuss efforts in improving computational predictions of properties and characterization data, machine learning approaches to develop mapping between structures, characterization signatures, and functional properties. Keywords: Density functional theory, Machine learning, Renewable Energy Materials Presenting authors email: mchan@anl.gov
- Page 1 and 2:
ABSTRACTS BOOK XXVII INTERNATIONAL
- Page 3 and 4:
DIRECTORy GENERAL CHAIR OF THE CONG
- Page 5 and 6:
Symposia A. Nanomaterials and 2D Ma
- Page 7 and 8:
Claudia Gutiérrez Wing SMM Preside
- Page 9 and 10:
Meeting Chairs Cecilia Noguez Insti
- Page 11 and 12:
GENERAL SPONSORs SYMPOSIA SPONSORs
- Page 13 and 14:
29 ICDD - INTERNATIONAL CENTRE FOR
- Page 15 and 16:
Plenary lectures Sunday, August 19t
- Page 17 and 18:
Plenary lectures Monday, August 20t
- Page 19 and 20:
Plenary lectures Tuesday, August 21
- Page 21 and 22:
Plenary lectures Wednesday, August
- Page 23 and 24:
Plenary lectures Thursday, August 2
- Page 25 and 26:
Science, Technology and Society Lec
- Page 27 and 28:
XXXVI
- Page 29 and 30:
Symposium A1 NANOSTRUCTURED CARBON
- Page 31 and 32:
• SA1-O001 Invited Talk GRAPHENE
- Page 33 and 34:
• SA1-O003 ON THE MECHANISM OF HY
- Page 35 and 36:
Keywords: Nanographene, Micropatter
- Page 37 and 38:
• SA1-O006 EXFOLIATION OF GRAPHEN
- Page 39 and 40:
3.- a); Dinesh K. Patel, Vivek Gupt
- Page 41 and 42:
• SA1-O009 SELF-ASSEMBLED THREE-D
- Page 43 and 44:
• SA1-O010 INTERACTION OF POTASSI
- Page 45 and 46:
• SA1-O012 ANTICORROSIVE POLYMER
- Page 47 and 48:
• SA1-O013 Invited Talk POROUS CA
- Page 49 and 50:
• SA1-O014 DESIGN OF INTERCONNECT
- Page 51 and 52:
[1] Ren, Xiaolei, et al. "Iron Phos
- Page 53 and 54:
• SA1-O017 Invited Talk ATOMISTIC
- Page 55 and 56:
• SA1-O018 Invited Talk ENGINEERE
- Page 57 and 58:
• SA1-O019 COMPARATIVE OF THE ADS
- Page 59 and 60:
Acknowledgment: NANOTEC, CONICET, U
- Page 61 and 62:
• SA1-O022 ALL-CARBON SUPERCAPACI
- Page 63 and 64:
• SA1-O024 Invited Talk TAILORING
- Page 65 and 66:
• SA1-O025 SURFACE MODIFICATION O
- Page 67 and 68:
• SA1-O026 CARBON NITRIDE AS NON-
- Page 69 and 70:
• SA1-O027 THEORETICAL STUDY OF G
- Page 71 and 72:
• SA1-O028 HEAVY METAL SENSING US
- Page 73 and 74:
• SA1-O030 MOLECULAR ENCAPSULATIO
- Page 75 and 76:
• SA1-O032 LUMINESCENT CENTRES IN
- Page 77 and 78:
• SA1-O033 OPTICAL PROPERTIES OF
- Page 79 and 80:
• SA1-O035 ROOM TEMPERATURE SUPER
- Page 81 and 82:
• SA1-O036 SINGLE WALLED BII3 AND
- Page 83 and 84:
• SA1-O037 MECHANICAL AND TRIBOLO
- Page 85 and 86:
AFM imaging of the plasma-treated U
- Page 87 and 88:
factor of 5670, respectively. There
- Page 89 and 90:
• SA1-P001 CENTER NITROGEN-VACANC
- Page 91 and 92:
• SA1-P003 MOLECULAR DYNAMICS STU
- Page 93 and 94:
• SA1-P005 HYDROGEN STORAGE ON LI
- Page 95 and 96:
scholarship with CVU 424130. We ack
- Page 97 and 98:
R. Wang, C. Xu, J. Sun, et al. Sci.
- Page 99 and 100:
• SA1-P009 FIRST PRINCIPLES CALCU
- Page 101 and 102:
• SA1-P011 SYNTHESIS AND CHARACTE
- Page 103 and 104:
• SA1-P013 SENSOR OF GRAPHENE BAS
- Page 105 and 106:
• SA1-P015 GRAPHENE-SILVER NANOPA
- Page 107 and 108:
Pérez and Ing. Willian Cauich Ruiz
- Page 109 and 110:
technical help provided by W. Cauic
- Page 111 and 112:
• SA1-P019 ADSORPTION OF CO2 AND
- Page 113 and 114:
Acknowledgment: This work has been
- Page 115 and 116:
Acknowledgment: This work was funde
- Page 117 and 118:
Avanzados, Instituto de Física, UN
- Page 119 and 120:
on the stability and magnetism in m
- Page 121 and 122:
• SA1-P025 CARBON STRUCTURES: AS
- Page 123 and 124:
material. Raman spectra clearly sho
- Page 125 and 126:
• SA1-P028 MOLECULAR MODELING TO
- Page 127 and 128:
• SA1-P030 SYNTHESIS OF N-DOPED G
- Page 129 and 130:
Deyanira Angeles (Chemistry of Mate
- Page 131 and 132:
Keywords: laser-scribed, electroche
- Page 133 and 134:
• SA1-P034 SYNTHESIS AND CHARACTE
- Page 135 and 136:
• SA1-P036 CHARACTERZATION AND PR
- Page 137 and 138:
• SA1-P038 MICROWAVE SYNTHESIS OF
- Page 139 and 140:
• SA1-P040 OPTICAL PROPERTIES OF
- Page 141 and 142:
R. Martínez Flores acknowledges fo
- Page 143 and 144:
• SA1-P043 SILICONE RUBBER COMPOS
- Page 145 and 146:
• SA1-P045 SYNTHESIS OF CARBON NA
- Page 147 and 148:
• SA1-P046 RAMAN SCATTERING AMPLI
- Page 149 and 150:
• SA1-P048 SYNTHESIS AND CHARACTE
- Page 151 and 152:
• SA1-P050 GRAPHENE OXIDE SUPPORT
- Page 153 and 154:
• SA1-P051 COMPARATIVE STUDY OF F
- Page 155 and 156:
• SA1-P053 CARBONS OBTAINED FROM
- Page 157 and 158:
ORAL SESSION
- Page 159 and 160:
• SA2-O002 Invited Talk ON THE IN
- Page 161 and 162:
• SA2-O004 Invited Talk GOOD THIN
- Page 163 and 164:
• SA2-O006 Invited Talk BIMETALLI
- Page 165 and 166:
higher electron conductivity while
- Page 167 and 168:
• SA2-O009 METHANOL REACTIVITY OV
- Page 169 and 170:
• SA2-O011 Invited Talk COMPUTER
- Page 171 and 172:
• SA2-O012 STUDY OF COPPER-PALLAD
- Page 173 and 174:
• SA2-O013 EFFECTS OF LIGAND EXCH
- Page 175 and 176:
• SA2-O014 Invited Talk UTILIZATI
- Page 177 and 178:
1. M. Vandichel, A. Moscu, H. Grön
- Page 179 and 180:
• SA2-O017 Invited Talk REALISTIC
- Page 181 and 182:
• SA2-O018 THERMODYNAMIC STABILIT
- Page 183 and 184:
• SA2-O020 Invited Talk SYNTHESIS
- Page 185 and 186:
• SA2-O021 ON THE STRUCTURE EVOLU
- Page 187 and 188:
• SA2-O023 ATOMIC-SCALE UNDERSTAN
- Page 189 and 190:
Keywords: phase diagram, nanoalloy,
- Page 191 and 192:
• SA2-O026 Invited Talk NANOPARTI
- Page 193 and 194:
• SA2-O027 SYNTHESIS AND CHARACTE
- Page 195 and 196:
POSTER SESSION
- Page 197 and 198:
• SA2-P002 STUDY OF THE OPTICAL A
- Page 199 and 200:
• SA2-P003 MAGNETIC PROPERTIES OF
- Page 201 and 202:
• SA2-P005 XRF SPECTROSCOPY ANALY
- Page 203 and 204:
• SA2-P007 Ag/Pt ALLOYS IN NANOPA
- Page 205 and 206:
• SA2-P008 BISMUTH TELLURIDE THIN
- Page 207 and 208:
• SA2-P009 MICROSTRUCTURAL AND ME
- Page 209 and 210:
• SA2-P010 STUDY OF MECHANICAL BE
- Page 211 and 212:
• SA2-P011 OPTICAL AND STRUCTURAL
- Page 213 and 214:
synthesis. Also to the ph heber vil
- Page 215 and 216:
• SA2-P014 STRUCTURAL CHARACTERIZ
- Page 217 and 218:
Symposium A3 EMERGING 2D NON-GRAPHE
- Page 219 and 220:
• SA3-O001 Invited Talk FUNDAMENT
- Page 221 and 222:
• SA3-O003 Invited Talk CONFORMAL
- Page 223 and 224:
• SA3-O004 STRONG ELECTRONIC MODU
- Page 225 and 226:
• SA3-O006 Invited Talk THE UNIFO
- Page 227 and 228:
Research sponsored by the U.S. Dept
- Page 229 and 230:
Acknowledgment: S.B.L. acknowledges
- Page 231 and 232:
Keywords: Adsorption energy, Bilaye
- Page 233 and 234:
• SA3-O011 ELECTRONIC AND MECHANI
- Page 235 and 236:
• SA3-O013 STRONGLY-BOUND EXCITON
- Page 237 and 238:
• SA3-O015 AB-INITIO STUDY OF THE
- Page 239 and 240:
“building blocks” for 2D crysta
- Page 241 and 242:
• SA3-O018 Invited Talk CREATING
- Page 243 and 244:
Acknowledgment: In situ aberration
- Page 245 and 246:
• SA3-O021 ELECTRON MICROSCOPY OF
- Page 247 and 248:
• SA3-O022 Invited Talk STRUCTURA
- Page 249 and 250:
POSTER SESSION
- Page 251 and 252:
• SA3-P002 CHARGE TRANSPORT IN NO
- Page 253 and 254:
• SA3-P004 ANALYSIS OF ELECTRONIC
- Page 255 and 256:
• SA3-P006 TUNING RYDBERG SERIES
- Page 257 and 258:
• SA3-P007 FABRICATION AND CHARAC
- Page 259 and 260:
Acknowledgment: We are thankful wit
- Page 261 and 262:
providing supercomputing resources
- Page 263 and 264:
• SA3-P011 ON THE STABILITY OF HY
- Page 265 and 266:
• SA3-P013 CALCULATION OF ELECTRO
- Page 267 and 268:
• SA3-P015 DEVELOPMENT OF GRAPHEN
- Page 269 and 270:
• SA3-P016 TOWARDS THE OPTICAL PR
- Page 271 and 272:
Symposium A4 SILICON BASED NANOSCAL
- Page 273 and 274:
• SA4-O002 Invited Talk OPTICAL,
- Page 275 and 276:
• SA4-O003 EVALUATION OF GRAPHENE
- Page 277 and 278:
1 Faraci et al., Giant photolumines
- Page 279 and 280:
• SA4-O006 SURFACE PASSIVATION EF
- Page 281 and 282:
• SA4-O007 Invited Talk CARRIER M
- Page 283 and 284:
• SA4-O008 Invited Talk IS HEXAGO
- Page 285 and 286:
POSTER SESSION
- Page 287 and 288:
[3] Naziruddin Khan, M., Al Dwayyan
- Page 289 and 290:
• SA4-P003 EFFECT OF DRY OXIDATIO
- Page 291 and 292:
• SA4-P005 POLYMORPHOUS SILICON-G
- Page 293 and 294:
• SA4-P007 LUMINESCENT THIN FILMS
- Page 295 and 296:
• SA4-P009 WHITE LUMINESCENCE ACT
- Page 297 and 298:
Acknowledgment: K González want to
- Page 299 and 300:
• SA4-P012 VISIBLE ELECTROLUMINES
- Page 301 and 302:
• SA4-P013 PHOTONIC CRYSTALS TUNE
- Page 303 and 304:
Keywords: Metal assisted chemical e
- Page 305 and 306:
• SA4-P016 STUDY OF PM-SI:H STRUC
- Page 307 and 308:
ORAL SESSION
- Page 309 and 310:
• SA5-O002 SURFACTANT-BASED STRAT
- Page 311 and 312:
ideal H2/H2O ratio preventing as mu
- Page 313 and 314:
Keywords: Plasma Polymer, Nano-arch
- Page 315 and 316:
characteristic of such materials wi
- Page 317 and 318:
• SA5-O007 Invited Talk PHYSICAL
- Page 319 and 320:
• SA5-O008 WEAR RESISTANCE OF Cux
- Page 321 and 322:
• SA5-O010 ENVIRONMENTAL BARRIER
- Page 323 and 324:
• SA5-O012 Invited Talk MAGNETRON
- Page 325 and 326:
This work was supported by the Scie
- Page 327 and 328:
[3] T. A. P. Rocha-Santos, “Senso
- Page 329 and 330:
• SA5-O016 Invited Talk LARGE SCA
- Page 331 and 332:
• SA5-O018 OPTIMIZATION OF MAGNET
- Page 333 and 334:
• SA5-O019 SYNTHESIS AND CHARACTE
- Page 335 and 336:
• SA5-O020 ATOMIC VERSION OF A PI
- Page 337 and 338:
• SA5-O021 Invited Talk HIGH PERF
- Page 339 and 340:
• SA5-P001 NANOSTRUCTURING AND NA
- Page 341 and 342:
• SA5-P003 DESALINATION BY DRAGGI
- Page 343 and 344:
• SA5-P005 CHARACTERIZATION OF TH
- Page 345 and 346:
• SA5-P007 FURTHER ADDITION OF IS
- Page 347 and 348:
Acknowledgment: • Fundação de A
- Page 349 and 350:
• SA5-P010 PERFORMANCE OF SURFACE
- Page 351 and 352:
• SA5-P012 STUDY OF STRUCTURAL PR
- Page 353 and 354:
• SA5-P014 OPTIMIZATION OF INKJET
- Page 355 and 356:
• SA5-P016 BILAYER COATINGS OF VN
- Page 357 and 358:
• SA5-P018 EFFECT OF SUBSTRATE PO
- Page 359 and 360:
• SA5-P020 TRANSPARENT POLYMER FI
- Page 361 and 362:
• SA5-P022 THEORETICAL-EXPERIMENT
- Page 363 and 364:
• SA5-P024 SUSTAINABLE ENERGY SOU
- Page 365 and 366:
• SA5-P026 HYBRID LAYERED NANOPHO
- Page 367 and 368:
Silicon oxide films were deposited
- Page 369 and 370:
ORAL SESSION
- Page 371 and 372:
• SA6-O002 ELECTRONIC THEORY OF C
- Page 373 and 374:
• SA6-O004 Invited Talk EFFICIENT
- Page 375 and 376:
• SA6-O006 THE SYNTHESIS AND CHAR
- Page 377 and 378:
• SA6-O008 SYNTHESIS AND CHARACTE
- Page 379 and 380:
• SA6-O009 SYNTHESIS OF METALLIC
- Page 381 and 382:
Acknowledgment: The authors acknowl
- Page 383 and 384:
• SA6-O012 Invited Talk SYNTHESIS
- Page 385 and 386:
• SA6-O013 OPTICALLY MONODISPERSE
- Page 387 and 388:
• SA6-O014 SYNTHESIS AND MORPHOLO
- Page 389 and 390:
• SA6-O015 TWO-STEP METHODS FOR S
- Page 391 and 392:
• SA6-O017 GREEN SYNTHESIS OF SIL
- Page 393 and 394:
The authors thanks the financial su
- Page 395 and 396:
• SA6-O020 INFLUENCE OF DIFFERENT
- Page 397 and 398:
• SA6-O022 Invited Talk SIGNATURE
- Page 399 and 400:
• SA6-O023 ELECTROMAGNETICALLY IN
- Page 401 and 402:
Advance for Nanoscience and Nanotec
- Page 403 and 404:
thermal characterizations are done
- Page 405 and 406:
• SA6-O027 Invited Talk ADVANCED
- Page 407 and 408:
• SA6-O028 OPTICAL CHARACTERIZATI
- Page 409 and 410:
• SA6-O029 ELECTRIC AND MAGNETIC-
- Page 411 and 412:
• SA6-O030 EFFECT OF APPLIED ELEC
- Page 413 and 414:
[3] S. N. Yadavalli, M. Saphianniko
- Page 415 and 416:
• SA6-O033 CHARACTERIZATION OF TH
- Page 417 and 418:
with high conductivity and superior
- Page 419 and 420:
• SA6-O036 USE OF NANOFLUIDS TO I
- Page 421 and 422:
• SA6-O038 Invited Talk TRANSFORM
- Page 423 and 424:
• SA6-O039 LUMINESCENT PROPERTIES
- Page 425 and 426:
• SA6-O040 REDUCTION AND EXFOLIAT
- Page 427 and 428:
• SA6-O042 GOLD NANOROD FUNCIONAL
- Page 429 and 430:
nitrous oxide quantification. The a
- Page 431 and 432:
• SA6-O045 CHANGES ON THE DISSOCI
- Page 433 and 434:
is found highly hydrophobic meanwhi
- Page 435 and 436:
• SA6-O048 CARBON NANOCOMPOSITES
- Page 437 and 438:
Acknowledgment: I would like to ack
- Page 439 and 440:
POSTER SESSION
- Page 441 and 442:
Aires (CICPBA) and the Universidad
- Page 443 and 444:
• SA6-P003 DIELECTRIC NANOFLUIDS:
- Page 445 and 446:
• SA6-P004 FABRICATION OF A MIS S
- Page 447 and 448:
• SA6-P005 POLYELECTROLYTES WITH
- Page 449 and 450:
• SA6-P007 MECHANICAL PROPERTIES
- Page 451 and 452:
• SA6-P009 SYNTHESIS OF DENDRIMER
- Page 453 and 454:
• SA6-P011 DIELECTRIC FUNCTION AN
- Page 455 and 456:
• SA6-P013 SYNTHESIS OF NEW HYDRO
- Page 457 and 458:
• SA6-P014 SYNTHESIS OF (Bi1.7Pb0
- Page 459 and 460:
• SA6-P015 SUBSTRATES SERS STUDY
- Page 461 and 462:
• SA6-P016 STUDY OF CDS:SB2S3 THI
- Page 463 and 464:
• SA6-P018 OPTICAL, RAMAN AND MIC
- Page 465 and 466:
Acknowledgment: Instituto Politécn
- Page 467 and 468:
• SA6-P021 SYNTHESIS OF SILICON N
- Page 469 and 470:
• SA6-P023 TiO2 POROUS PELLETS SY
- Page 471 and 472:
• SA6-P025 LIGHT EMISSION AND STR
- Page 473 and 474:
• SA6-P027 PREPARATION AND CHARAC
- Page 475 and 476:
• SA6-P028 EFFECT OF DOPANT IN TH
- Page 477 and 478:
• SA6-P029 OPTICAL AND THERMAL PR
- Page 479 and 480:
• SA6-P030 STUDY OF PHOTOLUMINESC
- Page 481 and 482:
[3] Yali Liu. Colloids and Surfaces
- Page 483 and 484:
• SA6-P033 NANOSTRUCTURED KETOROL
- Page 485 and 486:
• SA6-P034 SURFACE MODIFICATION O
- Page 487 and 488:
• SA6-P035 CELL VIABILITY OF A DR
- Page 489 and 490:
• SA6-P037 COMPOSITION AND DIAMET
- Page 491 and 492:
1. C. Rementer, et al., Applied Phy
- Page 493 and 494:
• SA6-P040 SYNTHESIS AND CHARACTE
- Page 495 and 496:
• SA6-P041 SYNTHESIS, SPECTROSCOP
- Page 497 and 498:
• SA6-P042 STUDY OF ELECTRON TRAN
- Page 499 and 500:
• SA6-P044 GA-DOPING IMPACT ON EM
- Page 501 and 502:
• SA6-P046 PHOTOCATALYTIC ACTIVIT
- Page 503 and 504:
• SA6-P048 PHOTOLUMINESCENCE STUD
- Page 505 and 506:
• SA6-P050 EFFECT OF GROWTH TIME
- Page 507 and 508:
• SA6-P052 ELECTRIC FIELD EFFECTS
- Page 509 and 510:
• SA6-P054 SERS ACTIVITY OF HYBRI
- Page 511 and 512:
• SA6-P055 ELECTROCHEMICAL GROWTH
- Page 513 and 514:
• SA6-P057 ENHANCED PHOTOLUMINESC
- Page 515 and 516:
• SA6-P058 GREEN SYNTHESIS AND CH
- Page 517 and 518:
Acknowledgment: The authors want to
- Page 519 and 520:
• SA6-P061 GROWTH OF AN ALUMINUM
- Page 521 and 522:
• SA6-P063 FLUORESCENCE SENSING O
- Page 523 and 524:
• SA6-P065 CO2 ADSORPTION BY MESO
- Page 525 and 526:
• SA6-P067 ATTRIBUTES OF NANOPART
- Page 527 and 528:
• SA6-P068 CTT1 PEPTIDE-LOADED PO
- Page 529 and 530:
• SA6-P070 CONSTRUCTION OF A MESO
- Page 531 and 532:
Acknowledgment: The authors acknowl
- Page 533 and 534:
• SA6-P073 BENDING ENERGY OF 2D M
- Page 535 and 536:
• SA6-P075 THEORETICAL STUDY OF U
- Page 537 and 538:
• SA6-P077 SILVER SULFIDE QUANTUM
- Page 539 and 540:
(CONACyT, INFRA-2012-01-188147), HP
- Page 541 and 542:
• SA6-P080 PRODUCTION OF NANO-SCA
- Page 543 and 544:
induced phase-structure Modificatio
- Page 545 and 546:
• SA6-P083 SYNTHESIS OF POLYMERIC
- Page 547 and 548:
• SA6-P084 ADSORPTION OF ALKALI A
- Page 549 and 550:
• SA6-P086 THEORETICAL STUDY OF N
- Page 551 and 552:
• SA6-P088 ANILINE ADSORPTION AND
- Page 553 and 554:
• SA6-P089 STUDY ON SHORT WAVELEN
- Page 555 and 556:
electrochemical assays, confirming
- Page 557 and 558:
Acknowledgment: Olga Rubi Juárez R
- Page 559 and 560:
• SA6-P093 EVALUATION OF THIOLATE
- Page 561 and 562:
• SA6-P095 SYNTHESIS OF TiO2 NANO
- Page 563 and 564:
• SA6-P097 PDMS NANODOMAINS CONFI
- Page 565 and 566:
• SA6-P099 PRODUCTION OF DIFFEREN
- Page 567 and 568:
• SA6-P101 DEVELOPMENT OF ZEOLITE
- Page 569 and 570:
synthesis of CNTs, nano-spheres and
- Page 571 and 572:
• SA6-P104 FORMATION OF VGA-ON CO
- Page 573 and 574:
• SA6-P106 SYNTHESIS AND CHARACTE
- Page 575 and 576:
• SA6-P107 SYNTHESIS OF NANOSTRUC
- Page 577 and 578:
• SA6-P109 VISIBLE LUMINESCENCE F
- Page 579 and 580:
• SA6-P110 THE EFFECT OF NICKEL D
- Page 581 and 582:
• SA6-P111 CELLULOSE NANOFIBERS T
- Page 583 and 584:
• SA6-P113 THERMODINAMIC STUDY ON
- Page 585 and 586:
• SA6-P114 A NOVEL MICROWAVE METH
- Page 587 and 588:
• SA6-P116 CONTROLLED SYNTHESIS O
- Page 589 and 590:
contaminated with Escherichia Coli
- Page 591 and 592:
• SA6-P119 CHARACTERIZATION OF Mn
- Page 593 and 594:
• SA6-P121 SYNTHESIS OF MAGNETITE
- Page 595 and 596:
• SA6-P123 EASY ROUTE FOR COATING
- Page 597 and 598:
• SA6-P125 GREEN SYNTHESIS OF ZIN
- Page 599 and 600:
• SA6-P127 PHOTOLUMINESCENCE, RAM
- Page 601 and 602:
• SA6-P129 MORPHOLOGICAL STUDY OF
- Page 603 and 604:
Acknowledgment: Universidad Naciona
- Page 605 and 606:
• SA6-P132 AL-DOPING IMPACT ON EM
- Page 607 and 608:
[1] L. Lu, X. Han, J. Li, J. Hua, M
- Page 609 and 610:
• SA6-P135 GROWTH OF SILVER DENDR
- Page 611 and 612:
• SA6-P137 SURFACE ENHANCED RAMAN
- Page 613 and 614:
• SA6-P139 BIOSYNTHESIS OF SILVER
- Page 615 and 616:
• SA6-P141 INTERBAND FORMATION FO
- Page 617 and 618:
Acknowledgment: The work was suppor
- Page 619 and 620:
• SA6-P144 SONOCHEMISTRY PROCESS
- Page 621 and 622:
Romero for support and knowledge on
- Page 623 and 624:
• SA6-P147 MODIFIED NANO-CLAYS WI
- Page 625 and 626:
• SA6-P148 EVALUATION OF THE CURC
- Page 627 and 628:
• SA6-P149 FE-DOPED TIO2 NANOTUBE
- Page 629 and 630:
• SA6-P151 DEVELOPMENT OF NANOSTR
- Page 631 and 632:
• SA6-P152 GROWTH KINETICS AND TH
- Page 633 and 634:
• SA6-P154 QUASI-PERIODIC MULTILA
- Page 635 and 636:
• SA6-P156 SYNTHESIS, CHARACTERIZ
- Page 637 and 638:
• SA6-P158 NANOENCAPSULATION (LIP
- Page 639 and 640:
• SA6-P160 EMISSION AND STRUCTURE
- Page 641 and 642:
• SA6-P162 STUDY OF SELF-ASSEMBLY
- Page 643 and 644:
• SA6-P164 STRUCTURAL CHANGES IN
- Page 645 and 646:
moderate temperatures, short crysta
- Page 647 and 648:
Symposium B1 NEW TRENDS IN POLYMER
- Page 649 and 650:
• SB1-O001 Invited Talk NOVEL, ME
- Page 651 and 652:
• SB1-O003 Invited Talk NAPHTYL-
- Page 653 and 654:
the Office of Basic Energy Science
- Page 655 and 656:
• SB1-O006 SYNTHESIS, CHARACTERIZ
- Page 657 and 658:
• SB1-O008 DETERMINATION OF RADIC
- Page 659 and 660:
• SB1-O010 PERFORMANCE STUDY OF N
- Page 661 and 662:
• SB1-O011 Invited Talk STRUCTURA
- Page 663 and 664:
• SB1-O013 Invited Talk Design an
- Page 665 and 666:
• SB1-O014 Invited Talk PROPERTY
- Page 667 and 668:
• SB1-O015 THE INFLUENCE OF CURE
- Page 669 and 670:
• SB1-O016 POLYMERIZATION REACTOR
- Page 671 and 672:
• SB1-O018 Invited Talk IN SILICO
- Page 673 and 674:
• SB1-O019 Invited Talk SURFACE-
- Page 675 and 676:
• SB1-O021 Invited Talk SYNTHESIS
- Page 677 and 678:
• SB1-O023 Invited Talk GLYCODEND
- Page 679 and 680:
• SB1-O025 Invited Talk TELODENDR
- Page 681 and 682:
• SB1-O027 Invited Talk NANOCELLU
- Page 683 and 684:
• SB1-O029 Invited Talk AMPHIPHIL
- Page 685 and 686:
• SB1-O031 MWCNTs-POLYMER COMPOSI
- Page 687 and 688:
• SB1-O032 SYNTHESIS AND CHARACTE
- Page 689 and 690:
which resulted in a decreased εrev
- Page 691 and 692:
• SB1-O035 IMPLEMENTING AND QUANT
- Page 693 and 694:
• SB1-O036 MULTILEVEL TOPOGRAPHIC
- Page 695 and 696:
POSTER SESSION
- Page 697 and 698:
onds present in the main chain. Thi
- Page 699 and 700:
Acknowledgment: We thank CONACyT fo
- Page 701 and 702:
• SB1-P004 MODIFICATION OF BEADS
- Page 703 and 704:
• SB1-P006 ENCAPSULATION OF ACTIV
- Page 705 and 706:
• SB1-P008 ELABORATION AND CHARAC
- Page 707 and 708:
• SB1-P009 PATTERN RECOGNITION OF
- Page 709 and 710:
• SB1-P010 PREPARATION AND CHARAC
- Page 711 and 712:
• SB1-P011 SYNTHESIS AND CHARACTE
- Page 713 and 714:
electronegativity and low molecular
- Page 715 and 716:
• SB1-P014 EVALUATION OF A MIXED
- Page 717 and 718:
• SB1-P015 THE INTERPRETATION OF
- Page 719 and 720:
• SB1-P017 SYNTHESIS AND CHARACTE
- Page 721 and 722:
• SB1-P019 BINARY GRAFTING OF INT
- Page 723 and 724:
• SB1-P021 SYNTHESIS AND CHARACTE
- Page 725 and 726:
• SB1-P023 STUDY OF THE ASSOCIATI
- Page 727 and 728:
• SB1-P025 MOLECULAR DYNAMICS ANA
- Page 729 and 730:
• SB1-P026 HYBRIDS OF LIPOSOMES F
- Page 731 and 732:
• SB1-P028 DIFFERENTIATION IN GEO
- Page 733 and 734:
• SB1-P030 SYNTHESIS OF L-LYSINE
- Page 735 and 736:
Acknowledgment: a) Consejo Nacional
- Page 737 and 738:
suggest that the method proposed ca
- Page 739 and 740:
CFATA for giving me the opportunity
- Page 741 and 742:
• SB1-P035 NON-EQUILIBRIUM KINETI
- Page 743 and 744:
• SB1-P037 POLYMER NETWORKS CONTA
- Page 745 and 746:
• SB1-P039 EVALUATION OF THE HOMO
- Page 747 and 748:
Acknowledgment: Convocatoria 2018:
- Page 749 and 750:
• SB1-P042 ANALYSIS OF CURING CYC
- Page 751 and 752:
Acknowledgment: CAPES and CNPq Keyw
- Page 753 and 754:
9078). Facultad de Química-UNAM, r
- Page 755 and 756:
References [1] Pardey, A. J., Rojas
- Page 757 and 758:
organic compounds using polymeric i
- Page 759 and 760:
Acknowledgment: We thank CONACyT fo
- Page 761 and 762:
• SB1-P049 CHARACTERIZATION OF NO
- Page 763 and 764:
• SB1-P050 SYNTHESIS AND CHARACTE
- Page 765 and 766:
• SB1-P052 TRIBOLOGICAL AND MECHA
- Page 767 and 768:
• SB1-P053 IMPROVEMENT IN THE HEA
- Page 769 and 770:
friction coefficient was determinat
- Page 771 and 772:
• SB1-P056 CHARACTERIZATION OF TH
- Page 773 and 774:
• SB1-P057 DEVELOPMENT OF A NANOC
- Page 775 and 776:
• SB1-P059 REMOVAL OF BROMOPHENOL
- Page 777 and 778:
• SB1-P061 EFFECT OF THE APPLICAT
- Page 779 and 780:
Acknowledgment: Alvarez-Venicio ack
- Page 781 and 782:
M.D.P. Carreón-Castro, V.M. Veláz
- Page 783 and 784:
• SB1-P065 A SOLUBLE AND CONCDUCT
- Page 785 and 786:
• SB1-P067 SYNTHESIS AND SEMICOND
- Page 787 and 788:
ITO/PEDOT:PSS/COPOLYMER:PC71BM/PFN/
- Page 789 and 790:
addition, the evaluation of the cop
- Page 791 and 792:
• SB1-P071 ELECTROCHEMICAL SYNTHE
- Page 793 and 794:
of carbonate radical (8-10%) for MB
- Page 795 and 796:
• SB1-P074 A NOVEL METHOD FOR SYN
- Page 797 and 798:
the reprocessed samples exhibited a
- Page 799 and 800:
• SB1-P077 SYNTHESIS CHEMICAL AND
- Page 801 and 802:
Keywords: Artificial muscles, elect
- Page 803 and 804:
• SB1-P080 SYNTHESIS AND CHARACTE
- Page 805 and 806:
two methods, favoring conversion to
- Page 807 and 808:
• SB1-P083 EFFECT OF THE CHEMICAL
- Page 809 and 810:
considering low NaOH:PG(-OH) molar
- Page 811 and 812:
and IN 203517) and from CONACYT Con
- Page 813 and 814:
• SB1-P087 SYNTHESIS AND CHARACTE
- Page 815 and 816:
• SB1-P089 SYNTHESIS AND CHARACTE
- Page 817 and 818:
• SB1-P091 EMULSION POLYMERIZATIO
- Page 819 and 820:
• SB1-P092 EFFECT OF THE CONCENTR
- Page 821 and 822:
• SB1-P094 ACCELERATED DEGRADATIO
- Page 823 and 824:
Keywords: soft matter, applications
- Page 825 and 826:
• SB1-P097 EFFECTS AND APPLICATIO
- Page 827 and 828:
• SB1-P098 LINEAR VISCOELASTICITY
- Page 829 and 830:
• SB1-P099 FOLLOWING THE BEHAVIOR
- Page 831 and 832:
• SB1-P100 MOLECULAR DYNAMICS SIM
- Page 833 and 834:
• SB1-P101 THERMODYNAMIC STUDY OF
- Page 835 and 836:
The increase in the permitivity can
- Page 837 and 838:
ORAL SESSION
- Page 839 and 840:
• SB2-O002 NANOPARTICLES FOR BIOM
- Page 841 and 842:
• SB2-O004 HIGHLY-STABLE SOFTENIN
- Page 843 and 844:
• SB2-O006 MICROSTRUCTURAL CHARAC
- Page 845 and 846:
colloid particles between CS-OA and
- Page 847 and 848:
• SB2-O009 A CHEMICAL ANALYSIS OF
- Page 849 and 850:
• SB2-O011 EFFECT OF THE CRYSTAL
- Page 851 and 852:
• SB2-O013 SYNTHESIS OF NYLON 6 B
- Page 853 and 854:
contributed to the development of b
- Page 855 and 856:
• SB2-O016 SYNTHESIS AND CHARACTE
- Page 857 and 858:
• SB2-O018 HIGH THROUGHPUT TOXICI
- Page 859 and 860:
• SB2-O020 STRUCTURAL-BASED STRAT
- Page 861 and 862:
• SB2-O022 ANTIMICROBIAL WOUND DR
- Page 863 and 864:
• SB2-O024 FIBROUS MATS BASED ON
- Page 865 and 866:
• SB2-O025 SYNTHESIS OF NANOCRYST
- Page 867 and 868:
• SB2-P001 DESIGN OF A BIOMATERIA
- Page 869 and 870:
• SB2-P002 PHOTO CONTROLLED DYNAM
- Page 871 and 872:
Acknowledgment: To the project at U
- Page 873 and 874:
• SB2-P005 EFFECT OF MODIFICATION
- Page 875 and 876:
• SB2-P007 TEOS-PVA/AGNPS HYDROGE
- Page 877 and 878:
• SB2-P009 NANOCELLULOSE TEMPLATE
- Page 879 and 880:
• SB2-P011 VERTICAL NANOWIRES ENH
- Page 881 and 882:
• SB2-P013 MAGNETO-LUMINESCENT GA
- Page 883 and 884:
Finally, owing to the cathodolumine
- Page 885 and 886:
• SB2-P016 BIOCOMPATIBILITY STUDY
- Page 887 and 888:
With only three immunizations, a ma
- Page 889 and 890:
These results suggest that naked Fe
- Page 891 and 892:
• SB2-P020 PHYSICOCHEMICAL CHARAC
- Page 893 and 894:
• SB2-P021 SURFACE ENHANCED RAMAN
- Page 895 and 896:
• SB2-P023 SYNTHESIS OF HOLLOW NA
- Page 897 and 898:
29: 2543-2564, 2008. PMID: 18470966
- Page 899 and 900:
• SB2-P026 MOLECULAR SIMULATION O
- Page 901 and 902:
elease. These nanocarriers represen
- Page 903 and 904:
• SB2-P029 STRUCTURAL AND PHOTOLU
- Page 905 and 906:
• SB2-P031 DESIGN AND MICROSTRUCT
- Page 907 and 908:
• SB2-P032 CRYSTALLIZATION AND GL
- Page 909 and 910:
Summary: Heart Disease and Stroke S
- Page 911 and 912:
[1] Patel, D. K., Singh, R. K., Sin
- Page 913 and 914:
• SB2-P036 ISOLATION AND CHARACTE
- Page 915 and 916:
• SB2-P038 SURFACE CHARACTERIZATI
- Page 917 and 918:
• SB2-P040 ANALYSIS AND FRACTAL D
- Page 919 and 920:
• SB2-P042 SYNTHESIS AND PROPERTI
- Page 921 and 922:
• SB2-P044 BIOMIMETIC PVA NANOPAR
- Page 923 and 924:
• SB2-P046 STUDY OF THE HYDROXYAP
- Page 925 and 926:
• SB2-P047 SPARK PLASMA EXTRUSION
- Page 927 and 928:
• SB2-P049 RHEOLOGICAL EFFECT OF
- Page 929 and 930:
• SB2-P050 CHARACTERIZATION OF BR
- Page 931 and 932:
• SB2-P052 POLYMER-NANOSTRUCTURED
- Page 933 and 934:
• SB2-P054 OPTICAL PROPERTIES MOD
- Page 935 and 936:
ORAL SESSION
- Page 937 and 938:
espectively. Based on the results,
- Page 939 and 940:
• SB3-O003 Invited Talk NANODIAMO
- Page 941 and 942:
also to thanks to the University of
- Page 943 and 944:
Acknowledgment: We would like to th
- Page 945 and 946:
NMSC. The study will continue as MT
- Page 947 and 948:
esults depict the DN hydrogels unde
- Page 949 and 950:
• SB3-O009 SCAFFOLD FOR TISSUE EN
- Page 951 and 952:
• SB3-O011 ELECTRIC STIMULATION I
- Page 953 and 954:
POSTER SESSION
- Page 955 and 956:
• SB3-P002 ANALYSIS AND TRANSFORM
- Page 957 and 958:
This work was funded by CONACyT (Me
- Page 959 and 960:
Acknowledgment: The authors would l
- Page 961 and 962:
transported to the brain, increasin
- Page 963 and 964:
Keywords: Tissue Engineering, Cardi
- Page 965 and 966:
Keywords: Tissue Engineering, 2D an
- Page 967 and 968:
CAPES and CNPq Keywords: SUTURE, 3D
- Page 969 and 970:
Keywords: Biomaterials for Tissue E
- Page 971 and 972:
in relation to the negative control
- Page 973 and 974:
ORAL SESSION
- Page 975 and 976:
a hybrid perovskite single crystal
- Page 977 and 978:
• SB4-O003 Invited Talk THIN FILM
- Page 979 and 980:
• SB4-O005 CDS THIN FILMS/CDS NPS
- Page 981 and 982:
• SB4-O006 EFFECTIVE PARAMETERS F
- Page 983 and 984:
• SB4-O008 Invited Talk NEW DESIG
- Page 985 and 986:
• SB4-O010 Invited Talk POLYSULFI
- Page 987 and 988:
• SB4-O012 Invited Talk FLEXIBLE
- Page 989 and 990:
• SB4-O014 TRANSFER PRINTING OF I
- Page 991 and 992:
• SB4-O016 MULTILAYER STUDY ON PU
- Page 993 and 994:
• SB4-O018 STUDY OF THE ELECTRIC
- Page 995 and 996:
• SB4-O020 Invited Talk ENGINEERI
- Page 997 and 998:
• SB4-O022 PIEZORESISTIVE AND THE
- Page 999 and 1000:
• SB4-O023 STUDY OF THE THERMOELE
- Page 1001 and 1002:
• SB4-O025 Invited Talk CONSIDERA
- Page 1003 and 1004:
• SB4-P001 STUDY OF CuS THIN FILM
- Page 1005 and 1006:
• SB4-P003 MORPHOLOGICAL EFFECTS
- Page 1007 and 1008:
• SB4-P005 FUNCTIONAL TRANSPARENT
- Page 1009 and 1010:
• SB4-P007 SYNTHESIS OF PENTACENE
- Page 1011 and 1012:
• SB4-P009 OPTOELECTRONIC PROPERT
- Page 1013 and 1014:
• SB4-P011 ENHANCEMENT OF PHOTOLU
- Page 1015 and 1016:
• SB4-P013 BENDING CYCLES STUDY O
- Page 1017 and 1018:
• SB4-P014 STUDY OF PL FOR THIN F
- Page 1019 and 1020:
Keywords: GaN, Thin Film Transistor
- Page 1021 and 1022:
• SB4-P017 SYNTHESIS AND CHARACTE
- Page 1023 and 1024:
• SB4-P019 SYNTHESIS OF ZrO2-PS H
- Page 1025 and 1026:
• SB4-P021 CuO THIN FILMS BY THE
- Page 1027 and 1028:
• SB4-P023 SYNTHESIS AND CHARACTE
- Page 1029 and 1030:
• SB4-P025 STUDY OF PbS THIN FILM
- Page 1031 and 1032:
• SB4-P026 EFFECT OF DEPOSITION R
- Page 1033 and 1034:
• SB4-P027 STUDY OF OPTICAL, MICR
- Page 1035 and 1036:
• SB4-P028 SYNTHESIS AND CHARACTE
- Page 1037 and 1038:
Symposium B5 FUNCTIONAL SURFACES AN
- Page 1039 and 1040:
• SB5-O001 Invited Talk NANOFIBRI
- Page 1041 and 1042:
• SB5-O003 LAYER-BY-LAYER ENCAPSU
- Page 1043 and 1044:
have shown that bacteria were compl
- Page 1045 and 1046:
• SB5-O006 Invited Talk MOLECULAR
- Page 1047 and 1048:
Acknowledgment: Thanks to Instituto
- Page 1049 and 1050:
• SB5-O009 NOVEL HYBRID-SEMICONDU
- Page 1051 and 1052:
• SB5-O011 Invited Talk MICROENGI
- Page 1053 and 1054:
• SB5-O012 IMMUNOMODULATION ON TH
- Page 1055 and 1056:
• SB5-O013 LIPOAMINO ACID: TWO-ST
- Page 1057 and 1058:
• SB5-O015 Invited Talk PROTEIN A
- Page 1059 and 1060:
• SB5-O016 FOCUSED ELECTRON AND I
- Page 1061 and 1062:
Acknowledgment: The UKs EPSRC is gr
- Page 1063 and 1064:
• SB5-O019 SUFACE BIOFUNCTIONALIZ
- Page 1065 and 1066:
• SB5-O021 N-(AMINOALKYL)-BIOTINA
- Page 1067 and 1068:
other treatments. These results all
- Page 1069 and 1070:
• SB5-O024 INFLUENCE OF THE SURFA
- Page 1071 and 1072:
POSTER SESSION
- Page 1073 and 1074:
food in Mexico. Agroecology and Sus
- Page 1075 and 1076:
• SB5-P003 CALCIUM-INDUCED TUBULI
- Page 1077 and 1078:
• SB5-P004 BACTERICIDAL BEHAVIOR
- Page 1079 and 1080:
the catalytic activity of the graph
- Page 1081 and 1082:
• SB5-P007 SYNTHESIS AND CHARACTE
- Page 1083 and 1084:
• SB5-P009 PHYSICAL CHEMICAL STUD
- Page 1085 and 1086:
• SB5-P010 EFFECT OF CHITOSAN-COA
- Page 1087 and 1088:
A special thanks to the PIIT nanote
- Page 1089 and 1090:
Carrier mixes allowed the probiotic
- Page 1091 and 1092:
• SB5-P014 DEVELOPMENT OF ANTIFOU
- Page 1093 and 1094:
Symposium B6 MATERIALS FOR MEDICAL
- Page 1095 and 1096:
• SB6-O001 Invited Talk SYNTHETIC
- Page 1097 and 1098:
In conclusion, we were able to fabr
- Page 1099 and 1100:
Acknowledgment: Grant MAT2015-66666
- Page 1101 and 1102:
• SB6-O005 DEVELOPMENT AND CHARAC
- Page 1103 and 1104:
Microscopy, Energy-Dispersive X-ray
- Page 1105 and 1106:
• SB6-O008 SYNTHESIS AND CHARACTE
- Page 1107 and 1108:
• SB6-O010 Invited Talk TOWARDS D
- Page 1109 and 1110:
ased dental implants chemically cor
- Page 1111 and 1112:
• SB6-O013 Invited Talk NEW PERSP
- Page 1113 and 1114:
• SB6-O015 INFLUENCE OF THE PROJE
- Page 1115 and 1116:
Moreira Teixeira, Patterson J, Luyt
- Page 1117 and 1118:
strength both in-vitro and in-vivo
- Page 1119 and 1120:
Acknowledgment: The authors have re
- Page 1121 and 1122:
iron were determined. The tissues w
- Page 1123 and 1124:
• SB6-O021 GOLD FLUORESCENT NANO-
- Page 1125 and 1126:
colon cancer, this system being muc
- Page 1127 and 1128:
• SB6-O024 Invited Talk POLYMER B
- Page 1129 and 1130:
ole for sarcosine in prostate cance
- Page 1131 and 1132:
In conclussion, the direct grafting
- Page 1133 and 1134:
• SB6-O028 LIGHT SHEET MICROSCOPY
- Page 1135 and 1136:
• SB6-O030 LANGMUIR MONOLAYERS AS
- Page 1137 and 1138:
• SB6-O031 POLYMER DECORATED PLAS
- Page 1139 and 1140:
• SB6-O033 PREPARATION AND CHARAC
- Page 1141 and 1142:
• SB6-O034 NANOCAVITATED TITANIUM
- Page 1143 and 1144:
• SB6-O035 PREPARATION AND IN VIT
- Page 1145 and 1146:
POSTER SESSION
- Page 1147 and 1148:
• SB6-P002 DEVELOPMENT AND CHARAC
- Page 1149 and 1150:
de la Provincia de Buenos Aires (CI
- Page 1151 and 1152:
as MR and fluorescence imaging agen
- Page 1153 and 1154:
• SB6-P006 CONTROLLED BACTERICIDE
- Page 1155 and 1156:
• SB6-P008 FRACTURE STRENGTH OF P
- Page 1157 and 1158:
• SB6-P010 EFFECT OF THE BIOABSOR
- Page 1159 and 1160:
2. Gautam R. Desiraju; Crystal Engi
- Page 1161 and 1162:
• SB6-P013 DEVELOPMENT OF MULTIFU
- Page 1163 and 1164:
• SB6-P014 GREEN SYNTHESIS OF SIL
- Page 1165 and 1166:
• SB6-P016 PLASMA OXIDATION OF A
- Page 1167 and 1168:
• SB6-P018 WEAR EFFECT ON THE REA
- Page 1169 and 1170:
• SB6-P020 INHIBITION OF THE ADHE
- Page 1171 and 1172:
• SB6-P022 EVALUATION OF ANTIBACT
- Page 1173 and 1174:
• SB6-P024 PREVALENCE OF SILVER R
- Page 1175 and 1176:
• SB6-P026 HYDROTALCITES INTERCAL
- Page 1177 and 1178:
• SB6-P028 STRUCTURE-STABILITY RE
- Page 1179 and 1180:
• SB6-P030 DEVELOPMENT OF A BIOSE
- Page 1181 and 1182:
• SB6-P032 CYTOTOXICITY ASSAYS ON
- Page 1183 and 1184:
• SB6-P034 APPLICATION OF THERMOS
- Page 1185 and 1186:
• SB6-P036 UPSIDE-DOWN JELLYFISH
- Page 1187 and 1188:
• SB6-P037 RADIATION-GRAFTING OF
- Page 1189 and 1190:
Acknowledgment: The present study w
- Page 1191 and 1192:
• SB6-P040 ELECTROSPINNING TECHNI
- Page 1193 and 1194:
• SB6-P042 SYNTHESIS AND CHARACTE
- Page 1195 and 1196:
Acknowledgment: The authors want to
- Page 1197 and 1198:
• SB6-P045 COMPARISON OF TWO METH
- Page 1199 and 1200:
• SB6-P046 PRINTABLE HYDROGELS FO
- Page 1201 and 1202:
prostheses: Overview and future pro
- Page 1203 and 1204:
Acknowledgment: The present work wa
- Page 1205 and 1206:
• SB6-P050 DEVELOPMENT AND DETERM
- Page 1207 and 1208:
• SB6-P052 CHARACTERIZATION AND E
- Page 1209 and 1210:
• SB6-P053 DESIGN AND IN VITRO EV
- Page 1211 and 1212:
• SB6-P054 PLLGA COPOLYMERS: A DE
- Page 1213 and 1214:
• SB6-P056 TAPIOCA STARCH-GALACTO
- Page 1215 and 1216:
• SB6-P057 PREPARATION AND CHARAC
- Page 1217 and 1218:
• SB6-P058 FORMATION AND EVALUATI
- Page 1219 and 1220:
• SB6-P059 ADDITION OF GALACTOMAN
- Page 1221 and 1222:
nixtamalization process. Amylose-li
- Page 1223 and 1224:
• SB6-P062 SYNTESIS AND CHARACTER
- Page 1225 and 1226:
experiments in the presence of DNA
- Page 1227 and 1228:
Acknowledgment: The authors would l
- Page 1229 and 1230:
• SB6-P066 LIPOSOMAL FORMULATION
- Page 1231 and 1232:
• SB6-P067 STUDY OF NIOSOMAS THER
- Page 1233 and 1234:
Osteoblast microencapsulation using
- Page 1235 and 1236:
• SB6-P070 IN VITRO BIOACTIVITY A
- Page 1237 and 1238:
• SB6-P072 SONIC GUIDE FOR BLIND
- Page 1239 and 1240:
PLGA(PTX) is a suitable therapeutic
- Page 1241 and 1242:
Acknowledgment: The authors would l
- Page 1243 and 1244:
performance in photo-thermal ablati
- Page 1245 and 1246:
• SB6-P077 STUDY OF THE APPLICATI
- Page 1247 and 1248:
• SB6-P079 ASSEMBLY AND PHYSICOCH
- Page 1249 and 1250:
• SB6-P080 SYNTHESIS OF OLIGOMER
- Page 1251 and 1252:
according to the device dimensions
- Page 1253 and 1254:
• SB6-P083 STUDY OF TiO2 AND SiO2
- Page 1255 and 1256:
• SB6-P085 SYNTHESIS OF NANOMEDIC
- Page 1257 and 1258:
REFERENCES: 1.- Santamaría Gonzál
- Page 1259 and 1260:
• SB6-P088 MICELLAR PHASE BEHAVIO
- Page 1261 and 1262:
• SB6-P090 AMOXICILLIN CONTROLLED
- Page 1263 and 1264:
nm laser produces temperature incre
- Page 1265 and 1266:
• SB6-P093 MAGNETIC NANOPARTICLES
- Page 1267 and 1268:
• SB6-P094 MAGNETIC PROPERTIES IN
- Page 1269 and 1270:
• SB6-P095 MICROWAVE-ASSISTED SYN
- Page 1271 and 1272:
grant INFR-2014-01-225455 to acquir
- Page 1273 and 1274:
Tecnología (CONACyT) Mexico for gr
- Page 1275 and 1276:
• SB6-P099 SONOCHEMICAL SYNTHESIS
- Page 1277 and 1278:
• SB6-P100 UP-CONVERSION NANOMATE
- Page 1279 and 1280:
• SB6-P101 NANOCOMPOSITE OF GRAPH
- Page 1281 and 1282:
• SB6-P103 GOLD NANOSTARS FOR TEN
- Page 1283 and 1284:
• SB6-P104 ADSORPTION AND SELF-AS
- Page 1285 and 1286:
loaded methodologies, i.e. during p
- Page 1287 and 1288:
• SB6-P107 FABRICATION AND STUDY
- Page 1289 and 1290:
Symposium C1 ELECTROCHEMICAL ENERGY
- Page 1291 and 1292:
• SC1-O001 Invited Talk MATERIALS
- Page 1293 and 1294:
compositions of Li and Na hydroxyl
- Page 1295 and 1296:
Acknowledgment: This work was parti
- Page 1297 and 1298:
• SC1-O005 NOVEL AMORPHOUS METAL
- Page 1299 and 1300:
• SC1-O007 APPLICATION OF NEW MET
- Page 1301 and 1302:
• SC1-O008 THERMODYNAMICS OF INTE
- Page 1303 and 1304:
• SC1-O010 PtPd/C BIMETALLIC NANO
- Page 1305 and 1306:
• SC1-O012 SYNTHESIS AND CHARACTE
- Page 1307 and 1308:
The authors thankful acknowledges t
- Page 1309 and 1310:
• SC1-O015 FACILE PREPARATION OF
- Page 1311 and 1312:
• SC1-O017 EFFECT OF THE OAM/OAC
- Page 1313 and 1314:
• SC1-O018 NEW 2D MATERIALS DESIG
- Page 1315 and 1316:
• SC1-O020 NANOCATALYST OF CuNiPt
- Page 1317 and 1318:
• SC1-P001 SUPERCAPACITIVE BEHAVI
- Page 1319 and 1320:
• SC1-P003 DEVELOPMENT OF HIGH SU
- Page 1321 and 1322:
• SC1-P005 STRUCTURAL AND ELECTRI
- Page 1323 and 1324:
• SC1-P007 COMPOSITE OF SULFUR AN
- Page 1325 and 1326:
• SC1-P008 3D PRINTED ASYMMETRIC
- Page 1327 and 1328:
• SC1-P009 CHICKEN MANURE-DERIVED
- Page 1329 and 1330:
more negative potentials than the t
- Page 1331 and 1332:
• Instituto para la Investigació
- Page 1333 and 1334:
• SC1-P013 USE OF BIOCARBONS-BASE
- Page 1335 and 1336:
• SC1-P014 OCTYLAMINE STABILIZED
- Page 1337 and 1338:
• SC1-P016 SYNTHESIS OF Mn DOPED
- Page 1339 and 1340:
• SC1-P017 SYNTHESIS OF SULFONATE
- Page 1341 and 1342:
Acknowledgment: RMB (366093) thanks
- Page 1343 and 1344:
• SC1-P020 SYNTHESIS OF ELECTROCE
- Page 1345 and 1346:
• SC1-P022 HYDROGEN PRODUCTION WI
- Page 1347 and 1348:
• SC1-P024 SYNTHESIS OF ACTIVATED
- Page 1349 and 1350:
• SC1-P026 CHARACTERIZATION OF Ni
- Page 1351 and 1352:
• SC1-P027 STUDY OF THE SYNTHESIS
- Page 1353 and 1354:
• SC1-P028 ELECTROCHEMICAL CHARAC
- Page 1355 and 1356:
Symposium C2 CHALLENGES IN MATERIAL
- Page 1357 and 1358:
• SC2-O001 Invited Talk MULTIFUNC
- Page 1359 and 1360:
• SC2-O003 Invited Talk ADVANCES
- Page 1361 and 1362:
• SC2-O005 Invited Talk PEROVSKIT
- Page 1363 and 1364:
• SC2-O006 Invited Talk DEVELOPME
- Page 1365 and 1366:
• SC2-O008 Invited Talk TUNING ME
- Page 1367 and 1368:
• SC2-O009 Invited Talk CHARGE ST
- Page 1369 and 1370:
• SC2-O011 Invited Talk GRAPHENE
- Page 1371 and 1372:
halide perovskite deposition and ch
- Page 1373 and 1374:
Acknowledgment: NANOTEC, CONICET, U
- Page 1375 and 1376:
co-financed by the European Regiona
- Page 1377 and 1378:
• SC2-O016 Invited Talk MICROSTRU
- Page 1379 and 1380:
• SC2-O018 Invited Talk HOT ELECT
- Page 1381 and 1382:
• SC2-O019 Invited Talk MATERIALS
- Page 1383 and 1384:
• SC2-O021 Invited Talk COMPUTATI
- Page 1385 and 1386:
• SC2-O023 EFFICIENT ELECTRICITY
- Page 1387 and 1388:
• SC2-O024 DEVELOPMENT OF PHASE C
- Page 1389 and 1390:
• SC2-O025 SUITABILITY OF ALUMINI
- Page 1391 and 1392:
• SC2-O027 Co7Sb2O12 SPINEL LIKE
- Page 1393 and 1394:
• SC2-O028 FLAT MIRRORS WITH MULT
- Page 1395 and 1396:
Ph, D. NIEN FARRERA VAZQUEZ. Ph, D.
- Page 1397 and 1398:
• SC2-O031 STUDY OF THE TRANSITIO
- Page 1399 and 1400:
• SC2-O032 EMPLASTE DE YESO DIATE
- Page 1401 and 1402:
• SC2-O034 Invited Talk ENERGY EF
- Page 1403 and 1404:
• SC2-O035 Invited Talk STEADY-ST
- Page 1405 and 1406:
• SC2-O037 CUBO DE LA VIDA Wilfre
- Page 1407 and 1408:
POSTER SESSION
- Page 1409 and 1410:
• SC2-P002 HYDROXYLATION OF LITHI
- Page 1411 and 1412:
• SC2-P003 Y-DOPED CERIA AND Y-DO
- Page 1413 and 1414:
4. The local entropy generation dec
- Page 1415 and 1416:
• SC2-P006 NANOCLUSTERS OF VANADI
- Page 1417 and 1418:
• SC2-P007 USE OF A GEOPOLYMER FO
- Page 1419 and 1420:
• SC2-P009 ADSORPTION OF H2 MOLEC
- Page 1421 and 1422:
• SC2-P011 DYNAMIC HEAT TRANSFER
- Page 1423 and 1424:
• SC2-P012 HIGH PERFORMANCE BCTZ-
- Page 1425 and 1426:
• SC2-P014 PHOTOELECTRODEPOSITION
- Page 1427 and 1428:
• SC2-P016 DEVELOPMENT OF NANOMET
- Page 1429 and 1430:
• SC2-P017 EFFECT OF THE Sb 5+ CA
- Page 1431 and 1432:
• SC2-P019 DEVELOPMENT OF SAMARIU
- Page 1433 and 1434:
• SC2-P020 SYNTHESIS AND CHARACTE
- Page 1435 and 1436:
• SC2-P022 EVALUATION OF TEMPERAT
- Page 1437 and 1438:
• SC2-P024 DEVELOPMENT OF A BAR F
- Page 1439 and 1440:
obtained by fitting the time traces
- Page 1441 and 1442:
ORAL SESSION
- Page 1443 and 1444:
• SC3-O002 Invited Talk PULSE LAS
- Page 1445 and 1446:
Func. Mater. 27, 1401468 (2017); [2
- Page 1447 and 1448:
• SC3-O005 EXPERIMENTAL AND THEOR
- Page 1449 and 1450:
• SC3-O006 Invited Talk SCULPTING
- Page 1451 and 1452:
• SC3-O008 Invited Talk SEMICONDU
- Page 1453 and 1454:
• SC3-O010 Invited Talk RENWABLE
- Page 1455 and 1456:
• SC3-O012 Invited Talk PHOTOELEC
- Page 1457 and 1458:
• SC3-O014 PHOTOCATALYTIC HYDROGE
- Page 1459 and 1460:
• SC3-O015 Invited Talk UNDERSTAN
- Page 1461 and 1462:
• SC3-O017 Invited Talk JET FUEL
- Page 1463 and 1464:
• SC3-O019 Invited Talk CONTSTRUC
- Page 1465 and 1466:
Integration, and Function Enabling,
- Page 1467 and 1468:
• SC3-P001 DYNAMIC MODEL FOR THE
- Page 1469 and 1470:
• SC3-P003 SOLAR WATER SPLITTING
- Page 1471 and 1472:
• SC3-P005 KINETIC CONSTANTS ASSO
- Page 1473 and 1474:
• SC3-P007 EVALUATION OF NICKEL E
- Page 1475 and 1476:
• SC3-P009 NICKEL-COBALT MIXED OX
- Page 1477 and 1478:
• SC3-P010 COBALT-PHOSPHATE COMPL
- Page 1479 and 1480:
ORAL SESSION
- Page 1481 and 1482:
• SC4-O002 Invited Talk NOVEL ORG
- Page 1483 and 1484:
• SC4-O003 SYNTHESIS OF REGIOREGU
- Page 1485 and 1486:
• SC4-O004 A HIGH OPEN CIRCUIT VO
- Page 1487 and 1488:
• SC4-O005 SCRIBING METHOD OPTIMI
- Page 1489 and 1490:
• SC4-O006 PREPARATION OF HALIDE
- Page 1491 and 1492:
• SC4-O007 EFFECT OF RAPID THERMA
- Page 1493 and 1494:
Keywords: p-type, Nickel Oxide, Hol
- Page 1495 and 1496:
• SC4-O010 FIRST PRINCIPLES STUDY
- Page 1497 and 1498:
• SC4-O012 THE IMPORTANCE OF INSE
- Page 1499 and 1500:
• SC4-O014 Invited Talk CIGS THIN
- Page 1501 and 1502:
• SC4-O016 Invited Talk GaAs PHOT
- Page 1503 and 1504:
• SC4-O018 NANOWIRE DEVICE DESIGN
- Page 1505 and 1506:
• SC4-O020 SILICON SOLAR CELL WIT
- Page 1507 and 1508:
• SC4-O022 PROCESSING AND CHARACT
- Page 1509 and 1510:
• SC4-O024 BLACK NICKEL ELECTRODE
- Page 1511 and 1512:
• SC4-O025 EFFECTS OF Cd- AND AG-
- Page 1513 and 1514:
• SC4-P001 SYNTHESIS AND CHARACTE
- Page 1515 and 1516:
• SC4-P003 DIPPING AS ALTERNATIVE
- Page 1517 and 1518:
• SC4-P005 NOVEL METHOD TO DETERM
- Page 1519 and 1520:
• SC4-P007 SYNTHESIS OF ZnO THIN
- Page 1521 and 1522:
Acknowledgment: Authors would like
- Page 1523 and 1524:
NCD films grown in this work make t
- Page 1525 and 1526:
• SC4-P011 MOLYBDENUM SULFIDE NAN
- Page 1527 and 1528:
• SC4-P012 SOL-GEL SYNTHESIS OF Z
- Page 1529 and 1530:
Nacional de Nanotecnología” of C
- Page 1531 and 1532:
• SC4-P015 β-CuGaO2 AS A STRONG
- Page 1533 and 1534:
the number of carriers generated an
- Page 1535 and 1536:
References: [1] C. Li, M. Liu, et a
- Page 1537 and 1538:
References [1] H. Zhou et al. Scien
- Page 1539 and 1540:
• SC4-P020 UP-CONVERSION PHOTOLUM
- Page 1541 and 1542:
• SC4-P022 EVALUATION OF THE EFFE
- Page 1543 and 1544:
• SC4-P024 CdS THIN FILMS DEPOSIT
- Page 1545 and 1546:
Keywords: Schiff base, Transition m
- Page 1547 and 1548:
• SC4-P027 EFFECT OF THERMAL TREA
- Page 1549 and 1550:
• SC4-P029 ANALYSIS OF TCT PV ARR
- Page 1551 and 1552:
[1] J. Pilo, A. Trejo, E. Carvajal,
- Page 1553 and 1554:
• SC4-P032 CONSTRUCTION OF A SOLA
- Page 1555 and 1556:
• SC4-P033 SOLAR CELL EFFICIENCY
- Page 1557 and 1558:
• SC4-P034 EVALUATION OF HIGHLY E
- Page 1559 and 1560:
• SC4-P036 EFFECT OF AMMONIUM HYD
- Page 1561 and 1562:
• SC4-P037 ELECTRODEPOSITION OF S
- Page 1563 and 1564:
• SC4-P038 ENHANCED PHOTORESPONSE
- Page 1565 and 1566:
deposited and heated films are of 2
- Page 1567 and 1568:
• SC4-P041 DESIGN AND DEVELOPMENT
- Page 1569 and 1570:
• SC4-P043 STUDY OF TRIPHENYLAMIN
- Page 1571 and 1572:
• SC4-P045 GROWTH AND CHARACTERIZ
- Page 1573 and 1574:
• SC4-P047 ENHANCED LIGHT HARVEST
- Page 1575 and 1576:
• SC4-P048 LUMINISCENCE AND CONDU
- Page 1577 and 1578:
• SC4-P050 PHOTOVOLTAIC STUDY OF
- Page 1579 and 1580:
• SC4-P052 INFLUENCE OF MESOPOROU
- Page 1581 and 1582:
• SC4-P054 EFFECT OF GE IN KESTER
- Page 1583 and 1584:
• SC4-P056 CHARACTERIZATION OF N-
- Page 1585 and 1586:
polymeric matrix for the fabricatio
- Page 1587 and 1588:
Acknowledgment: University of Puert
- Page 1589 and 1590:
• SC4-P060 THE PURSUIT OF HIGH ST
- Page 1591 and 1592:
• SC4-P062 MAGNETRON-SPUTTERED SE
- Page 1593 and 1594:
• SC4-P064 EFFECTS OF SECONDARY P
- Page 1595 and 1596:
• SC4-P065 INSTRUMENTATION AND CO
- Page 1597 and 1598:
• SC4-P066 RE-DESIGN, CONSTRUCTIO
- Page 1599 and 1600:
• SC4-P067 BEHAVIOR OF TiO2 MESOP
- Page 1601 and 1602:
• SC4-P069 DESIGN, CONSTRUCTION A
- Page 1603 and 1604:
• SC4-P071 SYNTHESIS AND CHARACTE
- Page 1605 and 1606:
• SC4-P073 Blue CdTe surface obta
- Page 1607 and 1608:
• SC4-P075 DISCUSSION OF THIN FIL
- Page 1609 and 1610:
• SC4-P077 ZnS DEPOSITION BY THE
- Page 1611 and 1612:
• SC4-P079 PHOTOVOLTAIC DEVICES O
- Page 1613 and 1614:
• SC4-P081 ELECTRONIC STRUCTURE A
- Page 1615 and 1616:
• SC4-P082 STUDY OF THE SPECIFIC
- Page 1617 and 1618:
• SC4-P084 ELECTRICAL CHARACTERIZ
- Page 1619 and 1620:
Acknowledgment: Instituto para la I
- Page 1621 and 1622:
• SC4-P087 ALUMINUM, ALUMINA AND
- Page 1623 and 1624:
Keywords: BiFeO3, Optical propertie
- Page 1625 and 1626:
• SC4-P090 STUDY OF PROPERTIES OF
- Page 1627 and 1628:
• SC4-P092 COMPUTATIONAL STUDY OF
- Page 1629 and 1630:
• SC4-P094 THEORETICAL STUDY OF S
- Page 1631 and 1632:
• SC4-P096 LEAD-FREE INORGANIC Cs
- Page 1633 and 1634:
• SC4-P098 STUDY OF THE OPTOELECT
- Page 1635 and 1636:
Acknowledgment: I thank the Polytec
- Page 1637 and 1638:
Acknowledgment: The authors gratefu
- Page 1639 and 1640:
• SC4-P102 STUDY OF MORPHOLOGICAL
- Page 1641 and 1642:
Keywords: Photovoltaic wastes, Part
- Page 1643 and 1644:
XPS characterization of MoOx thin f
- Page 1645 and 1646:
Project P-18. We would like to ackn
- Page 1647 and 1648:
• SC4-P107 STUDY OF OPTICAL, STRU
- Page 1649 and 1650:
• SC4-P109 CDTE PHOTO-LUMINESCENT
- Page 1651 and 1652:
• SC4-P110 SIMULATION OF REAL c-G
- Page 1653 and 1654:
• SC4-P112 INVERTED STRUCTURE OF
- Page 1655 and 1656:
• SC4-P114 EFFECT OF THE NUMBER O
- Page 1657 and 1658:
• SC4-P115 SIMULATION AND MODELLI
- Page 1659 and 1660:
• SC4-P117 DEPOSITION, CHARACTERI
- Page 1661 and 1662:
• SC4-P119 TRANSITION METAL COMPL
- Page 1663 and 1664:
• SC4-P121 OPTIMIZATION OF CDS QU
- Page 1665 and 1666:
• SC4-P123 GROWTH AND DISORDER CH
- Page 1667 and 1668:
• SC4-P124 DETECTION OF ELECTROLU
- Page 1669 and 1670:
• SC4-P126 PIPERIDINE-SUBSTITUTED
- Page 1671 and 1672:
• SC4-P127 COMPARISON OF SOLAR RA
- Page 1673 and 1674:
ORAL SESSION
- Page 1675 and 1676:
• SC4-O002 Invited Talk NOVEL ORG
- Page 1677 and 1678:
• SC4-O003 SYNTHESIS OF REGIOREGU
- Page 1679 and 1680:
• SC4-O004 A HIGH OPEN CIRCUIT VO
- Page 1681 and 1682:
• SC4-O005 SCRIBING METHOD OPTIMI
- Page 1683 and 1684:
• SC4-O006 PREPARATION OF HALIDE
- Page 1685 and 1686:
• SC4-O007 EFFECT OF RAPID THERMA
- Page 1687 and 1688:
Keywords: p-type, Nickel Oxide, Hol
- Page 1689 and 1690:
• SC4-O010 FIRST PRINCIPLES STUDY
- Page 1691 and 1692:
• SC4-O012 THE IMPORTANCE OF INSE
- Page 1693 and 1694:
• SC4-O014 Invited Talk CIGS THIN
- Page 1695 and 1696:
• SC4-O016 Invited Talk GaAs PHOT
- Page 1697 and 1698:
• SC4-O018 NANOWIRE DEVICE DESIGN
- Page 1699 and 1700:
• SC4-O020 SILICON SOLAR CELL WIT
- Page 1701 and 1702:
• SC4-O022 PROCESSING AND CHARACT
- Page 1703 and 1704:
• SC4-O024 BLACK NICKEL ELECTRODE
- Page 1705 and 1706:
• SC4-O025 EFFECTS OF Cd- AND AG-
- Page 1707 and 1708:
• SC4-P001 SYNTHESIS AND CHARACTE
- Page 1709 and 1710:
• SC4-P003 DIPPING AS ALTERNATIVE
- Page 1711 and 1712:
• SC4-P005 NOVEL METHOD TO DETERM
- Page 1713 and 1714:
• SC4-P007 SYNTHESIS OF ZnO THIN
- Page 1715 and 1716:
Acknowledgment: Authors would like
- Page 1717 and 1718:
NCD films grown in this work make t
- Page 1719 and 1720:
• SC4-P011 MOLYBDENUM SULFIDE NAN
- Page 1721 and 1722:
• SC4-P012 SOL-GEL SYNTHESIS OF Z
- Page 1723 and 1724:
Nacional de Nanotecnología” of C
- Page 1725 and 1726:
• SC4-P015 β-CuGaO2 AS A STRONG
- Page 1727 and 1728:
the number of carriers generated an
- Page 1729 and 1730:
References: [1] C. Li, M. Liu, et a
- Page 1731 and 1732:
References [1] H. Zhou et al. Scien
- Page 1733 and 1734:
• SC4-P020 UP-CONVERSION PHOTOLUM
- Page 1735 and 1736:
• SC4-P022 EVALUATION OF THE EFFE
- Page 1737 and 1738:
• SC4-P024 CdS THIN FILMS DEPOSIT
- Page 1739 and 1740:
Keywords: Schiff base, Transition m
- Page 1741 and 1742:
• SC4-P027 EFFECT OF THERMAL TREA
- Page 1743 and 1744:
• SC4-P029 ANALYSIS OF TCT PV ARR
- Page 1745 and 1746:
[1] J. Pilo, A. Trejo, E. Carvajal,
- Page 1747 and 1748:
• SC4-P032 CONSTRUCTION OF A SOLA
- Page 1749 and 1750:
• SC4-P033 SOLAR CELL EFFICIENCY
- Page 1751 and 1752:
• SC4-P034 EVALUATION OF HIGHLY E
- Page 1753 and 1754:
• SC4-P036 EFFECT OF AMMONIUM HYD
- Page 1755 and 1756:
• SC4-P037 ELECTRODEPOSITION OF S
- Page 1757 and 1758:
• SC4-P038 ENHANCED PHOTORESPONSE
- Page 1759 and 1760:
deposited and heated films are of 2
- Page 1761 and 1762:
• SC4-P041 DESIGN AND DEVELOPMENT
- Page 1763 and 1764:
• SC4-P043 STUDY OF TRIPHENYLAMIN
- Page 1765 and 1766:
• SC4-P045 GROWTH AND CHARACTERIZ
- Page 1767 and 1768:
• SC4-P047 ENHANCED LIGHT HARVEST
- Page 1769 and 1770:
• SC4-P048 LUMINISCENCE AND CONDU
- Page 1771 and 1772:
• SC4-P050 PHOTOVOLTAIC STUDY OF
- Page 1773 and 1774:
• SC4-P052 INFLUENCE OF MESOPOROU
- Page 1775 and 1776:
• SC4-P054 EFFECT OF GE IN KESTER
- Page 1777 and 1778:
• SC4-P056 CHARACTERIZATION OF N-
- Page 1779 and 1780:
polymeric matrix for the fabricatio
- Page 1781 and 1782:
Acknowledgment: University of Puert
- Page 1783 and 1784:
• SC4-P060 THE PURSUIT OF HIGH ST
- Page 1785 and 1786:
• SC4-P062 MAGNETRON-SPUTTERED SE
- Page 1787 and 1788:
• SC4-P064 EFFECTS OF SECONDARY P
- Page 1789 and 1790:
• SC4-P065 INSTRUMENTATION AND CO
- Page 1791 and 1792:
• SC4-P066 RE-DESIGN, CONSTRUCTIO
- Page 1793 and 1794:
• SC4-P067 BEHAVIOR OF TiO2 MESOP
- Page 1795 and 1796:
• SC4-P069 DESIGN, CONSTRUCTION A
- Page 1797 and 1798:
• SC4-P071 SYNTHESIS AND CHARACTE
- Page 1799 and 1800:
• SC4-P073 Blue CdTe surface obta
- Page 1801 and 1802:
• SC4-P075 DISCUSSION OF THIN FIL
- Page 1803 and 1804:
• SC4-P077 ZnS DEPOSITION BY THE
- Page 1805 and 1806:
• SC4-P079 PHOTOVOLTAIC DEVICES O
- Page 1807 and 1808:
• SC4-P081 ELECTRONIC STRUCTURE A
- Page 1809 and 1810:
• SC4-P082 STUDY OF THE SPECIFIC
- Page 1811 and 1812:
• SC4-P084 ELECTRICAL CHARACTERIZ
- Page 1813 and 1814:
Acknowledgment: Instituto para la I
- Page 1815 and 1816:
• SC4-P087 ALUMINUM, ALUMINA AND
- Page 1817 and 1818:
Keywords: BiFeO3, Optical propertie
- Page 1819 and 1820:
• SC4-P090 STUDY OF PROPERTIES OF
- Page 1821 and 1822:
• SC4-P092 COMPUTATIONAL STUDY OF
- Page 1823 and 1824:
• SC4-P094 THEORETICAL STUDY OF S
- Page 1825 and 1826:
• SC4-P096 LEAD-FREE INORGANIC Cs
- Page 1827 and 1828:
• SC4-P098 STUDY OF THE OPTOELECT
- Page 1829 and 1830:
Acknowledgment: I thank the Polytec
- Page 1831 and 1832:
Acknowledgment: The authors gratefu
- Page 1833 and 1834:
• SC4-P102 STUDY OF MORPHOLOGICAL
- Page 1835 and 1836:
Keywords: Photovoltaic wastes, Part
- Page 1837 and 1838:
XPS characterization of MoOx thin f
- Page 1839 and 1840:
Project P-18. We would like to ackn
- Page 1841 and 1842:
• SC4-P107 STUDY OF OPTICAL, STRU
- Page 1843 and 1844:
• SC4-P109 CDTE PHOTO-LUMINESCENT
- Page 1845 and 1846:
• SC4-P110 SIMULATION OF REAL c-G
- Page 1847 and 1848:
• SC4-P112 INVERTED STRUCTURE OF
- Page 1849 and 1850:
• SC4-P114 EFFECT OF THE NUMBER O
- Page 1851 and 1852:
• SC4-P115 SIMULATION AND MODELLI
- Page 1853 and 1854:
• SC4-P117 DEPOSITION, CHARACTERI
- Page 1855 and 1856:
• SC4-P119 TRANSITION METAL COMPL
- Page 1857 and 1858:
• SC4-P121 OPTIMIZATION OF CDS QU
- Page 1859 and 1860:
• SC4-P123 GROWTH AND DISORDER CH
- Page 1861 and 1862:
• SC4-P124 DETECTION OF ELECTROLU
- Page 1863 and 1864:
• SC4-P126 PIPERIDINE-SUBSTITUTED
- Page 1865 and 1866:
• SC4-P127 COMPARISON OF SOLAR RA
- Page 1867 and 1868:
ORAL SESSION
- Page 1869 and 1870:
Hellmann, and A. Kittel, Nature Com
- Page 1871 and 1872:
• SC6-O003 MEASUREMENT AND MODELI
- Page 1873 and 1874:
• SC6-O005 Invited Talk OPENING A
- Page 1875 and 1876:
• SC6-O007 Invited Talk ENERGY TR
- Page 1877 and 1878:
[7] E. M. Lifshitz and L. P. Pitaev
- Page 1879 and 1880:
• SC6-O010 Invited Talk TOPOLOGIC
- Page 1881 and 1882:
• SC6-O012 TOWARDS AN ALL-ELECTRI
- Page 1883 and 1884:
• SC6-O013 Invited Talk THERMAL T
- Page 1885 and 1886:
• SC6-O014 Invited Talk THERMAL R
- Page 1887 and 1888:
• SC6-O015 Invited Talk SURFACE-M
- Page 1889 and 1890:
• SC6-O017 Invited Talk FREQUENCY
- Page 1891 and 1892:
• SC6-O018 THERMAL RECTIFICATION
- Page 1893 and 1894:
POSTER SESSION
- Page 1895 and 1896:
• SC6-P002 FREQUENCY DOMAIN PHOTO
- Page 1897 and 1898:
• SC6-P004 EFFECTIVE MEDIUM THEOR
- Page 1899 and 1900:
theoretically verify the experiment
- Page 1901 and 1902:
• SC6-P007 THERMAL CHARACTERIZATI
- Page 1903 and 1904:
Symposium C7 METAL-ORGANIC FRAMEWOR
- Page 1905 and 1906:
• SC7-O001 Invited Talk OVERSOLUB
- Page 1907 and 1908:
• SC7-O003 FACILE ZIF-67@meso-Al2
- Page 1909 and 1910:
A.; Kapteijn, F.; Gascon, J. ChemCa
- Page 1911 and 1912:
• SC7-O006 Invited Talk CATALYSTS
- Page 1913 and 1914:
• SC7-O007 ACTIVE SITE ENGINEERIN
- Page 1915 and 1916:
• SC7-O008 Invited Talk ADDING SO
- Page 1917 and 1918:
• SC7-O009 STRUCTURE OF A NEW COP
- Page 1919 and 1920:
4. S. Van Cleuvenbergen, et al. Nat
- Page 1921 and 1922:
• SC7-O012 NOVEL Zn/Co ZEOLITIC I
- Page 1923 and 1924:
• SC7-O014 Invited Talk METAL-ORG
- Page 1925 and 1926:
The authors would like to acknowled
- Page 1927 and 1928:
• SC7-O017 MOF@GO COMPOSITES FOR
- Page 1929 and 1930:
• SC7-O019 Invited Talk DRUG VECT
- Page 1931 and 1932:
• SC7-O021 Invited Talk MOF-BASED
- Page 1933 and 1934:
POSTER SESSION
- Page 1935 and 1936:
• SC7-P002 SYNTHESIS AND CHARACTE
- Page 1937 and 1938:
• SC7-P003 EFFECT OF THE SYNTHESI
- Page 1939 and 1940:
• SC7-P005 ULTRASONIC SPRAY DEPOS
- Page 1941 and 1942:
2014-16823) and the People Programm
- Page 1943 and 1944:
• SC7-P008 POROUS CARBON DERIVED
- Page 1945 and 1946:
• SC7-P009 STUDY OF SILVER IONS R
- Page 1947 and 1948:
• SC7-P011 TUNING THE ADSORPTION
- Page 1949 and 1950:
• SC7-P013 EVALUATION OF METAL OR
- Page 1951 and 1952:
• SC7-P015 HYDROGEN ISOTOPE SEPAR
- Page 1953 and 1954:
• SC7-P017 SEPARATION OF H2-CO2 A
- Page 1955 and 1956:
Excellent reusability was obtained,
- Page 1957 and 1958:
ORAL SESSION
- Page 1959 and 1960:
36-45. (4) Fjermestad, T.; Li, W.;
- Page 1961 and 1962:
• SD1-O003 OVERCOMING SITE HETERO
- Page 1963 and 1964:
• SD1-O004 Invited Talk RATIONAL
- Page 1965 and 1966:
• SD1-O006 Invited Talk A CHEMICA
- Page 1967 and 1968:
• SD1-O008 Invited Talk MANY BODY
- Page 1969 and 1970:
• SD1-O010 Invited Talk ADVANCES
- Page 1971 and 1972:
• SD1-O012 Invited Talk THE SCAN
- Page 1973 and 1974:
• SD1-O013 SIMPLIFICATION OF META
- Page 1975 and 1976:
• SD1-O015 Invited Talk MOLECULAR
- Page 1977 and 1978:
M.R.B. and F.B.Z. acknowledge suppo
- Page 1979 and 1980:
Acknowledgment: Supported by NSF aw
- Page 1981 and 1982:
• SD1-O019 CONFIGURATIONAL ENERGY
- Page 1983 and 1984:
Acknowledgment: J.M.C. thanks the 1
- Page 1985 and 1986:
emarkably accurate structures and s
- Page 1987 and 1988:
shape of the surface polymorph, whi
- Page 1989 and 1990:
• SD1-O024 HOW TO CHOOSE THE DFT
- Page 1991 and 1992:
• SD1-O025 GLOBAL OPTIMIZATION OF
- Page 1993 and 1994:
• SD1-O026 AMORPHOUS ZnO: STRUCTU
- Page 1995 and 1996:
• SD1-O027 Invited Talk RELIABLE
- Page 1997 and 1998:
• SD1-O029 Invited Talk MAGNETISM
- Page 1999 and 2000:
• SD1-O031 FIRST-PRINCIPLES CALCU
- Page 2001 and 2002:
• SD1-O033 FERROMAGNETISM VS ANTI
- Page 2003 and 2004:
• SD1-O034 Invited Talk CLATHRATE
- Page 2005 and 2006:
• SD1-O035 Invited Talk POLYMORPH
- Page 2007 and 2008:
3. E. C. Dos Santos, et al.; J. Phy
- Page 2009 and 2010:
• SD1-O038 THEORETICAL STUDY OF T
- Page 2011 and 2012:
• SD1-O039 UNDERSTANDING MECHANOC
- Page 2013 and 2014:
Acknowledgment: We acknowledge fina
- Page 2015 and 2016:
• [3] K. Shceunemann, H.K. Mülle
- Page 2017 and 2018:
• SD1-O043 ACCELERATED DISCOVERY
- Page 2019 and 2020:
• SD1-O045 Invited Talk MODELING
- Page 2021 and 2022:
POSTER SESSION
- Page 2023 and 2024:
• SD1-P002 ON THE QUANTUM CHEMIST
- Page 2025 and 2026:
Acknowledgment: The computational r
- Page 2027 and 2028:
• SD1-P005 THEORETICAL STUDY OF S
- Page 2029 and 2030:
• SD1-P007 FIRST PRINCIPLES PREDI
- Page 2031 and 2032: • SD1-P008 INCREASE OF THE RESOLU
- Page 2033 and 2034: • SD1-P010 IMPROVED ATOMIZATION E
- Page 2035 and 2036: [3] Hosseinian, A., Bekhradnia, A.,
- Page 2037 and 2038: • SD1-P013 EXPLOITING THE SIMILAR
- Page 2039 and 2040: • SD1-P015 TUNNELING I-V CHARACTE
- Page 2041 and 2042: • SD1-P017 EFFECT OF INCREASING T
- Page 2043 and 2044: • SD1-P019 HEXATIC AND TETRATIC P
- Page 2045 and 2046: Symposium D2 MACHINE LEARNING AND D
- Page 2047 and 2048: • SD2-O001 BRIDGING THE ELECTRONI
- Page 2049 and 2050: • SD2-O003 Invited Talk MASSIVE A
- Page 2051 and 2052: Science User Facility supported by
- Page 2053 and 2054: • SD2-O006 FILLING THE GAPS IN A
- Page 2055 and 2056: • SD2-O008 Invited Talk INTEGRATE
- Page 2057 and 2058: • SD2-O010 DEEP LEARNING FOR SEGM
- Page 2059 and 2060: • SD2-O011 HIGH-THROUGHPUT GENERA
- Page 2061 and 2062: • SD2-O013 Invited Talk MATERIALS
- Page 2063 and 2064: The details will be discussed in my
- Page 2065 and 2066: Alamos National Security, LLC, thro
- Page 2067 and 2068: • SD2-O017 Invited Talk MACHINE (
- Page 2069 and 2070: Autónomo Fondo Nacional de Financi
- Page 2071 and 2072: • SD2-O020 Invited Talk MACHINE L
- Page 2073 and 2074: • SD2-O022 Invited Talk HOLISTIC
- Page 2075 and 2076: • SD2-O024 Invited Talk GUIDING M
- Page 2077 and 2078: • SD2-O026 SIMILARITY OF ELECTRON
- Page 2079 and 2080: • SD2-O028 Invited Talk DATA SCIE
- Page 2081: • SD2-O030 Invited Talk DESIGN OF
- Page 2085 and 2086: applied this approach to binary met
- Page 2087 and 2088: • SD2-O035 INTEGRATING MACHINE LE
- Page 2089 and 2090: • SD2-O036 MACHINE LEARNING APPRO
- Page 2091 and 2092: • SD2-O037 PREDICTING THE DISSOLU
- Page 2093 and 2094: • SD2-P001 PHYSICAL ASPECTS AND M
- Page 2095 and 2096: • SD2-P003 SELF-ASSEMBLY OF BLUE
- Page 2097 and 2098: • SD2-P005 3D MODELLING OF THE TR
- Page 2099 and 2100: • SD2-P007 MODELLING OF PHASE BEH
- Page 2101 and 2102: • SD2-P008 STUDY OF THE REACTIVIT
- Page 2103 and 2104: • SD2-P010 DEVELOPMENT OF A MONIT
- Page 2105 and 2106: • SD2-P011 COMPARISON OF THE EFFI
- Page 2107 and 2108: • SD2-P013 EFFECTS OF FINITE SIZE
- Page 2109 and 2110: ORAL SESSION
- Page 2111 and 2112: of analysis of variants include com
- Page 2113 and 2114: • SD3-O003 A COMPARISON OF ELECTR
- Page 2115 and 2116: • SD3-O005 Invited Talk STATISTIC
- Page 2117 and 2118: • SD3-O007 CONTROLLING THE MORPHO
- Page 2119 and 2120: problems due to the insufficient du
- Page 2121 and 2122: io-mineralized tissues, polished wi
- Page 2123 and 2124: • SD3-O011 CRYSTALLOGRAPHIC TEXTU
- Page 2125 and 2126: • SD3-O013 MICROSTRUCTURAL EVOLUT
- Page 2127 and 2128: • SD3-O014 EFFECT OF THE BORIDING
- Page 2129 and 2130: • SD3-O016 Invited Talk EBSD ANAL
- Page 2131 and 2132: • SD3-O018 EVOLUTION OF ROLLING A
- Page 2133 and 2134:
• SD3-O019 QUANTITATIVE DETERMINA
- Page 2135 and 2136:
• SD3-O021 Invited Talk LAYER STA
- Page 2137 and 2138:
POSTER SESSION
- Page 2139 and 2140:
• SD3-P002 EFFECT OF INITIAL TEXT
- Page 2141 and 2142:
• SD3-P004 EFFECT OF TEXTURE ON G
- Page 2143 and 2144:
Symposium D4 PROBING NANOMATERIALS
- Page 2145 and 2146:
• SD4-O001 Invited Talk NOVEL SOL
- Page 2147 and 2148:
• SD4-O003 Invited Talk ELECTRON
- Page 2149 and 2150:
plasmonic properties of graphene in
- Page 2151 and 2152:
conservation of AlGaN/GaN interface
- Page 2153 and 2154:
References: [1] S. Helveg, J. Catal
- Page 2155 and 2156:
• SD4-O008 DIRECT ATOMIC-SCALE OB
- Page 2157 and 2158:
• SD4-O009 ELECTRON MICROSCOPY OF
- Page 2159 and 2160:
• SD4-O010 Invited Talk PROBING S
- Page 2161 and 2162:
• SD4-O012 CARBON BASED NANOHYBRI
- Page 2163 and 2164:
• SD4-O013 Invited Talk MONOCHROM
- Page 2165 and 2166:
• SD4-O014 Invited Talk UNDERSTAN
- Page 2167 and 2168:
In order to avoid false symmetry in
- Page 2169 and 2170:
• SD4-O017 Invited Talk GRAPHENE
- Page 2171 and 2172:
• SD4-O018 Invited Talk NEW AND N
- Page 2173 and 2174:
[1] K.S. Novoselov et al., Science
- Page 2175 and 2176:
• SD4-O021 FERROELECTRIC MODULATE
- Page 2177 and 2178:
• SD4-O023 Invited Talk ADVANCES
- Page 2179 and 2180:
POSTER SESSION
- Page 2181 and 2182:
• SD4-P002 DOPED TiO2 MOSOPOROUS
- Page 2183 and 2184:
Acknowledgment: The authors want to
- Page 2185 and 2186:
Keywords: Multifunctional surfaces,
- Page 2187 and 2188:
Acknowledgment: The financial suppo
- Page 2189 and 2190:
assimilated, and its bioactive pote
- Page 2191 and 2192:
ORAL SESSION
- Page 2193 and 2194:
• SD5-O002 Invited Talk ATOMIC IN
- Page 2195 and 2196:
• SD5-O003 IN-SITU ATOMIC-SCALE O
- Page 2197 and 2198:
• SD5-O004 IN-SITU STUDY OF THE D
- Page 2199 and 2200:
• SD5-O006 Invited Talk IN SITU S
- Page 2201 and 2202:
further implemented in the quantita
- Page 2203 and 2204:
dynamics of single Barium ions in t
- Page 2205 and 2206:
Keywords: In Situ TEM, Battery Mate
- Page 2207 and 2208:
• SD5-O011 INVESTIGATION OF LIQUI
- Page 2209 and 2210:
• SD5-O012 DYNAMIC SURFACE PROCES
- Page 2211 and 2212:
• SD5-O013 Invited Talk NEW DESIG
- Page 2213 and 2214:
Keywords: EM, Deep Learning, AI Pre
- Page 2215 and 2216:
• SD5-O016 Invited Talk QUANTITAT
- Page 2217 and 2218:
• SD5-O017 Invited Talk DESIGN OF
- Page 2219 and 2220:
• SD5-O019 Invited Talk NANOPARTI
- Page 2221 and 2222:
• SD5-O021 Invited Talk IN SITU T
- Page 2223 and 2224:
• SD5-O023 A NEW FRAMEWORK FOR IN
- Page 2225 and 2226:
POSTER SESSION
- Page 2227 and 2228:
• SD5-P002 MICROSTRUCTURAL EVOLUT
- Page 2229 and 2230:
• SD5-P004 EFFECT OF IN-SITU HEAT
- Page 2231 and 2232:
ORAL SESSION
- Page 2233 and 2234:
• SD6-O002 Invited Talk CERAMIC D
- Page 2235 and 2236:
• SD6-O004 4D ULTRA-FAST X-RAY IM
- Page 2237 and 2238:
• SD6-O006 Invited Talk MICROSTRU
- Page 2239 and 2240:
• SD6-O007 Invited Talk CEMENT HY
- Page 2241 and 2242:
• SD6-O008 Invited Talk CHARACTER
- Page 2243 and 2244:
• SD6-O009 TREATMENT OF BACKGROUN
- Page 2245 and 2246:
• SD6-O011 STRUCTURAL CHARACTERIZ
- Page 2247 and 2248:
Acknowledgment: The contributions o
- Page 2249 and 2250:
• SD6-O014 Invited Talk X-RAY DIF
- Page 2251 and 2252:
• SD6-O016 HOMOGENEITY OF CO-MILL
- Page 2253 and 2254:
• SD6-P001 HPLC CHARACTERIZATION
- Page 2255 and 2256:
• SD6-P003 TEXTURE INFLUENCE ON T
- Page 2257 and 2258:
• SD6-P004 STUDYING THE STRUCTURE
- Page 2259 and 2260:
• SD6-P006 STRUCTURAL XRD and SEM
- Page 2261 and 2262:
• SD6-P008 THERMODYNAMICS OF THE
- Page 2263 and 2264:
• SD6-P009 STRUCTURAL AND OPTICAL
- Page 2265 and 2266:
• SD6-P011 STRUCTURAL EVOLUTION O
- Page 2267 and 2268:
• SD6-P013 CHARACTERIZATION OF AR
- Page 2269 and 2270:
• SD6-P015 EVOLUTION OF MORPHOLOG
- Page 2271 and 2272:
• SD6-P016 ANALYSIS OF THE MOLARI
- Page 2273 and 2274:
• SD6-P018 MAGNETIC STUDY ON A NA
- Page 2275 and 2276:
• SD6-P019 SYNTHESIS AND CHARACTE
- Page 2277 and 2278:
ORAL SESSION
- Page 2279 and 2280:
Pablo López Arriaga, IF-UNAM, for
- Page 2281 and 2282:
promotes an easier nucleation of th
- Page 2283 and 2284:
• SD7-O004 RELATIONSHIP BETWEEN H
- Page 2285 and 2286:
• SD7-O006 TRANSFORMATION OF NBC
- Page 2287 and 2288:
• SD7-O008 Invited Talk CRACK GRO
- Page 2289 and 2290:
• SD7-O010 EFFECT OF MAGNESIUM ON
- Page 2291 and 2292:
• SD7-O012 SYNTHESIS, CHARACTERIZ
- Page 2293 and 2294:
Acknowledgment: This work was suppo
- Page 2295 and 2296:
• SD7-O015 ALUMINUM ALLOY 7075-T6
- Page 2297 and 2298:
• SD7-O017 CHARACTERIZATION OF NA
- Page 2299 and 2300:
• SD7-O019 FABRICATION AND CHARAC
- Page 2301 and 2302:
to acknowledge the Laboratorio Avan
- Page 2303 and 2304:
References: [1] M.S. Safronova, W.R
- Page 2305 and 2306:
• SD7-O023 CHARACTERIZATION OF ZI
- Page 2307 and 2308:
• SD7-P001 MECHANICAL BEHAVIOR AT
- Page 2309 and 2310:
• SD7-P003 ANALYSIS OF 3D PLANAR
- Page 2311 and 2312:
Acknowledgment: The authors acknowl
- Page 2313 and 2314:
• SD7-P006 BIOSYNTHESIS OF IRON O
- Page 2315 and 2316:
• SD7-P007 EFFECT OF WC ON MECHAN
- Page 2317 and 2318:
• SD7-P009 SYNTHESIS AND CHARACTE
- Page 2319 and 2320:
• SD7-P011 EFFECT OF CHROMIUM AS
- Page 2321 and 2322:
Acknowledgment: This research was s
- Page 2323 and 2324:
Rosas Huerta want to thanks to CONA
- Page 2325 and 2326:
• SD7-P015 INFLUENCE OF AlN PHASE
- Page 2327 and 2328:
• SD7-P017 ANALYSIS OF THE STRUCT
- Page 2329 and 2330:
• SD7-P018 GRAIN REFINEMENT OF Al
- Page 2331 and 2332:
Acknowledgment: The technical assis
- Page 2333 and 2334:
Acknowledgment: The authors would l
- Page 2335 and 2336:
• SD7-P022 CHARACTERISATION OF AL
- Page 2337 and 2338:
• SD7-P024 STRUCTURAL STUDY OF HY
- Page 2339 and 2340:
• SD7-P026 FATIGUE STUDY AND EFFE
- Page 2341 and 2342:
• SD7-P027 EFFECTS OF GRAPHITE IN
- Page 2343 and 2344:
• SD7-P029 DEVELOPMENT OF A PROTO
- Page 2345 and 2346:
• SD7-P031 CHARACTERIZATION OF A
- Page 2347 and 2348:
• SD7-P033 SYNTHESIS, STRUCTURE A
- Page 2349 and 2350:
• SD7-P034 INFLUENCE OF THE COOLI
- Page 2351 and 2352:
• SD7-P036 MICROSTRUCTURAL EVALUA
- Page 2353 and 2354:
• SD7-P038 PRELIMINARY PROOFS OF
- Page 2355 and 2356:
References [1]. E. Salinas, E. Cere
- Page 2357 and 2358:
Urban Mining and Electrochemistry:
- Page 2359 and 2360:
• SD7-P042 INFLUENCE OF CARBURIZI
- Page 2361 and 2362:
• SD7-P044 MICROHARDNESS AND MICR
- Page 2363 and 2364:
• SD7-P046 CHARACTERIZATION OF BE
- Page 2365 and 2366:
• SD7-P047 CALCULATION OF GIBBS F
- Page 2367 and 2368:
• SD7-P049 PHOTOLUMINISCENT PROPE
- Page 2369 and 2370:
• SD7-P051 SINTESYS AND CHARACTER
- Page 2371 and 2372:
• SD7-P053 MANUFACTURING AND CHAR
- Page 2373 and 2374:
Acknowledgment: Authors acknowledge
- Page 2375 and 2376:
• SD7-P056 NON-DESTRUCTIVE SPATIA
- Page 2377 and 2378:
Acknowledgment: I.Betancourt acknow
- Page 2379 and 2380:
• SD7-P059 PHYSICAL PROPERTIES OF
- Page 2381 and 2382:
• SD7-P061 X-RAY RECIPROCAL SPACE
- Page 2383 and 2384:
• SD7-P063 SYNTHESIS AND CHARACTE
- Page 2385 and 2386:
• SD7-P065 CELLULOSE NANOCRYSTALS
- Page 2387 and 2388:
Symposium E1 STRUCTURAL LIGHTWEIGHT
- Page 2389 and 2390:
• SE1-O001 DENDRITIC AND NON-DEND
- Page 2391 and 2392:
characteristic cooling rates, which
- Page 2393 and 2394:
• SE1-O004 EFFECT OF CHEMICAL MOD
- Page 2395 and 2396:
• SE1-O006 Invited Talk HIGH-STRE
- Page 2397 and 2398:
• SE1-O008 EFFECT OF GADOLINIUM A
- Page 2399 and 2400:
• SE1-O010 Invited Talk UNDERSTAN
- Page 2401 and 2402:
• SE1-O012 Invited Talk PLASTIC D
- Page 2403 and 2404:
• SE1-O014 Invited Talk THE EFFEC
- Page 2405 and 2406:
• SE1-O016 CORROSION BEHAVIOR OF
- Page 2407 and 2408:
• SE1-O018 MECHANICAL BEHAVIOR AN
- Page 2409 and 2410:
• SE1-O020 DERIVATION OF THE FORM
- Page 2411 and 2412:
• SE1-P001 IMPLEMENTATION OF A PR
- Page 2413 and 2414:
characteristics as number density,
- Page 2415 and 2416:
Acknowledgment: This research was s
- Page 2417 and 2418:
• SE1-P005 STUDY OF THE PROCESS P
- Page 2419 and 2420:
• SE1-P006 HOT PRESSING AND PRESS
- Page 2421 and 2422:
• SE1-P008 EFFECT OF RE ON MICROS
- Page 2423 and 2424:
• SE1-P010 TEXTURE DEVELOPMENT DU
- Page 2425 and 2426:
[5] E. A. Albistur Goñil, J Pérez
- Page 2427 and 2428:
to the AZ80 also had a positive eff
- Page 2429 and 2430:
• SE1-P014 DETERMINATION OF THE F
- Page 2431 and 2432:
ORAL SESSION
- Page 2433 and 2434:
• SE2-O002 FINITE ELEMENT SIMULAT
- Page 2435 and 2436:
• SE2-O004 ANALYTICAL, COMPUTATIO
- Page 2437 and 2438:
• SE2-O005 Invited Talk SIMULATIO
- Page 2439 and 2440:
• SE2-O007 THERMAL AND MECHANICAL
- Page 2441 and 2442:
• SE2-O008 NON-LINEAR DEFORMATION
- Page 2443 and 2444:
• SE2-O010 STRUCTURAL AND MECHANI
- Page 2445 and 2446:
• SE2-O012 EFFECT OF THE FRACTALI
- Page 2447 and 2448:
• SE2-O014 BORONIZED LAYERS ON TH
- Page 2449 and 2450:
López-Cuevas , F.C. Robles-Hernand
- Page 2451 and 2452:
The Raman studies on the wear track
- Page 2453 and 2454:
• SE2-O018 INTERNAL CURING IN HIH
- Page 2455 and 2456:
• SE2-O020 STUDY OF THE BEHAVIOR
- Page 2457 and 2458:
cement mixtures by increasing the d
- Page 2459 and 2460:
Acknowledgment: We thank to the Uni
- Page 2461 and 2462:
• SE2-O024 LABORATORY INVESTIGATI
- Page 2463 and 2464:
• SE2-O026 IMPROVEMENT IN THE MEC
- Page 2465 and 2466:
• SE2-O027 PARAMETERS OF DURABILI
- Page 2467 and 2468:
3. P. A. Márquez Aguilar, M.Vlasov
- Page 2469 and 2470:
This study was supported by CONACYT
- Page 2471 and 2472:
• SE2-O031 RESISTANCE TO WEAR AT
- Page 2473 and 2474:
Acknowledgment: This project was su
- Page 2475 and 2476:
with a pore size >100μm, which is
- Page 2477 and 2478:
molar ratio and the thickness of th
- Page 2479 and 2480:
• SE2-O036 HILLOCK FORMATION ON N
- Page 2481 and 2482:
Acknowledgment: The authors thankfu
- Page 2483 and 2484:
• SE2-O039 MICROSTRUCTURAL EVOLUT
- Page 2485 and 2486:
• SE2-O040 Invited Talk LASER CLA
- Page 2487 and 2488:
Keywords: Metal matrix composites,
- Page 2489 and 2490:
• SE2-O043 A NOVEL EXPERIMENTAL T
- Page 2491 and 2492:
thank support from PICT-2014-0696 (
- Page 2493 and 2494:
S/cm respectively. Vickers microhar
- Page 2495 and 2496:
• SE2-O047 Invited Talk MAGNETORH
- Page 2497 and 2498:
• SE2-O049 SCALABLE FABRICATION O
- Page 2499 and 2500:
• SE2-O050 MECHANICAL PROPERTIES
- Page 2501 and 2502:
Acknowledgment: We acknowledge the
- Page 2503 and 2504:
• SE2-O053 INFLUENCE OF ELECTRODE
- Page 2505 and 2506:
POSTER SESSION
- Page 2507 and 2508:
• SE2-P002 FRACTURE FAILURE ASSES
- Page 2509 and 2510:
• SE2-P004 EFFECT OF EDGE DISLOCA
- Page 2511 and 2512:
• SE2-P006 DIGITAL SIGNAL ANALYSI
- Page 2513 and 2514:
• SE2-P008 STUDY OF THE HYDRATION
- Page 2515 and 2516:
• SE2-P009 EFFECT OF CARBON NANOT
- Page 2517 and 2518:
• SE2-P011 NANOINDENTATION STUDY
- Page 2519 and 2520:
• SE2-P013 SiO2 AND Al2O3 NANOPAR
- Page 2521 and 2522:
• SE2-P014 STRUCTURE AND THERMO-M
- Page 2523 and 2524:
• SE2-P015 FABRICATION AND CHARAC
- Page 2525 and 2526:
• SE2-P017 ON THE WAY FOR A SEMIA
- Page 2527 and 2528:
• SE2-P018 VALIDATION OF A COMPUT
- Page 2529 and 2530:
• SE2-P020 UNUSUAL ELASTIC DEFORM
- Page 2531 and 2532:
Process. Technol., Vol. 231, 2016.
- Page 2533 and 2534:
• SE2-P023 HYDROPHILIC PROPERTIES
- Page 2535 and 2536:
• SE2-P025 PREPARATION AND CHARAC
- Page 2537 and 2538:
• SE2-P027 STUDY OF CORROSION RES
- Page 2539 and 2540:
Control: Towards Innovative Develop
- Page 2541 and 2542:
• SE2-P030 INTERNAL CURING IN HIH
- Page 2543 and 2544:
• SE2-P032 REINFORCEMENT OF ALUMI
- Page 2545 and 2546:
• SE2-P033 STUDY OF THE MECHANISM
- Page 2547 and 2548:
• SE2-P035 ASPHALT PAVEMENT WITH
- Page 2549 and 2550:
• SE2-P037 DESIGN, SIMULATION AND
- Page 2551 and 2552:
• SE2-P039 MECHANICAL ACTIVATION
- Page 2553 and 2554:
• SE2-P041 ANALYSIS OF THE PROGRE
- Page 2555 and 2556:
• SE2-P043 COMPARISON OF THE MECH
- Page 2557 and 2558:
• SE2-P045 METAL OXIDES ADDITION
- Page 2559 and 2560:
• SE2-P046 SYNTHESIS AND THERMOLU
- Page 2561 and 2562:
• SE2-P048 MANUFACTURING OF NiCoC
- Page 2563 and 2564:
Acknowledgment: Faculty of mechanic
- Page 2565 and 2566:
• SE2-P051 ELECTROCHEMICAL EVALUA
- Page 2567 and 2568:
• SE2-P052 ORGANIC ADDITIVE FLUID
- Page 2569 and 2570:
• SE2-P054 CEMENT MORTARS OF CEME
- Page 2571 and 2572:
• SE2-P056 EVALUATION OF GOVERNME
- Page 2573 and 2574:
• SE2-P057 PARTIAL SUBSTITUTION O
- Page 2575 and 2576:
• SE2-P058 ANALYSIS OF COMPACTNES
- Page 2577 and 2578:
• SE2-P059 POZZOLANIC ACTIVITY OF
- Page 2579 and 2580:
• SE2-P060 EVALUATION OF THE BEHA
- Page 2581 and 2582:
• SE2-P062 PROPERTIES MATHEMATICA
- Page 2583 and 2584:
• SE2-P064 TENSILE STRENGTH OF NO
- Page 2585 and 2586:
• SE2-P066 STRUCTURAL AND OPTICAL
- Page 2587 and 2588:
• SE2-P068 BIOACTIVE COATING ON A
- Page 2589 and 2590:
• SE2-P070 EFFECT OF CITRIC ACID
- Page 2591 and 2592:
ORAL SESSION
- Page 2593 and 2594:
• SE3-O002 OXIDATION OF PYROLYTIC
- Page 2595 and 2596:
• SE3-O004 INFLUENCE OF THE ENTHA
- Page 2597 and 2598:
• SE3-O006 MeV PROTON IRRADIATION
- Page 2599 and 2600:
• SE3-O008 DOSIMETRIC CHARACTERIZ
- Page 2601 and 2602:
• SE3-O010 Invited Talk SPIN-ORBI
- Page 2603 and 2604:
• SE3-O012 GLOVE-BOX VERSION OF A
- Page 2605 and 2606:
• SE3-O014 Ag NANOPARTICLES EFFEC
- Page 2607 and 2608:
Acknowledgment: Thanks DGAPA-UNAM f
- Page 2609 and 2610:
• SE3-O017 LOWER DETECTABLE DOSE
- Page 2611 and 2612:
• SE3-P001 STUDY OF LITHIUM BORAT
- Page 2613 and 2614:
• SE3-P003 INCORPORATION OF QUANT
- Page 2615 and 2616:
• SE3-P005 HYDROQUINONE EFFECT ON
- Page 2617 and 2618:
• SE3-P007 THERMOLUMINESCENCE PRO
- Page 2619 and 2620:
• SE3-P009 SYNTHESIS OF TiO2:Eu P
- Page 2621 and 2622:
• SE3-P011 SYNTHESIS, CHARACTERIZ
- Page 2623 and 2624:
• SE3-P013 THERMOLUMINESCENCE OF
- Page 2625 and 2626:
• SE3-P015 EFFECT OF Dy DOPING ON
- Page 2627 and 2628:
• SE3-P017 YALO3:CARBON DOTS SYNT
- Page 2629 and 2630:
• SE3-P019 IMPACT OF AGING DEGRAD
- Page 2631 and 2632:
ORAL SESSION
- Page 2633 and 2634:
Acknowledgment: The authors thank t
- Page 2635 and 2636:
• SE4-O003 EFFECT OF HEAT TREATME
- Page 2637 and 2638:
• SE4-O005 MAIN FACTORS AFFECTING
- Page 2639 and 2640:
• SE4-O007 EFFECT OF A NON-CONVEN
- Page 2641 and 2642:
passive film, which redounds to an
- Page 2643 and 2644:
• SE4-O010 NONLINEAR DYNAMIC STUD
- Page 2645 and 2646:
• SE4-O012 LOW POWER - HI PROPAGA
- Page 2647 and 2648:
• SE4-O014 EVALUATION OF CORROSIO
- Page 2649 and 2650:
• SE4-O016 ISOTHERMAL AND CYCLIC
- Page 2651 and 2652:
• SE4-O017 STUDY OF CORROSION OF
- Page 2653 and 2654:
• SE4-O019 HIGH TEMPERATURE OXIDA
- Page 2655 and 2656:
• SE4-O021 COMPARATIVE STUDY OF T
- Page 2657 and 2658:
• SE4-O022 ELECTROCHEMICAL CHARAC
- Page 2659 and 2660:
• SE4-O024 HOT CORROSION OF 7YSZ
- Page 2661 and 2662:
• SE4-O026 MICROSTRUCTURAL EVOLUT
- Page 2663 and 2664:
• SE4-O027 FABRICATION AND MICROS
- Page 2665 and 2666:
• SE4-O029 ELECTROCHEMICAL EVALUA
- Page 2667 and 2668:
• SE4-O031 ELECTROCHEMICAL NOISE
- Page 2669 and 2670:
• SE4-O033 STRENGTH AND CORROSION
- Page 2671 and 2672:
• SE4-O035 EFFECT OF CORROSION IN
- Page 2673 and 2674:
• SE4-P001 CORROSION BEHAVIOR OF
- Page 2675 and 2676:
• SE4-P003 PREPARATION AND CHARAC
- Page 2677 and 2678:
• SE4-P005 EFFECT OF ASPHALTENES
- Page 2679 and 2680:
• SE4-P007 CORROSION RESISTANT PE
- Page 2681 and 2682:
• SE4-P008 COATING OF NATURAL COR
- Page 2683 and 2684:
• SE4-P010 HIGH TEMPERATURE OXIDA
- Page 2685 and 2686:
• SE4-P012 ELECTROCHEMICAL DEPOSI
- Page 2687 and 2688:
• SE4-P014 ANALYSIS OF THE SUPERF
- Page 2689 and 2690:
Keywords: Corrosion, Concrete, Chlo
- Page 2691 and 2692:
• SE4-P017 GENERATION OF PITTING
- Page 2693 and 2694:
Acknowledgment: The authors wish to
- Page 2695 and 2696:
• SE4-P020 ELECTROCHEMICAL TECHNI
- Page 2697 and 2698:
• SE4-P022 PERFORMANCE OF SPRAY C
- Page 2699 and 2700:
• SE4-P024 HIERARCHICAL TaN / Ta
- Page 2701 and 2702:
Keywords: concrete, corrosion, rein
- Page 2703 and 2704:
• SE4-P027 CORROSION BEHAVIOR OF
- Page 2705 and 2706:
• SE4-P029 EVALUACIÓN ELECTROQU
- Page 2707 and 2708:
• SE4-P031 THERMAL FATIGUE EFFECT
- Page 2709 and 2710:
• SE4-P032 ASSESSMENT OF THE EMPL
- Page 2711 and 2712:
• SE4-P034 PARTITION ANALYSIS OF
- Page 2713 and 2714:
• SE4-P036 FAILURE ANALYSIS AND D
- Page 2715 and 2716:
• SE4-P037 EFFECT OF BORON, ZIRCO
- Page 2717 and 2718:
• SE4-P039 EXPERIMENTAL AND DFT S
- Page 2719 and 2720:
• SE4-P041 SEM IN THE STUDY OF FR
- Page 2721 and 2722:
• SE4-P043 EVALUATION OF A CATHOD
- Page 2723 and 2724:
ORAL SESSION
- Page 2725 and 2726:
sheet and tool steel alloys and the
- Page 2727 and 2728:
• SE5-O003 EFFECT OF COLD ROLLING
- Page 2729 and 2730:
• SE5-O004 HOT FORMING BEHAVIOUR
- Page 2731 and 2732:
hardenability changes in the inter-
- Page 2733 and 2734:
• SE5-O007 MICROSTRUCTURAL EVOLUT
- Page 2735 and 2736:
• SE5-O008 TRENDS IN STEEL USAGE
- Page 2737 and 2738:
include potential changes that will
- Page 2739 and 2740:
• SE5-O011 THERMAL TREATMENTS IN
- Page 2741 and 2742:
• SE5-O013 HIGH TEMPERATURE OXIDA
- Page 2743 and 2744:
• SE5-O014 Invited Talk THE ROLE
- Page 2745 and 2746:
• SE5-O016 RELATIONSHIP BETWEEN T
- Page 2747 and 2748:
Víctor García’s studies were sp
- Page 2749 and 2750:
Keywords: Metallurgy, welding, heat
- Page 2751 and 2752:
• SE5-O020 MICROSTRUCTURE AND MEC
- Page 2753 and 2754:
• SE5-O022 THEORETICAL AND PRACTI
- Page 2755 and 2756:
• SE5-O023 INFLUENCE OF THE PHASE
- Page 2757 and 2758:
POSTER SESSION
- Page 2759 and 2760:
Acknowledgment: Authors would like
- Page 2761 and 2762:
• SE5-P003 HEAT INPUT EFFECT ON T
- Page 2763 and 2764:
• SE5-P004 MICROSTRUCTURAL CHARAC
- Page 2765 and 2766:
Science and Technology (Consejo Nac
- Page 2767 and 2768:
Keywords: Carburizing, Distortion,
- Page 2769 and 2770:
Keywords: simulation, injection pro
- Page 2771 and 2772:
• SE5-P009 EFFECT OF THE ANNEALIN
- Page 2773 and 2774:
• SE5-P011 CORROSION OF STEEL TEN
- Page 2775 and 2776:
• SE5-P013 STUDY OF THE PERFORMAN
- Page 2777 and 2778:
• SE5-P015 ELECTRON BEAM SURFACE
- Page 2779 and 2780:
• SE5-P017 INVESTIGATION OF THERM
- Page 2781 and 2782:
Symposium F1 NEW ADVANCES IN SUPERC
- Page 2783 and 2784:
• SF1-O001 Invited Talk COMPARATI
- Page 2785 and 2786:
Acknowledgements: Authors would lik
- Page 2787 and 2788:
• SF1-O004 Invited Talk THE MARRI
- Page 2789 and 2790:
• SF1-O005 Invited Talk SIZE-INDU
- Page 2791 and 2792:
• SF1-O007 THE COULOMB BASED MAGN
- Page 2793 and 2794:
• SF1-O008 Invited Talk ROLE OF D
- Page 2795 and 2796:
Acknowledgment: MRA, APB and MA ack
- Page 2797 and 2798:
• SF1-O011 Invited Talk MAGNETIC
- Page 2799 and 2800:
[1] Li, G. and Yang, Y. Nature Phot
- Page 2801 and 2802:
Keywords: Exchange coupling, Nanost
- Page 2803 and 2804:
(2014). 10 J.P. Domann and G.P. Car
- Page 2805 and 2806:
obtained, changing cooling rate, im
- Page 2807 and 2808:
In this work, we present our endeav
- Page 2809 and 2810:
• SF1-O018 EXPLORATION OF NOISE I
- Page 2811 and 2812:
• SF1-P001 ANALYTICAL AND NUMERIC
- Page 2813 and 2814:
Acknowledgment: Research has been s
- Page 2815 and 2816:
Acknowledgment: I would like to tha
- Page 2817 and 2818:
• SF1-P005 STUDY OF PHYSICAL PROP
- Page 2819 and 2820:
• SF1-P007 PRODUCTION OF THE SUPE
- Page 2821 and 2822:
• SF1-P009 MAGNETIC AND RAMAN STU
- Page 2823 and 2824:
• SF1-P011 OPTIMAL DOPING FOR D-W
- Page 2825 and 2826:
• SF1-P013 STRUCTURAL, MAGNETIC,
- Page 2827 and 2828:
Symposium F2 ADVANCES IN FUNCTIONAL
- Page 2829 and 2830:
• SF2-O001 Invited Talk CRYSTAL G
- Page 2831 and 2832:
• SF2-O002 MONOMER DERIVED POLY(F
- Page 2833 and 2834:
• SF2-O004 EFFECT OF HEAT TREATME
- Page 2835 and 2836:
(3) Cherrington, R.; Hughes, D. J.;
- Page 2837 and 2838:
• SF2-O007 A SIMPLE METHOD TO OBT
- Page 2839 and 2840:
• SF2-O009 ZnO/PEDOT:PSS SCHOTTKY
- Page 2841 and 2842:
• SF2-O011 ELECTRICAL, MORPHOLOGI
- Page 2843 and 2844:
Acknowledgment: The authors gratefu
- Page 2845 and 2846:
• SF2-O014 PHASE SENSITIVE PREASU
- Page 2847 and 2848:
design of the PD, the distance betw
- Page 2849 and 2850:
Keywords: Diamond semiconductor, po
- Page 2851 and 2852:
• SF2-O018 FABRICATION AND CHARAC
- Page 2853 and 2854:
• SF2-O019 SELENIZATIONOF CIGSe S
- Page 2855 and 2856:
• SF2-O020 STRUCTURAL, ELECTRONIC
- Page 2857 and 2858:
• SF2-O021 SPRAY PYROLYSIS SYSTEM
- Page 2859 and 2860:
• SF2-O023 Invited Talk CARBON DO
- Page 2861 and 2862:
• SF2-O025 PHOTOCATALYTIC OXIDATI
- Page 2863 and 2864:
• SF2-O026 ARSENIC (V) REMOVAL US
- Page 2865 and 2866:
Keywords: Red mud, Functionalizatio
- Page 2867 and 2868:
• SF2-O029 SYNTHESIS AND PHOTOCAT
- Page 2869 and 2870:
• SF2-O030 Invited Talk STRUCTURA
- Page 2871 and 2872:
POSTER SESSION
- Page 2873 and 2874:
Keywords: TiO2-based transparent co
- Page 2875 and 2876:
• SF2-P003 HOPPING CONDUCTIVITY I
- Page 2877 and 2878:
• SF2-P004 LUMINISCENCE VARIATION
- Page 2879 and 2880:
• SF2-P006 FREQUENCY - ELECTRICAL
- Page 2881 and 2882:
• SF2-P007 FABRICATION OF THIN FI
- Page 2883 and 2884:
• SF2-P009 EFFECT OF POLYELECTROL
- Page 2885 and 2886:
dendrimers was confirmed using prot
- Page 2887 and 2888:
• SF2-P012 EFFECT OF THE REACTANT
- Page 2889 and 2890:
• SF2-P014 CHEMICAL-RESISTIVE BEH
- Page 2891 and 2892:
egimes for synthesis of white light
- Page 2893 and 2894:
• SF2-P017 COMPARATIVE STUDY OF T
- Page 2895 and 2896:
• SF2-P018 EFFECT OF TIME AND BAL
- Page 2897 and 2898:
• SF2-P020 TEORETICAL STUDY OF BA
- Page 2899 and 2900:
Keywords: Hybrid Perovskites, X-Ray
- Page 2901 and 2902:
• SF2-P023 ELECTRICAL, OPTICAL, A
- Page 2903 and 2904:
for InP@ZnS QDs.; also, we observed
- Page 2905 and 2906:
• SF2-P026 CHEMICAL SYNTHESIS AND
- Page 2907 and 2908:
• SF2-P028 CR REMOVAL FROM DRINKI
- Page 2909 and 2910:
• SF2-P030 SYNTHESIS AND CHARACTE
- Page 2911 and 2912:
• SF2-P032 INFLUENCE OF MEAN KINE
- Page 2913 and 2914:
• SF2-P033 EFFECT OF THE TEMPERAT
- Page 2915 and 2916:
• SF2-P035 STUDY OF THE EFFECT OF
- Page 2917 and 2918:
Supercomputing Center DGTIC-UNAM. J
- Page 2919 and 2920:
• SF2-P038 FIRST PRINCIPLES STUDY
- Page 2921 and 2922:
• SF2-P039 NANOWIRE/NANOLAYER HET
- Page 2923 and 2924:
• SF2-P041 ELECTROCHROMISM OF TiO
- Page 2925 and 2926:
• SF2-P042 INFLUENCE OF BORE DOPI
- Page 2927 and 2928:
• SF2-P044 EFFECT OF EUROPIUM ON
- Page 2929 and 2930:
measurements, M. Galvan and M. Aven
- Page 2931 and 2932:
• SF2-P047 SYNTHESIS OF SILVER NA
- Page 2933 and 2934:
• SF2-P049 INFLUENCE OF GRAPHENE
- Page 2935 and 2936:
• SF2-P051 OPTO-ELECTRONICS PROPE
- Page 2937 and 2938:
• SF2-P053 ELECTROCHEMICAL, MICRO
- Page 2939 and 2940:
• SF2-P054 PALLADIUM ASSISTED HOM
- Page 2941 and 2942:
• SF2-P056 AlGaN-BASED METAL-SEMI
- Page 2943 and 2944:
• SF2-P058 BI-TE BASED TOPOLOGICA
- Page 2945 and 2946:
• SF2-P060 STRUCTURAL TRNASFORMAT
- Page 2947 and 2948:
• SF2-P061 DENSITY FUNCTIONAL STU
- Page 2949 and 2950:
• SF2-P063 SYNTHESIS AND CHARACTE
- Page 2951 and 2952:
• SF2-P065 SURFACE MORPHOLOGIES O
- Page 2953 and 2954:
• SF2-P067 NUMERICAL MODELLING FO
- Page 2955 and 2956:
• SF2-P068 PREPARATION, CHARACTER
- Page 2957 and 2958:
• SF2-P070 PHYSICAL PROPERTIES OF
- Page 2959 and 2960:
• SF2-P072 SYNTHESIS, CHARACTERIZ
- Page 2961 and 2962:
[2] Ze-Xing Cai, Hua-Yao Li, Xiao-N
- Page 2963 and 2964:
Symposium F3 CATALYSIS BY MATERIALS
- Page 2965 and 2966:
• SF3-O001 Invited Talk ANALYSIS
- Page 2967 and 2968:
• SF3-O002 CHARACTERIZATION AND E
- Page 2969 and 2970:
• SF3-O003 TRIMETALLIC NIMOW SULF
- Page 2971 and 2972:
Ni/HMOR catalysts. The reaction was
- Page 2973 and 2974:
Polytechnic University of Valencia,
- Page 2975 and 2976:
• SF3-O007 NICKEL- IRON NANOPARTI
- Page 2977 and 2978:
• SF3-O008 STUDY OF THE EFFECT OF
- Page 2979 and 2980:
Acknowledgment: The authors acknowl
- Page 2981 and 2982:
Raman and XRD and their band gap en
- Page 2983 and 2984:
• SF3-O012 HYDROGEN PRODUCTION FR
- Page 2985 and 2986:
Keywords: Hydrodeoxygenation, Phosp
- Page 2987 and 2988:
• SF3-O015 INFLUENCE OF STRUCTURI
- Page 2989 and 2990:
• SF3-O016 Invited Talk CATALYTIC
- Page 2991 and 2992:
authors appreciate the support of A
- Page 2993 and 2994:
showed high selectivity to decalins
- Page 2995 and 2996:
• SF3-O020 Invited Talk MOLYBDENU
- Page 2997 and 2998:
• SF3-O022 Invited Talk EFFECT OF
- Page 2999 and 3000:
• SF3-O024 SOLAR-IRRADIATION DRIV
- Page 3001 and 3002:
• SF3-O025 DOPED TiO2 AS PHOTOCAT
- Page 3003 and 3004:
• SF3-O027 OPTIMIZATION OF THE CA
- Page 3005 and 3006:
• SF3-O028 PHOTO-DEGRADATION OF A
- Page 3007 and 3008:
• SF3-O029 Invited Talk MECHANIST
- Page 3009 and 3010:
of toluene was carried out at atmos
- Page 3011 and 3012:
the bands, in both synthesis method
- Page 3013 and 3014:
• SF3-O033 IRON-DOPED GRAPHITIC C
- Page 3015 and 3016:
• SF3-O035 TEMPLATE-FREE SELF-ASS
- Page 3017 and 3018:
8593. [4] G. Corro, C. Cano, J.L.G.
- Page 3019 and 3020:
• SF3-O038 DRY REFORMING OF METHA
- Page 3021 and 3022:
• SF3-O040 BIMETALLIC COPPER-BASE
- Page 3023 and 3024:
• SF3-O041 SYNTHESIS AND CHARACTE
- Page 3025 and 3026:
• SF3-O042 GOLD SELECTIVE DEPOSIT
- Page 3027 and 3028:
• SF3-O044 Invited Talk SYNTHESIS
- Page 3029 and 3030:
• SF3-O046 TREATMENT OF PHENOL BY
- Page 3031 and 3032:
• SF3-O048 CERIUM AND ZIRCONIUM O
- Page 3033 and 3034:
• SF3-O049 Invited Talk EFFECT OF
- Page 3035 and 3036:
• SF3-O050 THEORETICAL STUDY ON T
- Page 3037 and 3038:
The functionalized composites were
- Page 3039 and 3040:
Keywords: Schiff bases, Asymmetric
- Page 3041 and 3042:
• SF3-O054 BIODIESEL PRODUCTION F
- Page 3043 and 3044:
• SF3-O055 Invited Talk AROMATIZA
- Page 3045 and 3046:
R. M. (. Hassan Atashin Ph.D., «Ma
- Page 3047 and 3048:
1. H. Kabashima and H. Hattori, Cat
- Page 3049 and 3050:
• SF3-O059 Invited Talk EX-SITU A
- Page 3051 and 3052:
• SF3-O061 Invited Talk CATALYTIC
- Page 3053 and 3054:
atio of 3 and 2.5 more than the TiO
- Page 3055 and 3056:
POSTER SESSION
- Page 3057 and 3058:
The results of the simulation in th
- Page 3059 and 3060:
• SF3-P003 PHOTODEGRADATION OF 2,
- Page 3061 and 3062:
• SF3-P004 CORRELATION OF RH PART
- Page 3063 and 3064:
• SF3-P005 CATALYSTS DEVELOPMENT
- Page 3065 and 3066:
superoxide species on Ag 0 . The hi
- Page 3067 and 3068:
• SF3-P008 AN IMPROVED ADSORPTION
- Page 3069 and 3070:
• SF3-P009 SYNTHESIS AND CHARACTE
- Page 3071 and 3072:
• SF3-P011 KINETIC MODELING IN TH
- Page 3073 and 3074:
• SF3-P013 SYNTHESIS AND CHARACTE
- Page 3075 and 3076:
• SF3-P015 NAPHTHALENE HIDROGENAT
- Page 3077 and 3078:
Acknowledgment: Funding for this pr
- Page 3079 and 3080:
• SF3-P018 SYNTHESIS AND CHARACTE
- Page 3081 and 3082:
Scheme 1. 2-amino-4H-pyrans synthes
- Page 3083 and 3084:
XPS studies reveal that a TiO 2 amo
- Page 3085 and 3086:
Acknowledgment: Diego A. García-Ra
- Page 3087 and 3088:
• SF3-P023 NAPHTALENE HYDROGENATI
- Page 3089 and 3090:
and 4,6-dimethyldibenzothiophene. S
- Page 3091 and 3092:
• SF3-P026 VARIATION OF NIFE NANO
- Page 3093 and 3094:
Keywords: Oximes, SiO2 nanoparticle
- Page 3095 and 3096:
Materials From Nanoscience to Catal
- Page 3097 and 3098:
• SF3-P030 ADSORPTION AND DISSOCI
- Page 3099 and 3100:
• SF3-P031 TRANSITIONS STATES USI
- Page 3101 and 3102:
• SF3-P033 CARBON FORMATION OVER
- Page 3103 and 3104:
Hydrogen is vigorously pursued as a
- Page 3105 and 3106:
• SF3-P036 EVALUATION OF THE CATA
- Page 3107 and 3108:
• SF3-P037 SYNTHESIS AND CHARACTE
- Page 3109 and 3110:
• SF3-P039 EFFECTS OF FAU-TYPE ZE
- Page 3111 and 3112:
• SF3-P040 ENHANCED PHOTOCATALYTI
- Page 3113 and 3114:
• SF3-P041 CATALYTIC PROPERTIES O
- Page 3115 and 3116:
• SF3-P043 STUDY OF THE PHOTOCATA
- Page 3117 and 3118:
• SF3-P044 ELECTROCHEMICAL PERFOR
- Page 3119 and 3120:
• SF3-P046 SYNTHESIS AND CHARACTE
- Page 3121 and 3122:
• SF3-P048 SYNTHESIS AND CHARACTE
- Page 3123 and 3124:
• SF3-P050 NANOPARTICLES OF IRON
- Page 3125 and 3126:
• SF3-P051 Al2O3-TiO2-ZrO2 MIXED
- Page 3127 and 3128:
• SF3-P052 SYNTHESIS AND CHARACTE
- Page 3129 and 3130:
• SF3-P054 NICKEL NANOPARTICLES C
- Page 3131 and 3132:
• SF3-P056 HYDROGEN PRODUCTION BY
- Page 3133 and 3134:
• SF3-P058 PRODUCTION AND IMMOBIL
- Page 3135 and 3136:
• SF3-P060 DEVELOPMENT OF TRIMETA
- Page 3137 and 3138:
• SF3-P061 Ni CATALYSTS SUPPORTED
- Page 3139 and 3140:
• SF3-P063 CARBON DIOXIDE METHANA
- Page 3141 and 3142:
• SF3-P065 SYNTHESIS OF Pt-K-Ce /
- Page 3143 and 3144:
• SF3-P067 SYNTHESIS AND CHARACTE
- Page 3145 and 3146:
the adsorption of MB on the P25 cat
- Page 3147 and 3148:
to promote tandem reactions, requir
- Page 3149 and 3150:
ORAL SESSION
- Page 3151 and 3152:
• SF4-O002 FROM ELECTRONIC STABIL
- Page 3153 and 3154:
• SF4-O004 Invited Talk PROBING T
- Page 3155 and 3156:
• SF4-O005 SHINING LIGHT ON BORON
- Page 3157 and 3158:
• SF4-O007 Invited Talk Au/H: THE
- Page 3159 and 3160:
• SF4-O008 Invited Talk BORON IN
- Page 3161 and 3162:
• SF4-O010 DEVELOPMENT OF ANTICOR
- Page 3163 and 3164:
• SF4-O011 INTERFACIAL PROPERTIES
- Page 3165 and 3166:
• SF4-O013 Invited Talk C-H AND C
- Page 3167 and 3168:
V.A. Azov, K.R. Asmis, S. Grabowsky
- Page 3169 and 3170:
• SF4-O016 INSIGHTS ON THE PHASE
- Page 3171 and 3172:
Acknowledgment: The work in Mexico
- Page 3173 and 3174:
• SF4-P001 INDUCED MAGNETIC FIELD
- Page 3175 and 3176:
• SF4-P003 INFLUENCE OF THE INCUB
- Page 3177 and 3178:
ORAL SESSION
- Page 3179 and 3180:
• SF5-O002 SYNTHESIS AND EVALUATI
- Page 3181 and 3182:
• SF5-O004 APPLICATION OF LAYERED
- Page 3183 and 3184:
Acknowledgment: The authors would l
- Page 3185 and 3186:
Acknowledgment: The authors thank:
- Page 3187 and 3188:
• SF5-O008 Invited Talk THE USE O
- Page 3189 and 3190:
• SF5-O010 STIMULATION BY ELECTRI
- Page 3191 and 3192:
• SF5-O012 NATURAL CORROSION INHI
- Page 3193 and 3194:
Depollution, Springer, London 2013
- Page 3195 and 3196:
Keywords: Biogenic silica/titania c
- Page 3197 and 3198:
• SF5-O016 NITRIFICATION IN A MEM
- Page 3199 and 3200:
• SF5-O017 SUSTAINABLE BIO-BASED
- Page 3201 and 3202:
• SF5-O019 Invited Talk SYNTHESIS
- Page 3203 and 3204:
• SF5-O021 PREPARATION OF CERAMIC
- Page 3205 and 3206:
• SF5-O022 TRACE LEVEL PESTICIDE
- Page 3207 and 3208:
• SF5-O024 DEGRADATION OF ORGANOP
- Page 3209 and 3210:
• SF5-O026 Invited Talk ON THE RO
- Page 3211 and 3212:
• SF5-O028 POLYMERIC PHOTOSENSITI
- Page 3213 and 3214:
• SF5-O030 ZIRCONIUM ORGANOPHOSPH
- Page 3215 and 3216:
• SF5-O031 Invited Talk IMPROVEME
- Page 3217 and 3218:
• SF5-O033 A METHOD TO REDUCE WAS
- Page 3219 and 3220:
• SF5-O035 THE FATIGUE RESISTANCE
- Page 3221 and 3222:
Keywords: Mineralogical characteriz
- Page 3223 and 3224:
potentially infinite variety of nan
- Page 3225 and 3226:
• SF5-O039 DEVELOPMENT OF A NON-I
- Page 3227 and 3228:
• SF5-O041 Mg/Al LAYERED DOUBLE H
- Page 3229 and 3230:
• SF5-O042 GRAPHENE OXIDE FUNCTIO
- Page 3231 and 3232:
• SF5-O044 Invited Talk VISIBLE L
- Page 3233 and 3234:
• SF5-O046 STRUCTURAL CHARACTERIZ
- Page 3235 and 3236:
• SF5-O048 CARBON EMISSIONS AND B
- Page 3237 and 3238:
Keywords: byproducts, metals, remov
- Page 3239 and 3240:
as subproduct of metallurgic indust
- Page 3241 and 3242:
• SF5-O052 INERTIZATION OF PYRITI
- Page 3243 and 3244:
• SF5-O054 STUDY OF GETTING PAPER
- Page 3245 and 3246:
• SF5-P001 ENHANCE OF BIOGAS PROD
- Page 3247 and 3248:
• SF5-P003 SYNTHESIS AND CHARACTE
- Page 3249 and 3250:
The authors are grateful to CONACYT
- Page 3251 and 3252:
• SF5-P006 OBTENTION OF BIOETHANO
- Page 3253 and 3254:
• SF5-P008 DEVELOPMENT AND CHARAC
- Page 3255 and 3256:
• SF5-P010 BIODEGRADABILITY STUDY
- Page 3257 and 3258:
diseases so it was deduced that the
- Page 3259 and 3260:
composites. Institute of Materials
- Page 3261 and 3262:
Keywords: BiFeO3, rare-earth doping
- Page 3263 and 3264:
• SF5-P015 EVALUATION OF TITANIUM
- Page 3265 and 3266:
• SF5-P017 PHOTOCATALYTIC ACTIVIT
- Page 3267 and 3268:
• SF5-P018 SYNTHESIS OF ZNAL HYDR
- Page 3269 and 3270:
[4] J. Samdani, K. Samdani, N. H. K
- Page 3271 and 3272:
• SF5-P021 SYNTHESIS OF LaFeO3 VI
- Page 3273 and 3274:
• SF5-P022 SYNTHESIS OF MONOLITHS
- Page 3275 and 3276:
• SF5-P023 TB-SUBSTITUTED HYDROXY
- Page 3277 and 3278:
• SF5-P025 PREPARATION AND CHARAC
- Page 3279 and 3280:
• SF5-P026 DETERMINATION OF THE T
- Page 3281 and 3282:
Acknowledgment: Alejandra Michelle
- Page 3283 and 3284:
water samples both before and after
- Page 3285 and 3286:
• SF5-P030 SYNTHESIS AND CHARACTE
- Page 3287 and 3288:
• SF5-P032 MODELING OF ACTIVATED
- Page 3289 and 3290:
• SF5-P034 ADSORPTION OF TEXTILE
- Page 3291 and 3292:
• SF5-P036 MAGNETIC BIODEGRADABLE
- Page 3293 and 3294:
• SF5-P037 MAGNETIC BIOCOMPOSITE
- Page 3295 and 3296:
• SF5-P038 POLYELECTROLYTES CONTA
- Page 3297 and 3298:
• SF5-P040 COLORIMETRIC DETECTION
- Page 3299 and 3300:
• SF5-P042 SYNTHESIS AND CHARACTE
- Page 3301 and 3302:
• SF5-P043 FLOTATION OF A COMPLEX
- Page 3303 and 3304:
• SF5-P045 MEASUREMENTS OF THE TH
- Page 3305 and 3306:
• SF5-P047 MECHANICAL AND THERMAL
- Page 3307 and 3308:
• SF5-P049 TERA HERTZ EMISSION SI
- Page 3309 and 3310:
• SF5-P050 CARACTERIZACIÓN Y EVA
- Page 3311 and 3312:
• SF5-P052 POLIMERYC OPTIC FIBER
- Page 3313 and 3314:
• SF5-P053 EFFECT OF THE FORMULAT
- Page 3315 and 3316:
• SF5-P055 COMPLEMENTARY EXPERIME
- Page 3317 and 3318:
(3-aminopropyl) triethoxysilane. Gr
- Page 3319 and 3320:
• SF5-P058 DEVELOPMENT OF MIXED O
- Page 3321 and 3322:
• SF5-P059 MECHANICAL PROPERTIES
- Page 3323 and 3324:
• SF5-P061 POLYURETHANE FOAM-PHEN
- Page 3325 and 3326:
• SF5-P062 WATER AND CHLORIDE PER
- Page 3327 and 3328:
• SF5-P064 ELABORATION OF BRICKS
- Page 3329 and 3330:
• SF5-P066 DESIGN OF ASPHALT MIXT
- Page 3331 and 3332:
• SF5-P067 MECHANICAL PROPERTIES
- Page 3333 and 3334:
• SF5-P068 EFFECT OF THE ADDITION
- Page 3335 and 3336:
• SF5-P070 HYBRID CEMENTS BASED O
- Page 3337 and 3338:
• SF5-P072 TRACE LEVEL PESTICIDE
- Page 3339 and 3340:
• SF5-P074 SYNTHESIS AND CHARACTE
- Page 3341 and 3342:
Keywords: CO2 electrochemical reduc
- Page 3343 and 3344:
• SF5-P077 EFFECT OF THE pH ON PR
- Page 3345 and 3346:
10 mL aliquots of a 10 mg/L NaF sol
- Page 3347 and 3348:
Acknowledgment: To CONACYT, for the
- Page 3349 and 3350:
• SF5-P081 METHYLENE BLUE DEGRADA
- Page 3351 and 3352:
differentiate the specific mechanis
- Page 3353 and 3354:
Martínez Molina, W., Navarro Sánc
- Page 3355 and 3356:
• SF5-P085 POROSITY DEVELOPMENT O
- Page 3357 and 3358:
• SF5-P086 SUGARCANE AGROINDUSTRI
- Page 3359 and 3360:
• SF5-P088 BIOETHANOL PRODUCTION
- Page 3361 and 3362:
• SF5-P089 CHARACTERIZATION OF BI
- Page 3363 and 3364:
• SF5-P091 PHYSICS PROPERTIES OF
- Page 3365 and 3366:
• SF5-P093 EVALUATION OF THE BIOC
- Page 3367 and 3368:
Acknowledgment: AGRADESCO A LA UNIV
- Page 3369 and 3370:
• SF5-P096 PHOTOSENSITIZING EVALU
- Page 3371 and 3372:
Keywords: Ag nanoparticles, TiO2, P
- Page 3373 and 3374:
• SF5-P099 FABRICATION OF ZnO AT
- Page 3375 and 3376:
• SF5-P101 OBTAINING HYDROXYHYDRO
- Page 3377 and 3378:
• SF5-P103 STRUCTURAL CHARACTERIZ
- Page 3379 and 3380:
• SF5-P105 SYNTHESIS OF NANOCOMPO
- Page 3381 and 3382:
• SF5-P106 EFFICIENT DEGRADATION
- Page 3383 and 3384:
• SF5-P107 CO2 PHOTOREDUCTION WIT
- Page 3385 and 3386:
• SF5-P108 MICROWAVE-ASSISTED HYD
- Page 3387 and 3388:
• SF5-P110 ELABORATION AND CHARAC
- Page 3389 and 3390:
Symposium F6 STRATEGIES FOR ACADEMY
- Page 3391 and 3392:
• SF6-O001 Invited Talk ESTRATEGI
- Page 3393 and 3394:
• SF6-O002 TRAINING OF LEADERS IN
- Page 3395 and 3396:
• SF6-O003 VISUAL ANALYSIS OF CER
- Page 3397 and 3398:
• SF6-O005 VINCULACIÓN AGRICULTU
- Page 3399 and 3400:
adaptar y dimensionar los component
- Page 3401 and 3402:
• SF6-O008 Invited Talk INTEGRACI
- Page 3403 and 3404:
• SF6-O010 THE IDEAL ACADEMIC ENV
- Page 3405 and 3406:
• SF6-O011 POSGRADOS PROFESIONALI
- Page 3407 and 3408:
• SF6-O012 APLICACIONES TECNOLOGI
- Page 3409 and 3410:
• SF6-O014 CENTURY 21TH UNIVERSIT
- Page 3411 and 3412:
• SF6-O016 TRANSLATIONAL RESEARCH
- Page 3413 and 3414:
Acknowledgment: Mónica Casalet es
- Page 3415 and 3416:
• SF6-O019 PLASMA STIRLING CRYOGE
- Page 3417 and 3418:
• SF6-O021 Invited Talk EL CENTRO
- Page 3419 and 3420:
• SF6-P001 BENEFICIOS DE LA CIENC
- Page 3421 and 3422:
• SF6-P002 STRATEGIES TO PROMOTE
- Page 3423 and 3424:
Acknowledgment: The authors would l
- Page 3425 and 3426:
Keywords: Educational model, Social
- Page 3427 and 3428:
• SF6-P006 LA GESTIÓN DEL CONOCI
- Page 3429 and 3430:
• SF6-P007 EFECTO DE LA PRODUCTIV
- Page 3431 and 3432:
• SF6-P009 FABRICACIÓN DE UN DIS
- Page 3433 and 3434:
• SF6-P011 STUDY OF THE STABILITY
- Page 3435 and 3436:
• SF6-P012 DEVELOPMENT OF SOLAR C
- Page 3437 and 3438:
• SF6-P014 DESARROLLO DE UNA PLAN
- Page 3439 and 3440:
• SF6-P015 NANO PARTICULAS DE PLA
- Page 3441 and 3442:
• SF6-P017 OZONIZATION OF EMU OIL
- Page 3443 and 3444:
Symposium F7 PREPARING THE NEXT GEN
- Page 3445 and 3446:
• SF7-O002 Invited Talk PREPARING
- Page 3447 and 3448:
• SF7-O004 Invited Talk Pitt-NOCM
- Page 3449 and 3450:
• SF7-O006 ONGOING TRANSFORMATION
- Page 3451 and 3452:
• SF7-O008 IMPORTANT PHILOSOPHICA
- Page 3453 and 3454:
• SF7-O009 IMPORTANCE OF METACOGN
- Page 3455 and 3456:
• SF7-O011 Invited Talk SCIENTIST
- Page 3457 and 3458:
• SF7-O013 Invited Talk CASE STUD
- Page 3459 and 3460:
• SF7-O015 Invited Talk STRATEGIE
- Page 3461 and 3462:
Engineering and Diversity (CEED) co
- Page 3463 and 3464:
is at its mid point in its time fra
- Page 3465 and 3466:
• SF7-O019 Invited Talk SEVENTEEN
- Page 3467 and 3468:
• SF7-O021 THE BEAUTY OF SCIENCE
- Page 3469 and 3470:
• SF7-O022 Invited Talk USING RES
- Page 3471 and 3472:
• SF7-O024 Invited Talk MATERIALS
- Page 3473 and 3474:
• SF7-O026 Invited Talk FOSTERING
- Page 3475 and 3476:
• SF7-O027 THE SCIENTIFIC METHOD
- Page 3477 and 3478:
• SF7-P001 HIGH SCHOOL STUDENTS C
- Page 3479 and 3480:
• SF7-P003 SYNTHESIS OF RADIOABSO
- Page 3481 and 3482:
Motor skill improvement was associa
- Page 3483 and 3484:
• SF7-P006 SIO2/DA MATERIAL FOR D
- Page 3485 and 3486:
• SF7-P007 CARBURIZING, A NON-EQU
- Page 3487 and 3488:
• SF7-P009 CHARACTERIZATION OF TH
- Page 3489 and 3490:
• SF7-P011 EVALUATION OF THE SHAP
- Page 3491 and 3492:
Symposium WMC 4TH NANOMXCN: MEXICO-
- Page 3493 and 3494:
• SWMC-O001 PROGRESSES AND OPPORT
- Page 3495 and 3496:
• SWMC-O003 Invited Talk MULTIFUN
- Page 3497 and 3498:
Challenges and perspectives for Afr
- Page 3499 and 3500:
• SWMC-O006 Invited Talk EFFICIEN
- Page 3501 and 3502:
Energy, Office of Science, Office o
- Page 3503 and 3504:
• SWMC-O009 Invited Talk APPLICAT
- Page 3505 and 3506:
Acknowledgment: We acknowledge fina
- Page 3507 and 3508:
metals. The pathway to commercializ
- Page 3509 and 3510:
• SWMC-O013 RECENT DEVELOPMENTS A
- Page 3511 and 3512:
• SWMC-O015 THIRD GENERATION SOLA
- Page 3513 and 3514:
• SWMC-O016 NANOCOMPOSITES OF POL
- Page 3515 and 3516:
Acknowledgment: The work was suppor
- Page 3517 and 3518:
References 1 W. Zhao, et al., J. Am
- Page 3519 and 3520:
that may be viable for scale-up. Ex
- Page 3521 and 3522:
• SWMC-O021 Invited Talk UNDERSTA
- Page 3523 and 3524:
• SWMC-O023 Invited Talk THE ROLE
- Page 3525 and 3526:
• SWMC-O024 Invited Talk SPECIATI
- Page 3527 and 3528:
• SWMC-O026 THE USE OF WASTE ACTI
- Page 3529 and 3530:
tests were conducted under solar si
- Page 3531 and 3532:
• SWMC-O029 CONTROLLING THE FORMA
- Page 3533 and 3534:
Acknowledgment: CONACYT 251279 Keyw
- Page 3535 and 3536:
• SWMC-O032 Invited Talk EVALUATI
- Page 3537 and 3538:
• SWMC-O034 IS NANOSILVER BETTER
- Page 3539 and 3540:
• SWMC-O036 Invited Talk NANO-BIO
- Page 3541 and 3542:
• SWMC-P001 ADHESION MEASUREMENTS
- Page 3543 and 3544:
• SWMC-P003 STUDY OF THE INFLUENC
- Page 3545 and 3546:
• SWMC-P005 THE INFLUENCE OF MESO
- Page 3547 and 3548:
as precursor of the anchoring group
- Page 3549 and 3550:
• SWMC-P008 UNDERSTANDING THE ROL
- Page 3551 and 3552:
• SWMC-P010 THERMAL STABILITY OF
- Page 3553 and 3554:
• SWMC-P012 DEVELOPMENT OF COUNTE
- Page 3555 and 3556:
follow trend was observed TiO 2 ≈
- Page 3557 and 3558:
3. D.Y. Li, Y.G. Zhang, Y.L. Zhang,
- Page 3559 and 3560:
• SWMC-P016 TiO2, Al2O3 , ZrO2 AN
- Page 3561 and 3562:
ORAL SESSION
- Page 3563 and 3564:
• SWS-O002 Invited Talk LACCASES
- Page 3565 and 3566:
• SWS-O003 Invited Talk LEVERAGIN
- Page 3567 and 3568:
Acknowledgment: Funding USDA-NIFA,
- Page 3569 and 3570:
• SWS-O006 SEARCH FOR ANTARCTIC E
- Page 3571 and 3572:
• SWS-O007 INCREASING THE THRESHO
- Page 3573 and 3574:
• SWS-O008 DESIGN OF UNIDIRECTION
- Page 3575 and 3576:
were used, which contains the progr
- Page 3577 and 3578:
• SWS-O011 STUDY OF MICRO-CRACKIN
- Page 3579 and 3580:
• SWS-O013 PREDICTION OF TEMPERAT
- Page 3581 and 3582:
• SWS-O015 MICROSTRUCTURE PREDICT
- Page 3583 and 3584:
References: [1] Materials Research
- Page 3585 and 3586:
Acknowledgment: The authors acknowl
- Page 3587 and 3588:
• SWS-O019 LEAD-FREE PIEZOELECTRI
- Page 3589 and 3590:
• SWS-O021 ASSESSMENT OF THE THER
- Page 3591 and 3592:
• SWS-O023 Invited Talk RESEARCH
- Page 3593 and 3594:
• SWS-O025 Invited Talk RESEARCH
- Page 3595 and 3596:
AUTHOR INDEX A Acosta, Alonso Acost
- Page 3597 and 3598:
AUTHOR INDEX Allende Alarcón, Luis
- Page 3599 and 3600:
AUTHOR INDEX Arancibia Bulnes, Cami
- Page 3601 and 3602:
AUTHOR INDEX Ayala Villalobos, Scar
- Page 3603 and 3604:
AUTHOR INDEX Becerril Rodriguez, Da
- Page 3605 and 3606:
AUTHOR INDEX Bucio, E. Bucio, Emili
- Page 3607 and 3608:
AUTHOR INDEX Cano Castillo, Guadalu
- Page 3609 and 3610:
AUTHOR INDEX Castillo Santillan, Ma
- Page 3611 and 3612:
AUTHOR INDEX Chávez García, Hugo
- Page 3613 and 3614:
AUTHOR INDEX Cook, Cameron SD1-O046
- Page 3615 and 3616:
AUTHOR INDEX Damman, Pascal Dang, H
- Page 3617 and 3618:
AUTHOR INDEX Diaz Nava, Maria del C
- Page 3619 and 3620:
AUTHOR INDEX Endrino, Jose Luis SB6
- Page 3621 and 3622:
AUTHOR INDEX Fajardo San Miguel, Ge
- Page 3623 and 3624:
AUTHOR INDEX Francis, Leonard Franc
- Page 3625 and 3626:
AUTHOR INDEX García Hernández, Ma
- Page 3627 and 3628:
AUTHOR INDEX Gnade, Bruce Go, Yohan
- Page 3629 and 3630:
AUTHOR INDEX Gonzalez Varela, Danie
- Page 3631 and 3632:
AUTHOR INDEX Gutierrez Viezcas, Jos
- Page 3633 and 3634:
AUTHOR INDEX Hernandez Hernandez, A
- Page 3635 and 3636:
AUTHOR INDEX Herrera, Bernardo Herr
- Page 3637 and 3638:
AUTHOR INDEX Jackman, Richard Jacob
- Page 3639 and 3640:
AUTHOR INDEX Kabachkov, Evgeniy SA1
- Page 3641 and 3642:
AUTHOR INDEX Lapas, Luciano C. SC6-
- Page 3643 and 3644:
AUTHOR INDEX Liz Marzán, Luis M. L
- Page 3645 and 3646:
AUTHOR INDEX López, Mario Alberto
- Page 3647 and 3648:
AUTHOR INDEX Malpica Maldonado, Jon
- Page 3649 and 3650:
AUTHOR INDEX Martínez Peréz, Rosa
- Page 3651 and 3652:
AUTHOR INDEX Melo Banda, Jose Aaró
- Page 3653 and 3654:
AUTHOR INDEX Mitre Martínez, Doris
- Page 3655 and 3656:
AUTHOR INDEX Moreno, Juan Roberto S
- Page 3657 and 3658:
AUTHOR INDEX Neto, Antônio de Brit
- Page 3659 and 3660:
AUTHOR INDEX Olvera Amador, María
- Page 3661 and 3662:
AUTHOR INDEX Pacheco, José Sergio
- Page 3663 and 3664:
AUTHOR INDEX Pereyra, Andrea M. SB6
- Page 3665 and 3666:
AUTHOR INDEX Pineda Triana, Yaneth
- Page 3667 and 3668:
AUTHOR INDEX R R. Cortez, Youshiky
- Page 3669 and 3670:
AUTHOR INDEX Renteria Urquiza, Mait
- Page 3671 and 3672:
AUTHOR INDEX Robles Hernandez, Fran
- Page 3673 and 3674:
AUTHOR INDEX Rodriguez, Nancy Rodr
- Page 3675 and 3676:
AUTHOR INDEX Ruiz Vilchez, Julio Ad
- Page 3677 and 3678:
AUTHOR INDEX Sánchez González, No
- Page 3679 and 3680:
AUTHOR INDEX Sastre, Angela Sathish
- Page 3681 and 3682:
AUTHOR INDEX Solana Madruga, Elena
- Page 3683 and 3684:
AUTHOR INDEX Tamayo Pérez, Ulises
- Page 3685 and 3686:
AUTHOR INDEX Torres Torres, J. Gilb
- Page 3687 and 3688:
AUTHOR INDEX Valenzuela, Miguel Val
- Page 3689 and 3690:
AUTHOR INDEX Velázquez Osorio, Adr
- Page 3691 and 3692:
AUTHOR INDEX Wang, Lin SWMC-O022 Wu
- Page 3693 and 3694:
AUTHOR INDEX Zarazua, Isaac SC4-P05
Inappropriate
Loading...
Inappropriate
You have already flagged this document.
Thank you, for helping us keep this platform clean.
The editors will have a look at it as soon as possible.
Mail this publication
Loading...
Embed
Loading...
Delete template?
Are you sure you want to delete your template?
DOWNLOAD ePAPER
This ePaper is currently not available for download.
You can find similar magazines on this topic below under ‘Recommendations’.